Exploiting the complementary strengths of multi-layer CNN features for image retrieval.
Neurocomputing(2017)
摘要
Deep convolutional neural networks have demonstrated breakthrough accuracies for image classification. A series of feature extractors learned from CNN have been used in other computer vision tasks. However, CNN features of different layers aim to encode different-level information. High-layer features care more about semantic information but less detail information, while low-layer features contain more detail information but suffer from the problem of background clutter and semantic ambiguity. We propose to exploit complementary strengths of different layers in a simple but effective way. A mapping function is designed to highlight the effectiveness of low-layer similarity, when measuring fine-grained similarity between query image and its nearest neighbors with similar semantic. Extensive experiments show that our method can achieve competitive performance on popular retrieval benchmarks. Extensive experiments show that the proposed method outperforms the features extracted from single layers and their direct concatenations. Meanwhile, our method achieves competitive performance on popular retrieval benchmarks.
更多查看译文
关键词
Image retrieval,CNN,Multi-level features
AI 理解论文
溯源树
样例
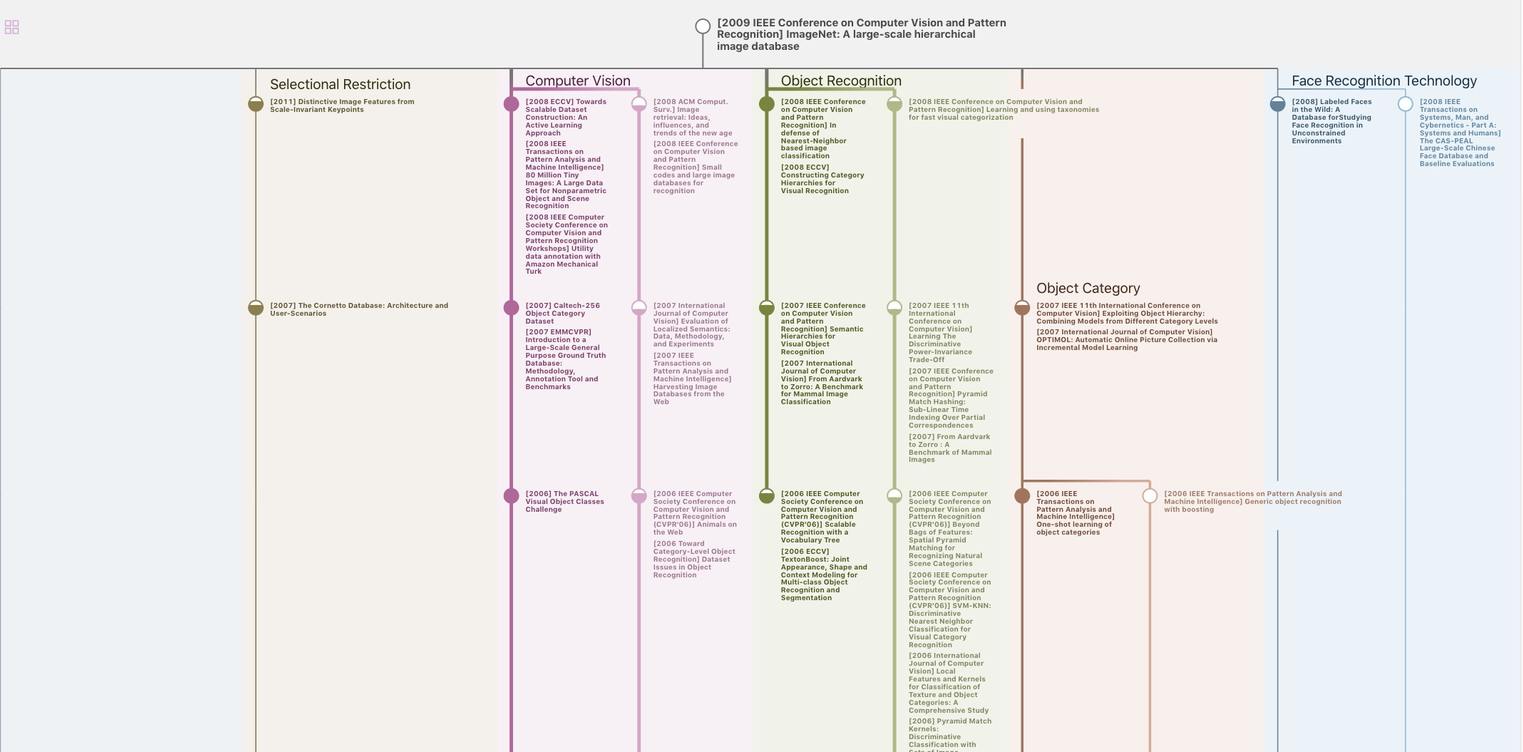
生成溯源树,研究论文发展脉络
Chat Paper
正在生成论文摘要