The decomposed normalized maximum likelihood code-length criterion for selecting hierarchical latent variable models
KDD(2019)
摘要
We propose a new model selection criterion based on the minimum description length principle in a name of the decomposed normalized maximum likelihood (DNML) criterion. Our criterion can be applied to a large class of hierarchical latent variable models, such as naïve Bayes models, stochastic block models, latent Dirichlet allocations and Gaussian mixture models, to which many conventional information criteria cannot be straightforwardly applied due to non-identifiability of latent variable models. Our method also has an advantage that it can be exactly evaluated without asymptotic approximation with small time complexity. We theoretically justify DNML in terms of hierarchical minimax regret and estimation optimality. Our experiments using synthetic data and benchmark data demonstrate the validity of our method in terms of computational efficiency and model selection accuracy. We show that our criterion especially dominate other existing criteria when sample size is small and when data are noisy.
更多查看译文
关键词
Model selection,Hierarchical latent variable models,MDL
AI 理解论文
溯源树
样例
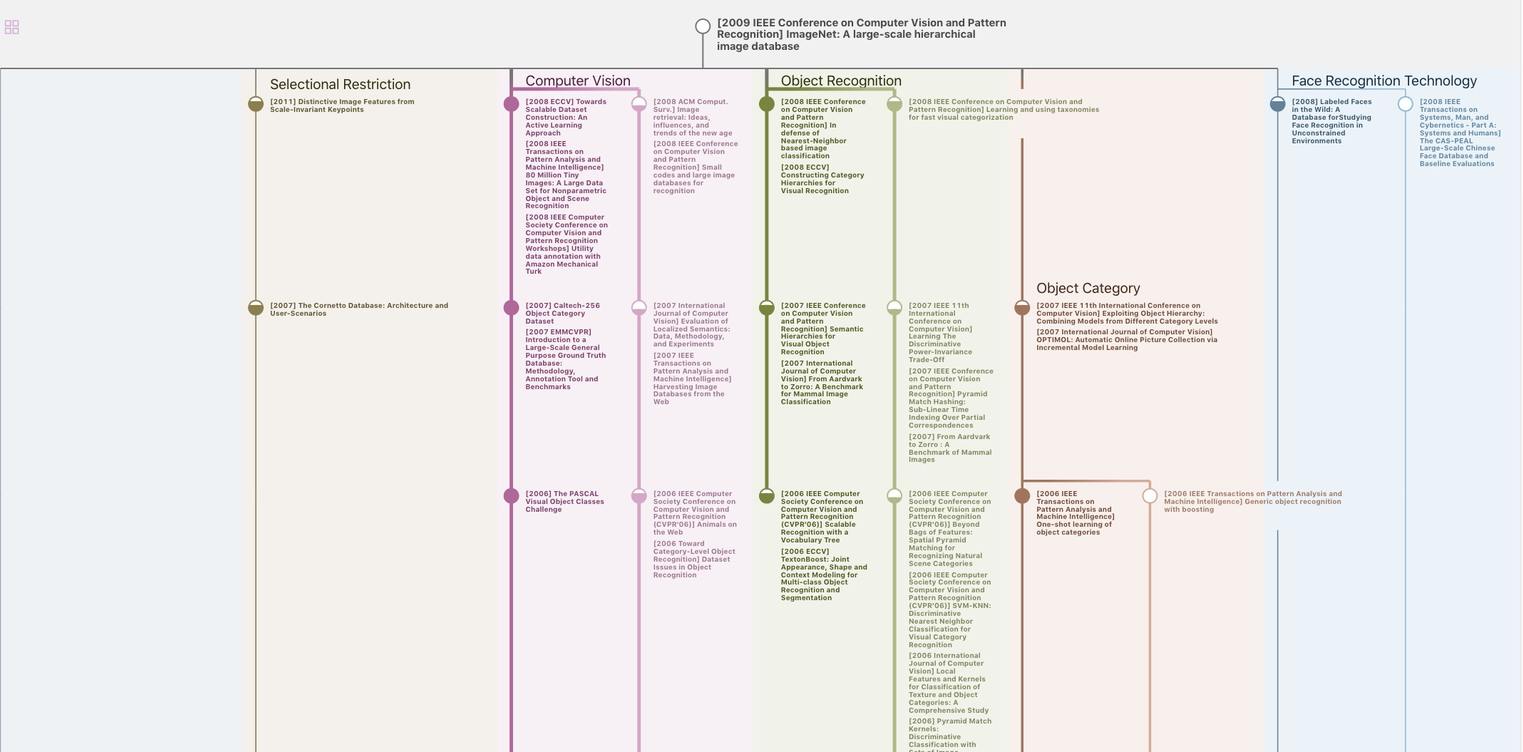
生成溯源树,研究论文发展脉络
Chat Paper
正在生成论文摘要