Differentiation between acute and chronic myocardial infarction by means of texture analysis of late gadolinium enhancement and cine cardiac magnetic resonance imaging.
European Journal of Radiology(2017)
摘要
The purpose of this study was to differentiate acute from chronic myocardial infarction using machine learning techniques and texture features extracted from cardiac magnetic resonance imaging (MRI). The study group comprised 22 cases with acute myocardial infarction (AMI) and 22 cases with chronic myocardial infarction (CMI). Cine and late gadolinium enhancement (LGE) MRI were analyzed independently to differentiate AMI from CMI. A total of 279 texture features were extracted from predefined regions of interest (ROIs): the infarcted area on LGE MRI, and the entire myocardium on cine MRI. Classification performance was evaluated by a nested cross-validation approach combining a feature selection technique with three predictive models: random forest, support vector machine (SVM) with Gaussian Kernel, and SVM with polynomial kernel. The polynomial SVM yielded the best classification performance. Receiver operating characteristic curves provided area-under-the-curve (AUC) (mean±standard deviation) of 0.86±0.06 on LGE MRI using 72 features; AMI sensitivity=0.81±0.08 and specificity=0.84±0.09. On cine MRI, AUC=0.82±0.06 using 75 features; AMI sensitivity=0.79±0.10 and specificity=0.80±0.10. We concluded that texture analysis can be used for differentiation of AMI from CMI on cardiac LGE MRI, and also on standard cine sequences in which the infarction is visually imperceptible in most cases.
更多查看译文
关键词
Cardiac MRI,Classification,Myocardial infarction,Texture analysis
AI 理解论文
溯源树
样例
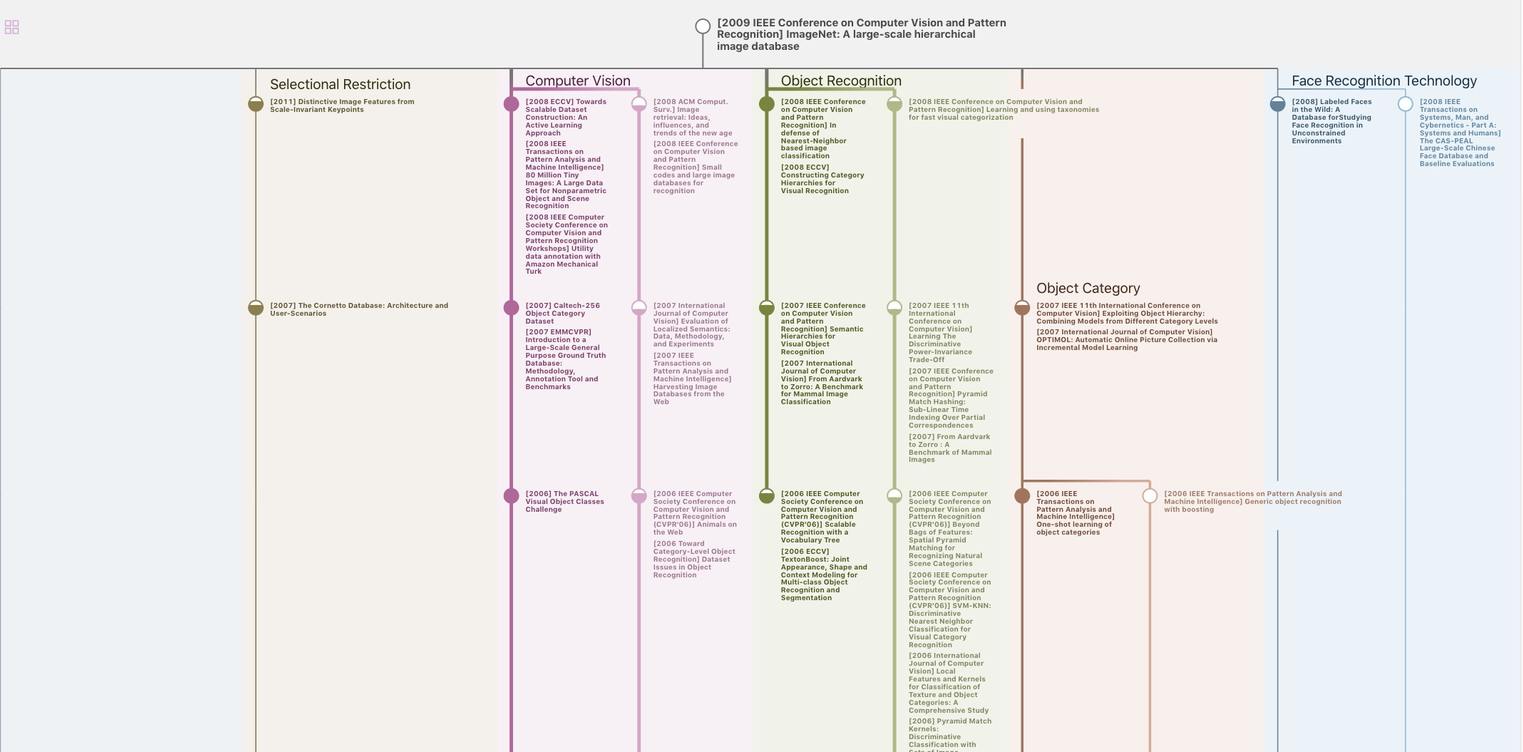
生成溯源树,研究论文发展脉络
Chat Paper
正在生成论文摘要