Modeling, analysis, and experimental comparison of streaming graph-partitioning policies.
Journal of Parallel and Distributed Computing(2017)
摘要
In recent years, many distributed graph-processing systems have been designed and developed to analyze large-scale graphs. For all distributed graph-processing systems, partitioning graphs is a key part of processing and an important aspect to achieve good processing performance. To keep low the overhead of partitioning graphs, even when processing the ever-increasing modern graphs, many previous studies use lightweight streaming graph-partitioning policies. Although many such policies exist, currently there is no comprehensive study of their impact on load balancing and communication overheads, and on the overall performance of graph-processing systems. This relative lack of understanding hampers the development and tuning of new streaming policies, and could limit the entire research community to the existing classes of policies. We address these issues in this work. We begin by modeling the execution time of distributed graph-processing systems. By analyzing this model under the load of realistic graph-data characteristics, we propose a method to identify important performance issues and then design new streaming graph-partitioning policies to address them. By using three typical large-scale graphs and three popular graph-processing algorithms, we conduct comprehensive experiments to study the performance of our and of many alternative streaming policies on a real distributed graph-processing system. We also explore the impact on performance of using different real-world networks and of other real-world technical details. We further discuss how to use our results, the coverage of our model and method, and the design of future partitioning policies.
更多查看译文
关键词
Streaming graph-partitioning policies,Large-scale graphs,Graph-processing systems,Modeling analysis,Performance evaluation
AI 理解论文
溯源树
样例
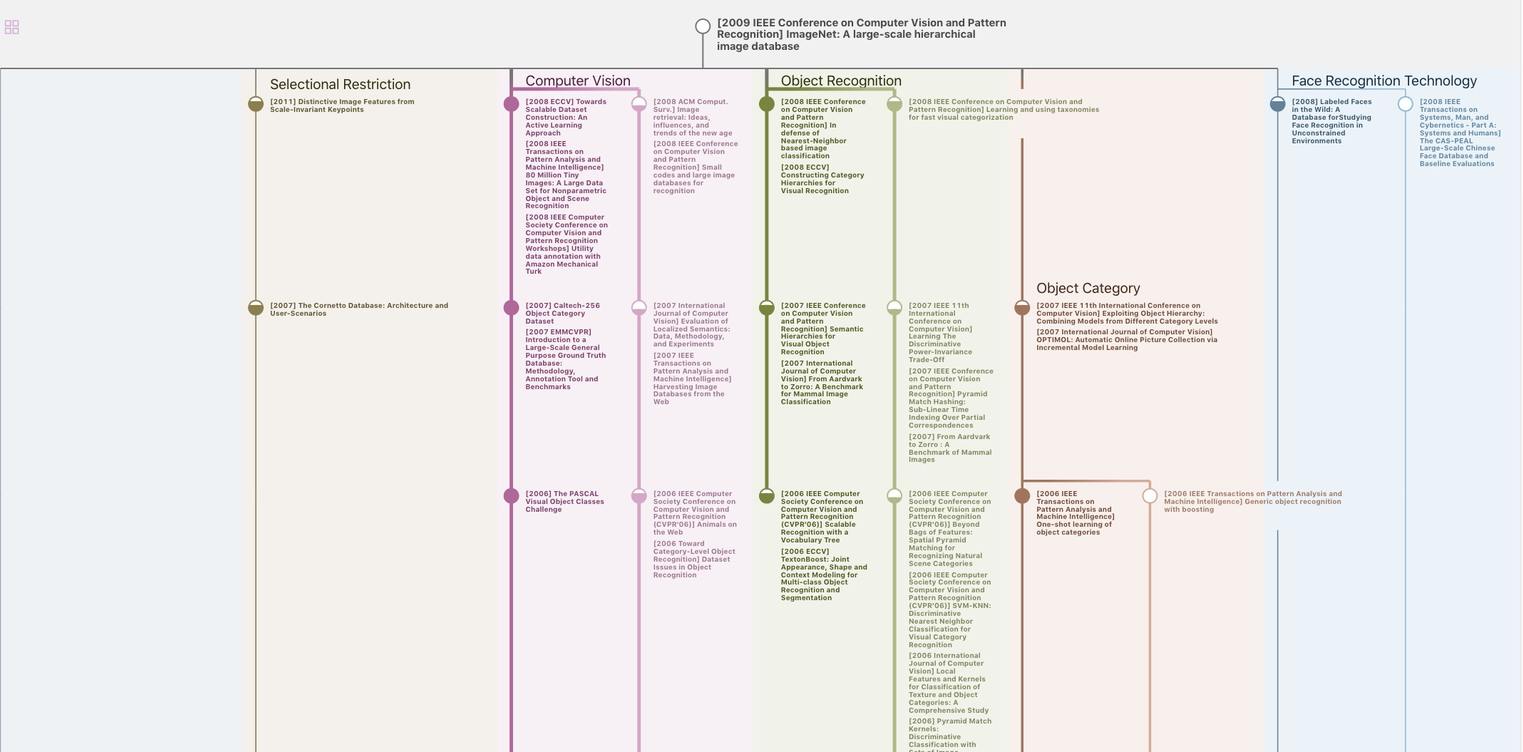
生成溯源树,研究论文发展脉络
Chat Paper
正在生成论文摘要