A hybrid feature selection algorithm for gene expression data classification.
Neurocomputing(2017)
摘要
In the DNA microarray research field, the increasing sample size and feature dimension of the gene expression data prompt the development of an efficient and robust feature selection algorithm for gene expression data classification. In this study, we propose a hybrid feature selection algorithm that combines the mutual information maximization (MIM) and the adaptive genetic algorithm (AGA). Experimental results show that the proposing MIMAGA-Selection method significantly reduces the dimension of gene expression data and removes the redundancies for classification. The reduced gene expression dataset provides highest classification accuracy compared to conventional feature selection algorithms. We also apply four different classifiers to the reduced dataset to demonstrate the robustness of the proposed MIMAGA-Selection algorithm.
更多查看译文
关键词
Feature selection,Mutual information maximization,Adaptive genetic algorithm,Gene expression data
AI 理解论文
溯源树
样例
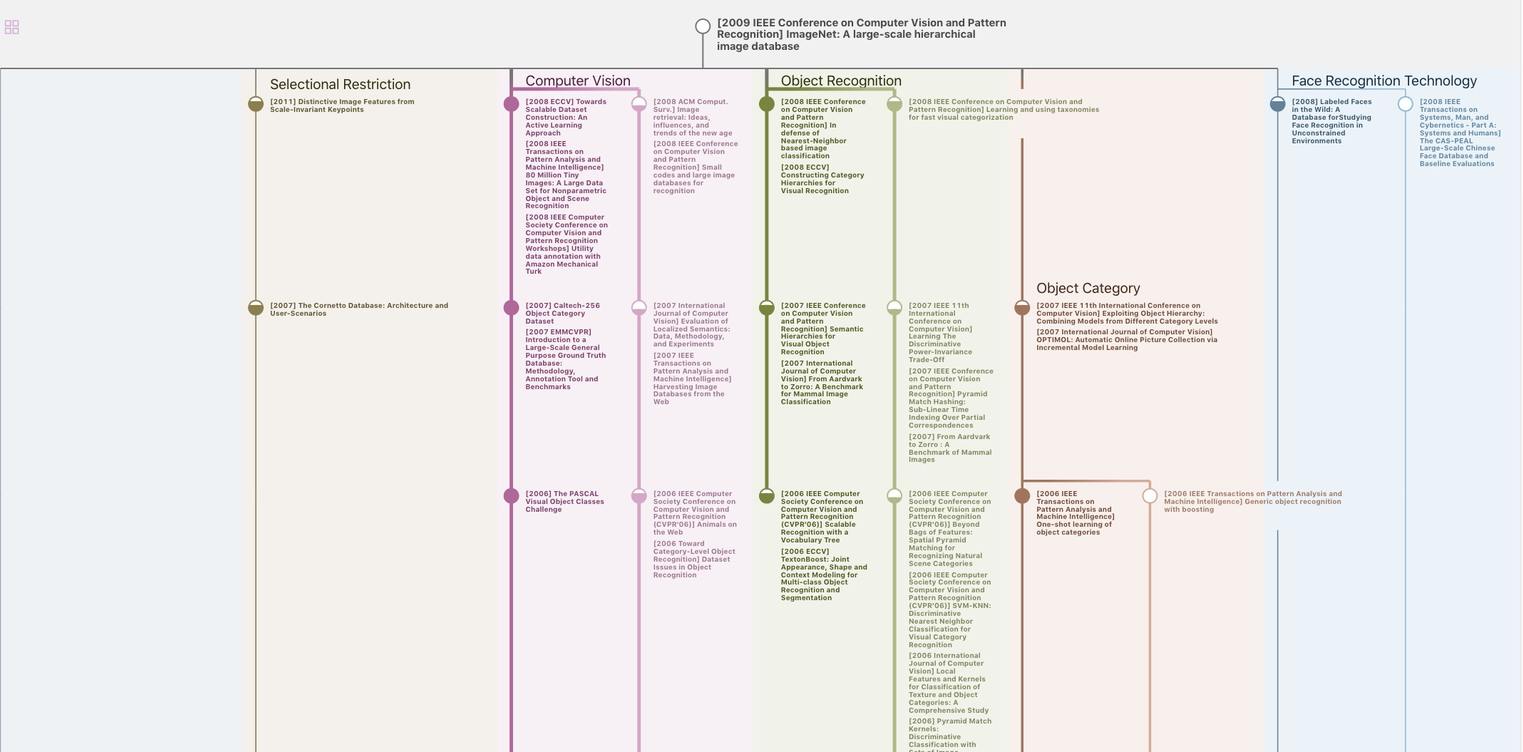
生成溯源树,研究论文发展脉络
Chat Paper
正在生成论文摘要