Activity Recognition in Individuals Walking With Assistive Devices: The Benefits of Device-Specific Models.
JMIR rehabilitation and assistive technologies(2017)
摘要
BACKGROUND:Wearable sensors gather data that machine-learning models can convert into an identification of physical activities, a clinically relevant outcome measure. However, when individuals with disabilities upgrade to a new walking assistive device, their gait patterns can change, which could affect the accuracy of activity recognition.
OBJECTIVE:The objective of this study was to assess whether we need to train an activity recognition model with labeled data from activities performed with the new assistive device, rather than data from the original device or from healthy individuals.
METHODS:Data were collected from 11 healthy controls as well as from 11 age-matched individuals with disabilities who used a standard stance control knee-ankle-foot orthosis (KAFO), and then a computer-controlled adaptive KAFO (Ottobock C-Brace). All subjects performed a structured set of functional activities while wearing an accelerometer on their waist, and random forest classifiers were used as activity classification models. We examined both global models, which are trained on other subjects (healthy or disabled individuals), and personal models, which are trained and tested on the same subject.
RESULTS:Median accuracies of global and personal models trained with data from the new KAFO were significantly higher (61% and 76%, respectively) than those of models that use data from the original KAFO (55% and 66%, respectively) (Wilcoxon signed-rank test, P=.006 and P=.01). These models also massively outperformed a global model trained on healthy subjects, which only achieved a median accuracy of 53%. Device-specific models conferred a major advantage for activity recognition.
CONCLUSIONS:Our results suggest that when patients use a new assistive device, labeled data from activities performed with the specific device are needed for maximal precision activity recognition. Personal device-specific models yield the highest accuracy in such scenarios, whereas models trained on healthy individuals perform poorly and should not be used in patient populations.
更多查看译文
关键词
activities of daily living,machine learning,orthotic devices,rehabilitation,wearables
AI 理解论文
溯源树
样例
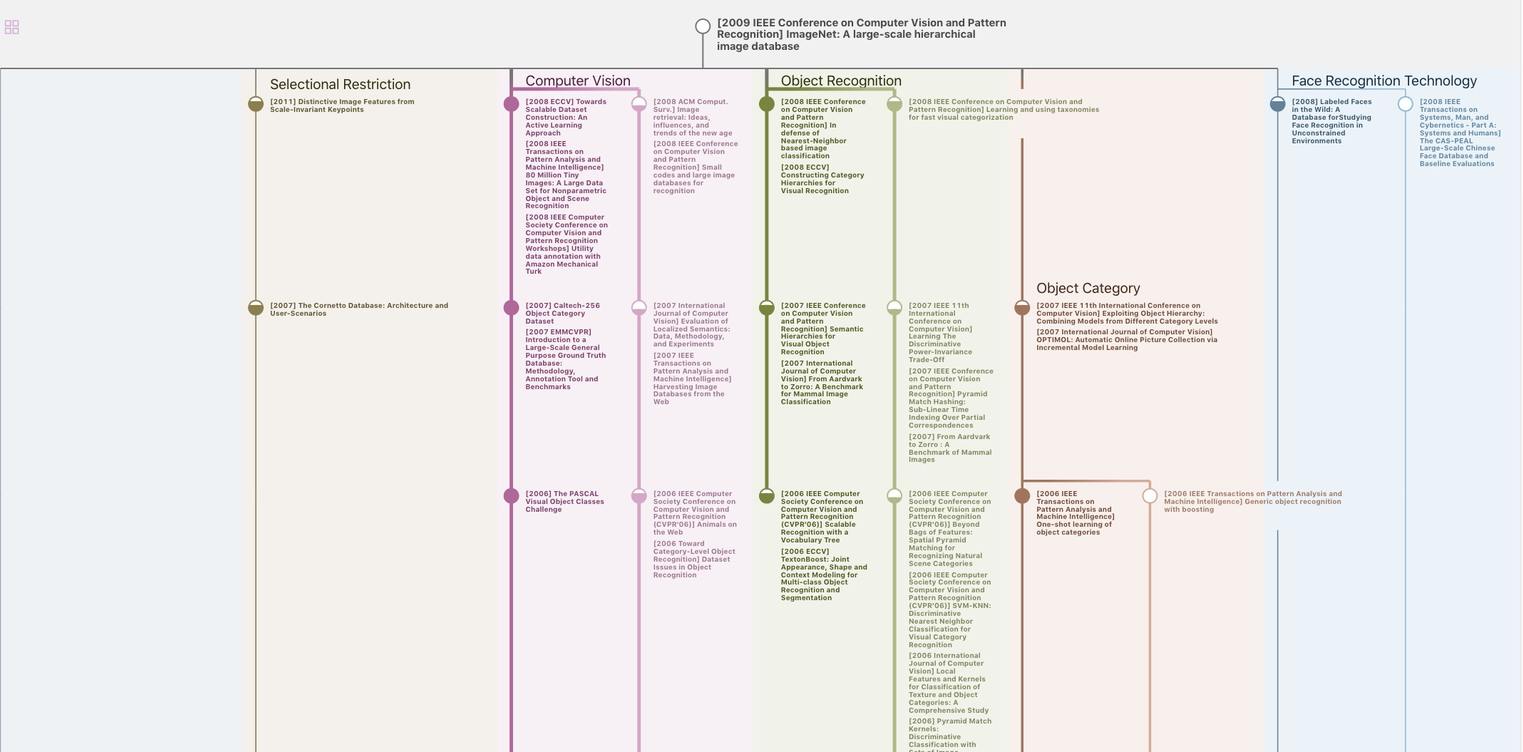
生成溯源树,研究论文发展脉络
Chat Paper
正在生成论文摘要