Data mining process for identification of non-spontaneous saccadic movements in clinical electrooculography.
Neurocomputing(2017)
摘要
In this paper we evaluate the use of the machine learning algorithms Support Vector Machines (SVM), K-Nearest Neighbors (KNN) and Classification and Regression Trees (CART) to identify non-spontaneous saccades in clinical electrooculography tests. We propose a modification to an adaptive threshold estimation algorithm for detecting signal impulses without the need for any manually pre-established parameters. Data mining tasks such as feature selection and model tuning were performed, obtaining very efficient models using only 3 attributes: amplitude deviation, absolute response latency and relative latency. The models were evaluated with signals recorded from subjects affected by Spinocerebellar Ataxia type 2 (SCA2). Results obtained by the algorithm show accuracies over 98%, recalls over 98% and precisions over 95% for the three models evaluated.
更多查看译文
关键词
Saccade identification,Clinical electrooculography,Support vector machines,K-nearest neighbors,Classification and regression trees
AI 理解论文
溯源树
样例
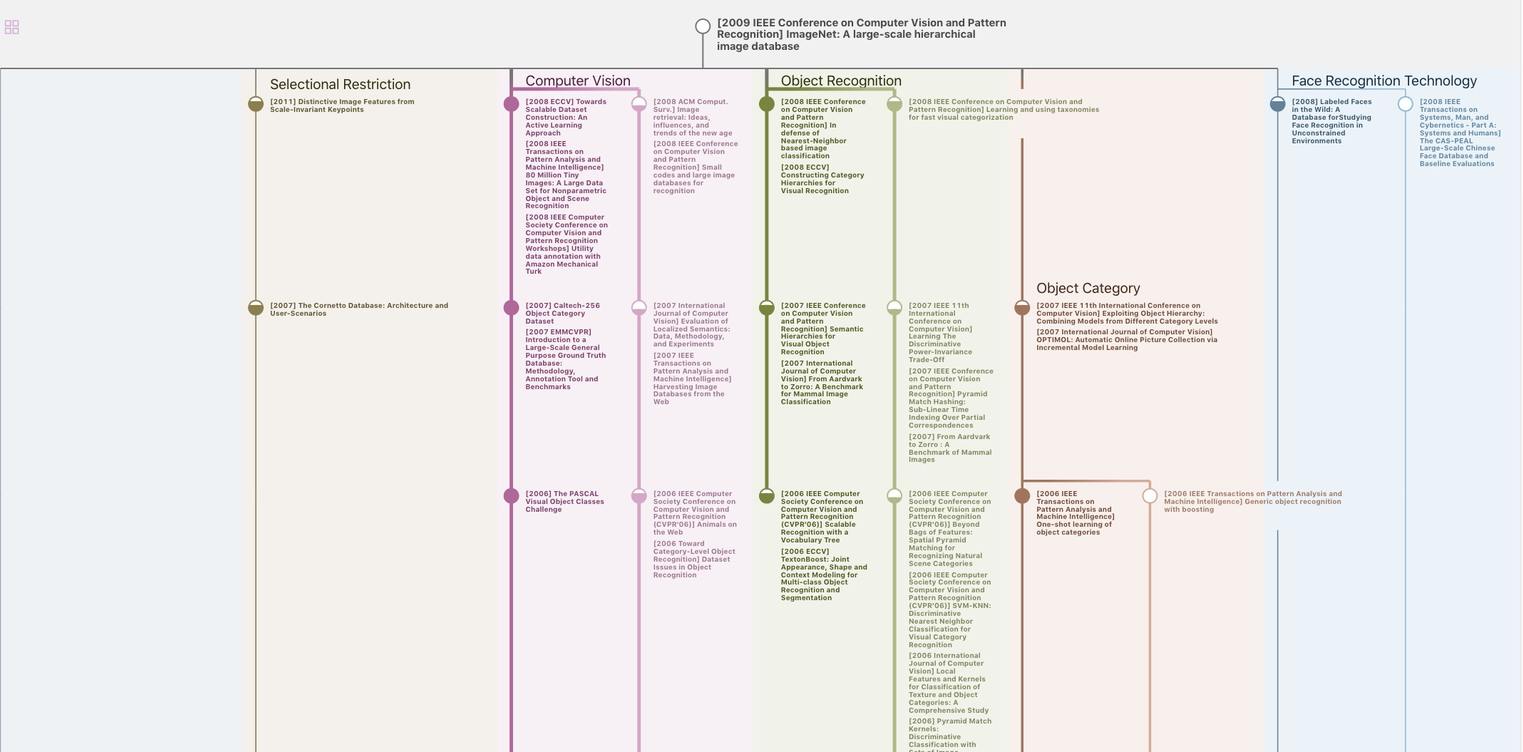
生成溯源树,研究论文发展脉络
Chat Paper
正在生成论文摘要