FP 2 -MIA: A Membership Inference Attack Free of Posterior Probability in Machine Unlearning.
Provable Security (ProvSec)(2022)
摘要
Generally speaking, machine learning is to train an ML model (original model) on a dataset to perform a certain function. But sometimes, in order to protect the data privacy of a specified user, machine unlearning requires the original model owner to delete the specified user's data in its training dataset and retrain a new model (unlearned model). However, the research of CCS'21 shows that the adversary can judge whether a data sample is deleted by comparing the prediction vectors of the original and unlearned models, thus being attacked by membership inference. To mitigate this privacy leak, CCS'21 proposes that models that only output predicted labels (i.e.,, cannot obtain model posterior probabilities) can effectively defend against existing attacks. However, our research shows that even machine unlearning models that only output labels have certain privacy risks. This paper proposes an inference attack that does not rely on posterior probability against machine unlearning, named FP2-MIA. Specifically, the adversary queries the original and unlearned models for candidate data samples respectively, and adds perturbations to them to change the predicted labels of the two models, and then the adversary uses the magnitude of the perturbations to distinguish whether they are deleted data samples. We conduct experiments on four datasets, MNIST, CIFAR10, CIFAR100 and STL10. The results show that member inference can be effectively inferred even when only the predicted labels are output, in which the AUC (Area Under Curve) index on the MNIST dataset is as high as 0.96.
更多查看译文
关键词
Membership inference attack,Label-only,Machine learning
AI 理解论文
溯源树
样例
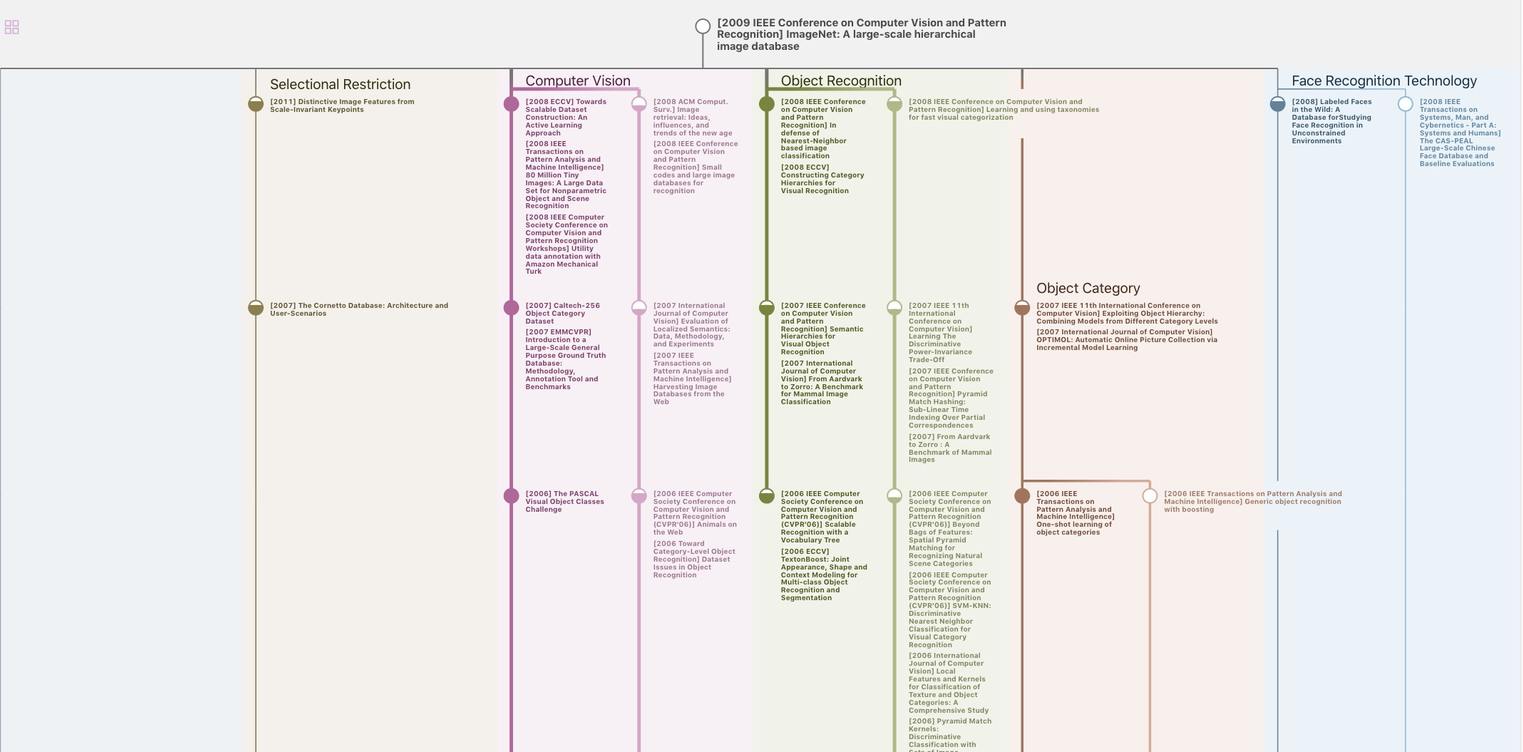
生成溯源树,研究论文发展脉络
Chat Paper
正在生成论文摘要