Measures of Similarity in Memory-Based Collaborative Filtering Recommender System: A Comparison
Proceedings of the 4th Multidisciplinary International Social Networks Conference(2017)
摘要
Collaborative filtering (CF) technique in recommender systems (RS) is a well-known and popular technique that exploits relationships between users or items to make product recommendations to an active user. The effectiveness of existing memory based algorithms depend on the similarity measure that is used to identify nearest neighbours. However, similarity measures utilize only the ratings of co-rated items while computing the similarity between a pair of users or items. In most of the e-commerce applications, the rating matrix is too sparse since even active users of an online system tend to rate only a few items of the entire set of items. Therefore, co-rated items among users are even sparser. Moreover, the ratings a user gives an individual item tells us nothing about his comprehensive interest without which the generated recommendations may not be satisfactory to a user. In order to be able to address these issues, a comprehensive study is made of the various existing measures of similarity in a collaborative filtering recommender system (CFRS) and a hierarchical categorization of products has been proposed to understand the interest of a user in a wider scope so as to provide better recommendations as well as to alleviate data sparsity.
更多查看译文
AI 理解论文
溯源树
样例
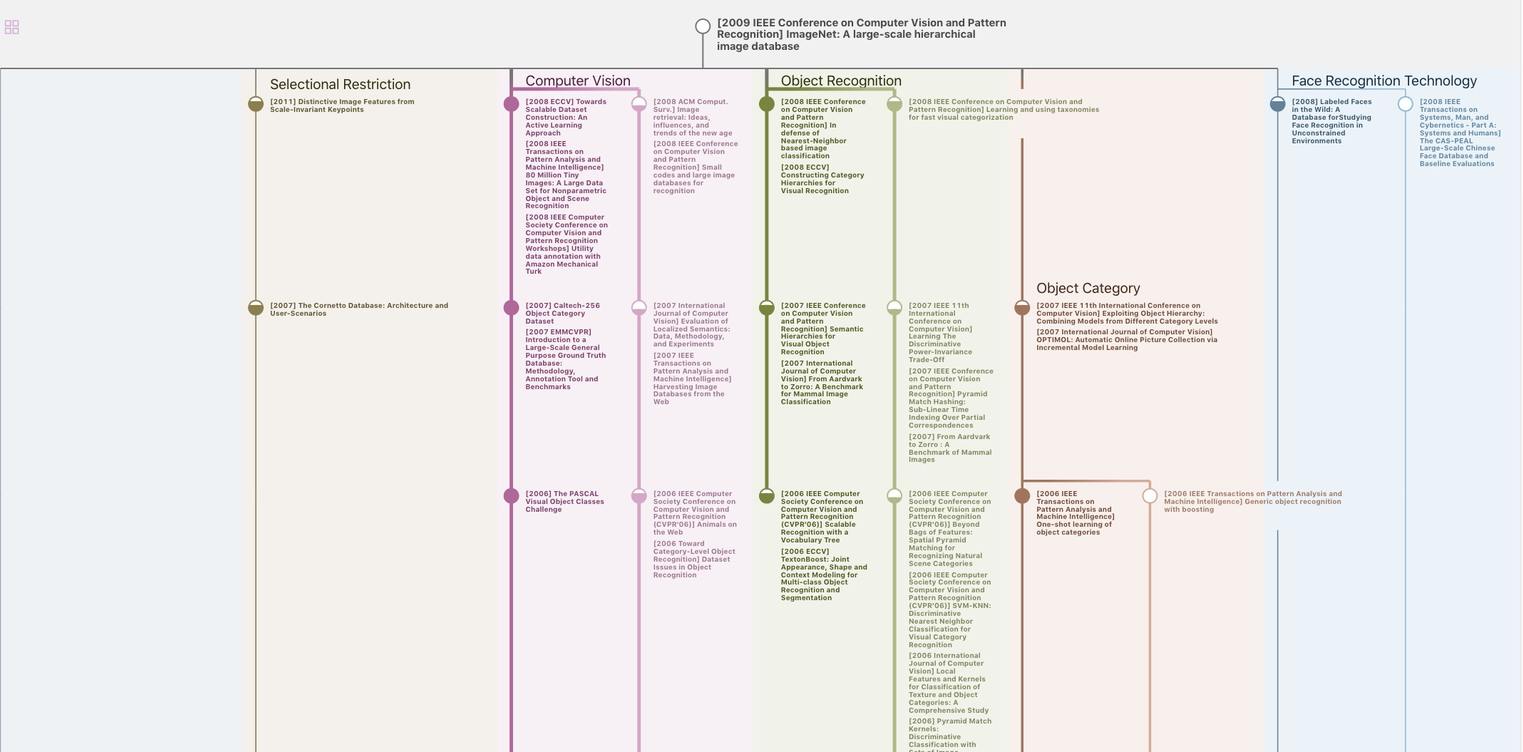
生成溯源树,研究论文发展脉络
Chat Paper
正在生成论文摘要