Empirical Mode Decomposition based ensemble deep learning for load demand time series forecasting.
Appl. Soft Comput.(2017)
摘要
Graphical abstractDisplay Omitted HighlightsAn ensemble deep learning method has been proposed for load demand forecasting.The hybrid method composes of Empirical Mode Decomposition and Deep Belief Network.Empirical Mode Decomposition based methods outperform the single structure models.Deep learning shows more advantages when the forecasting horizon increases. Load demand forecasting is a critical process in the planning of electric utilities. An ensemble method composed of Empirical Mode Decomposition (EMD) algorithm and deep learning approach is presented in this work. For this purpose, the load demand series were first decomposed into several intrinsic mode functions (IMFs). Then a Deep Belief Network (DBN) including two restricted Boltzmann machines (RBMs) was used to model each of the extracted IMFs, so that the tendencies of these IMFs can be accurately predicted. Finally, the prediction results of all IMFs can be combined by either unbiased or weighted summation to obtain an aggregated output for load demand. The electricity load demand data sets from Australian Energy Market Operator (AEMO) are used to test the effectiveness of the proposed EMD-based DBN approach. Simulation results demonstrated attractiveness of the proposed method compared with nine forecasting methods.
更多查看译文
关键词
Empirical Mode Decomposition,Deep learning,Ensemble method,Time series forecasting,Load demand forecasting,Neural networks,Support vector regression,Random forests
AI 理解论文
溯源树
样例
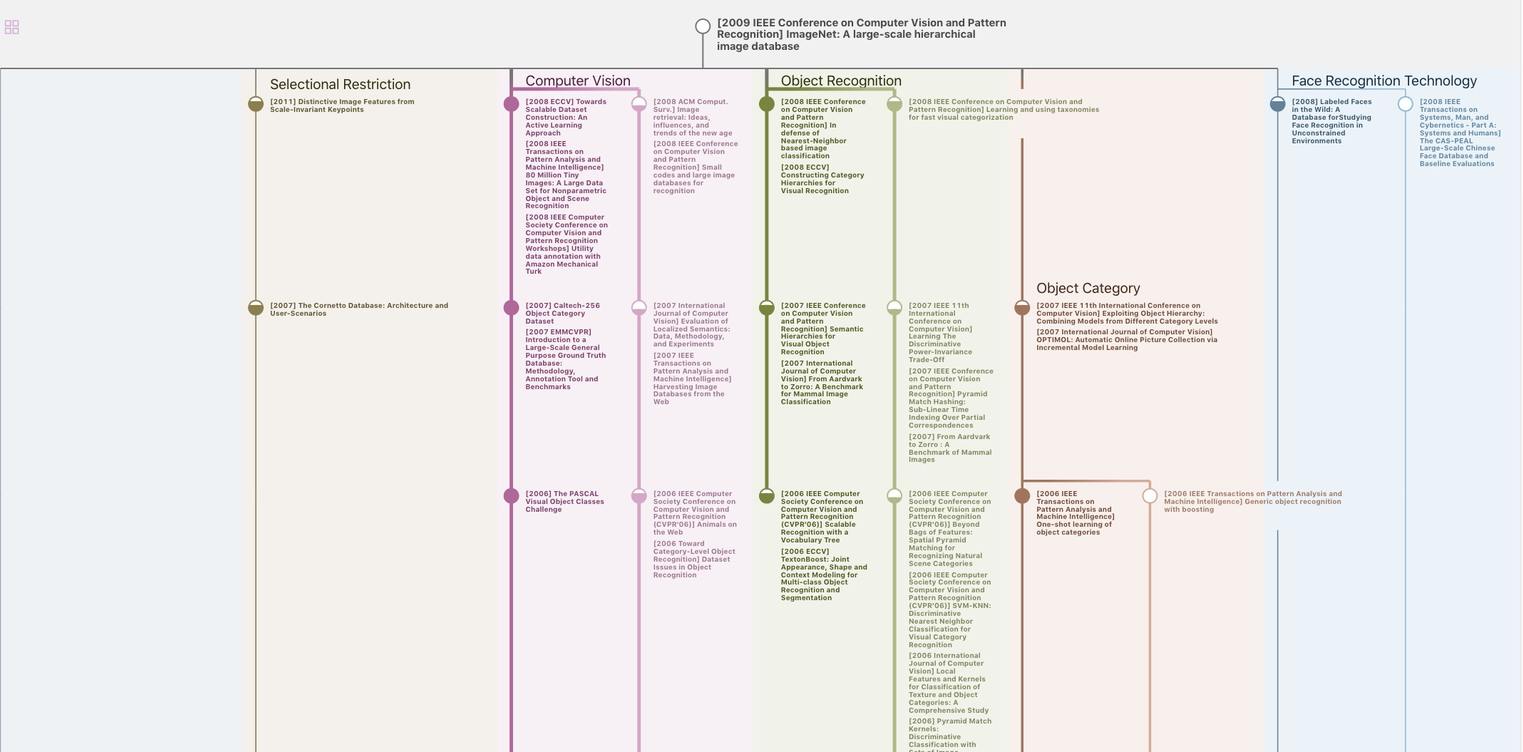
生成溯源树,研究论文发展脉络
Chat Paper
正在生成论文摘要