Modelling Semantic Context of OOV Words in Large Vocabulary Continuous Speech Recognition.
IEEE/ACM Trans. Audio, Speech & Language Processing(2017)
摘要
The diachronic nature of broadcast news data leads to the problem of out-of-vocabulary OOV words in large vocabulary continuous speech recognition LVCSR systems. Analysis of OOV words reveals that a majority of them are proper names PNs. However, PNs are important for automatic indexing of audio-video content and for obtaining reliable automatic transcriptions. In this paper, we focus on the problem of OOV PNs in diachronic audio documents. To enable the recovery of the PNs missed by the LVCSR system, relevant OOV PNs are retrieved by exploiting the semantic context of the LVCSR transcriptions. For retrieval of OOV PNs, we explore topic and semantic context derived from latent Dirichlet allocation LDA topic models, continuous word vector representations and the neural bag-of-words NBOW model which is capable of learning task specific word and context representations. We propose a neural bag-of-weighted words NBOW2 model which learns to assign higher weights to words that are important for retrieval of an OOV PN. With experiments on French broadcast news videos, we show that the NBOW and NBOW2 models outperform the methods based on raw embeddings from LDA and Skip-gram models. Combining the NBOW and NBOW2 models gives a faster convergence during training. Second pass speech recognition experiments, in which the LVCSR vocabulary and language model are updated with the retrieved OOV PNs, demonstrate the effectiveness of the proposed context models.
更多查看译文
关键词
Context,Vocabulary,Context modeling,Speech recognition,Semantics,Training,Computational modeling
AI 理解论文
溯源树
样例
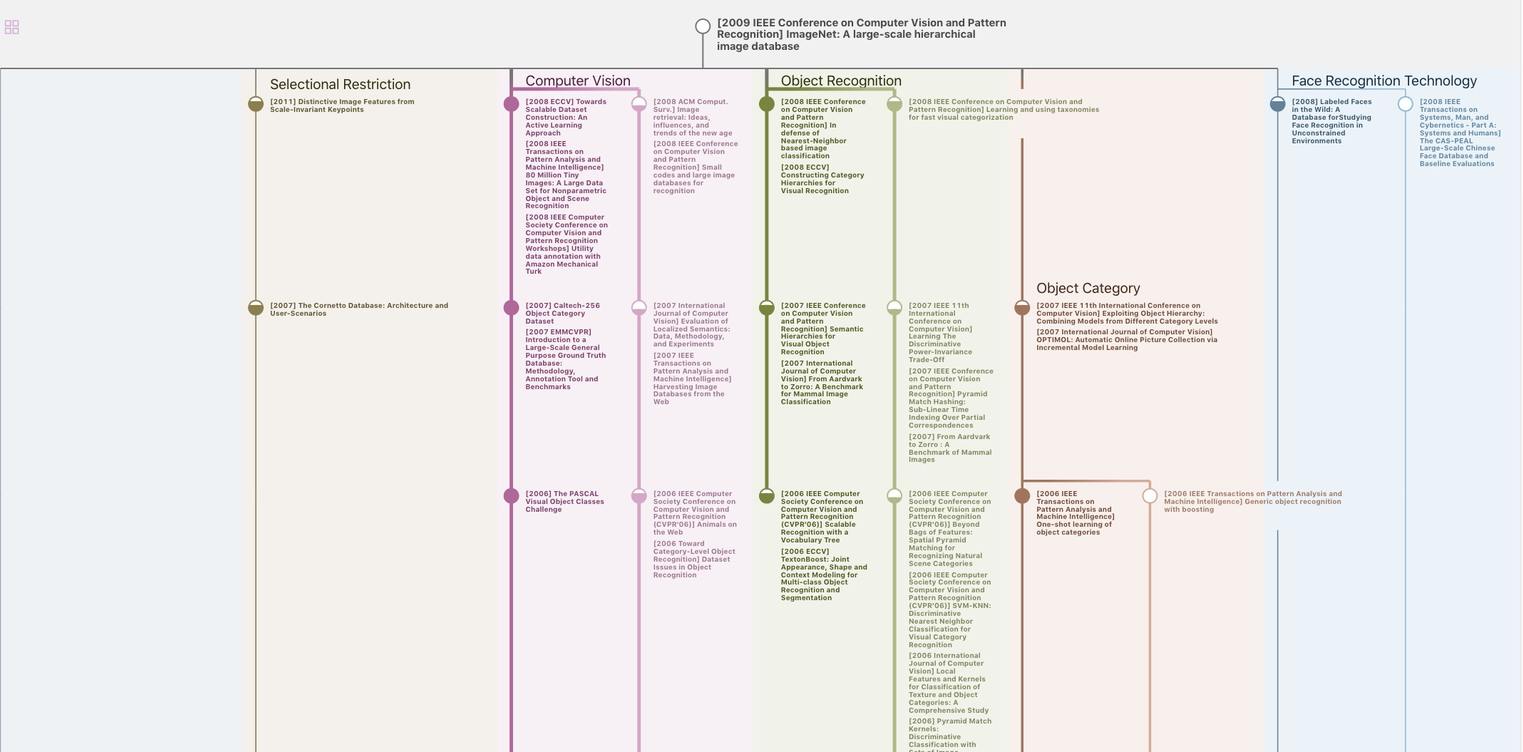
生成溯源树,研究论文发展脉络
Chat Paper
正在生成论文摘要