On the Structure of Cortical Microcircuits Inferred from Small Sample Sizes (vol 37, pg 8498, 2017)
JOURNAL OF NEUROSCIENCE(2017)
摘要
The structure in cortical microcircuits deviates from what would be expected in a purely random network, which has been seen as evidence of clustering. To address this issue, we sought to reproduce the nonrandom features of cortical circuits by considering several distinct classes of network topology, including clustered networks, networks with distance-dependent connectivity, and those with broad degree distributions. To our surprise, we found that all of these qualitatively distinct topologies could account equally well for all reported nonrandom features despite being easily distinguishable from one another at the network level. This apparent paradox was a consequence of estimating network properties given only small sample sizes. In other words, networks that differ markedly in their global structure can look quite similar locally. This makes inferring network structure from small sample sizes, a necessity given the technical difficulty inherent in simultaneous intracellular recordings, problematic. We found that a network statistic called the sample degree correlation (SDC) overcomes this difficulty. The SDC depends only on parameters that can be estimated reliably given small sample sizes and is an accurate fingerprint of every topological family. We applied the SDC criterion to data from rat visual and somatosensory cortex and discovered that the connectivity was not consistent with any of these main topological classes. However, we were able to fit the experimental data with a more general network class, of which all previous topologies were special cases. The resulting network topology could be interpreted as a combination of physical spatial dependence and nonspatial, hierarchical clustering.
更多查看译文
关键词
clustering,cortical connectivity,microcircuits,multiple patch-clamp,networks,statistics
AI 理解论文
溯源树
样例
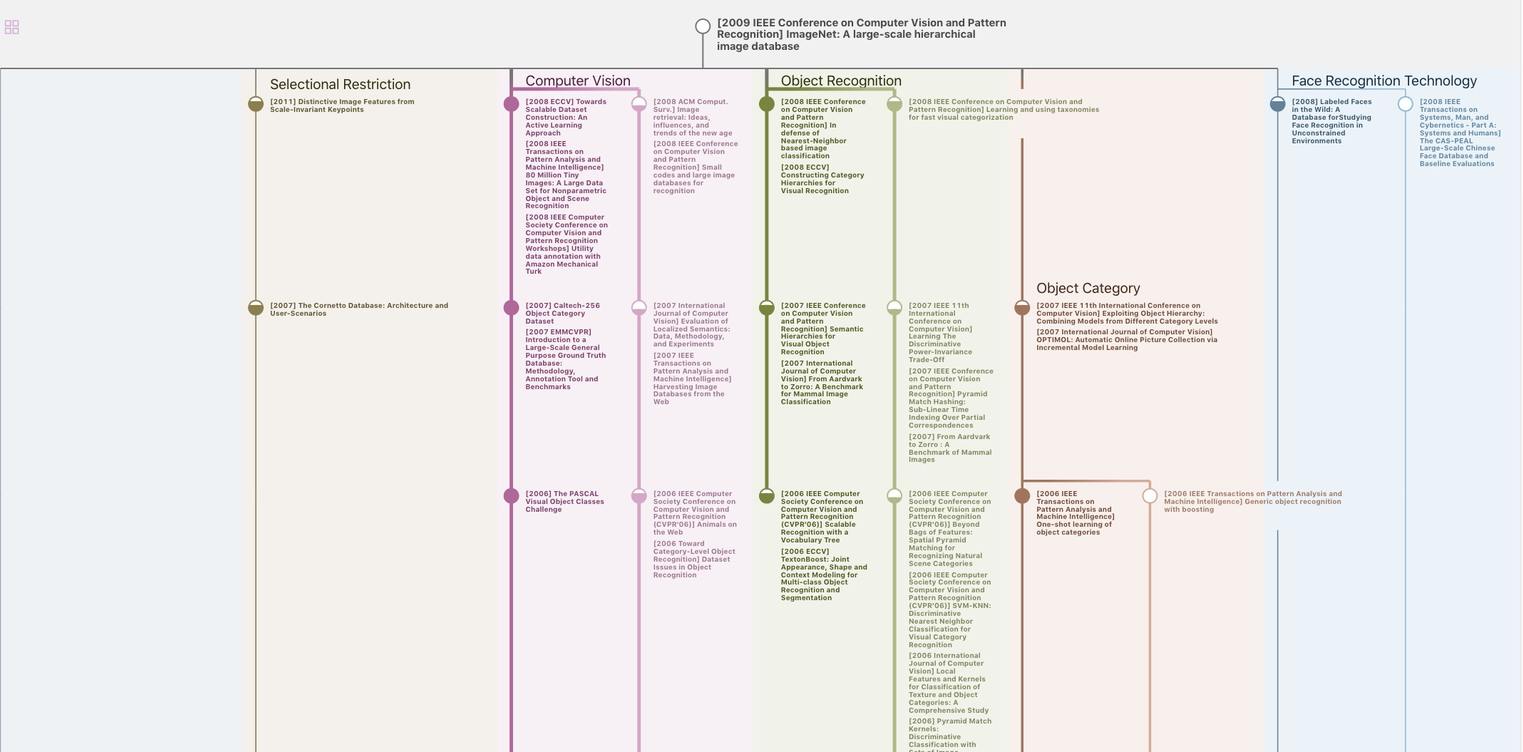
生成溯源树,研究论文发展脉络
Chat Paper
正在生成论文摘要