Adaptive Thresholding and Reweighting to Improve Domain Transfer Learning for Unbalanced Data with Applications to EEG Imbalance
2016 15th IEEE International Conference on Machine Learning and Applications (ICMLA)(2016)
摘要
Domain adaptation methods can be highly sensitive to class balance, particularly the usually unknown balance of the unlabeled test set. In this work, we analyze the effect of imbalance on a well-known algorithm, ARTL (Adaptation Regularization Transfer Learning) and propose four approaches for mitigating the adverse effects of imbalance. These include (1) balancing the training set for pseudo-label calculation, (2) applying adaptive thresholding to pseudo-label calculation, (3) using class reweighting in the optimization objective, and (4) applying adaptive thresholding to the output objective. We tested these methods with the UCI newsgroup dataset and on three types of imbalanced EEG (electroencephalogram) classification problems. We observed significant improvements, particularly for cases of extreme imbalance, which are not well addressed by standard classification techniques.
更多查看译文
关键词
transfer learning,imbalance,adaptive threshold
AI 理解论文
溯源树
样例
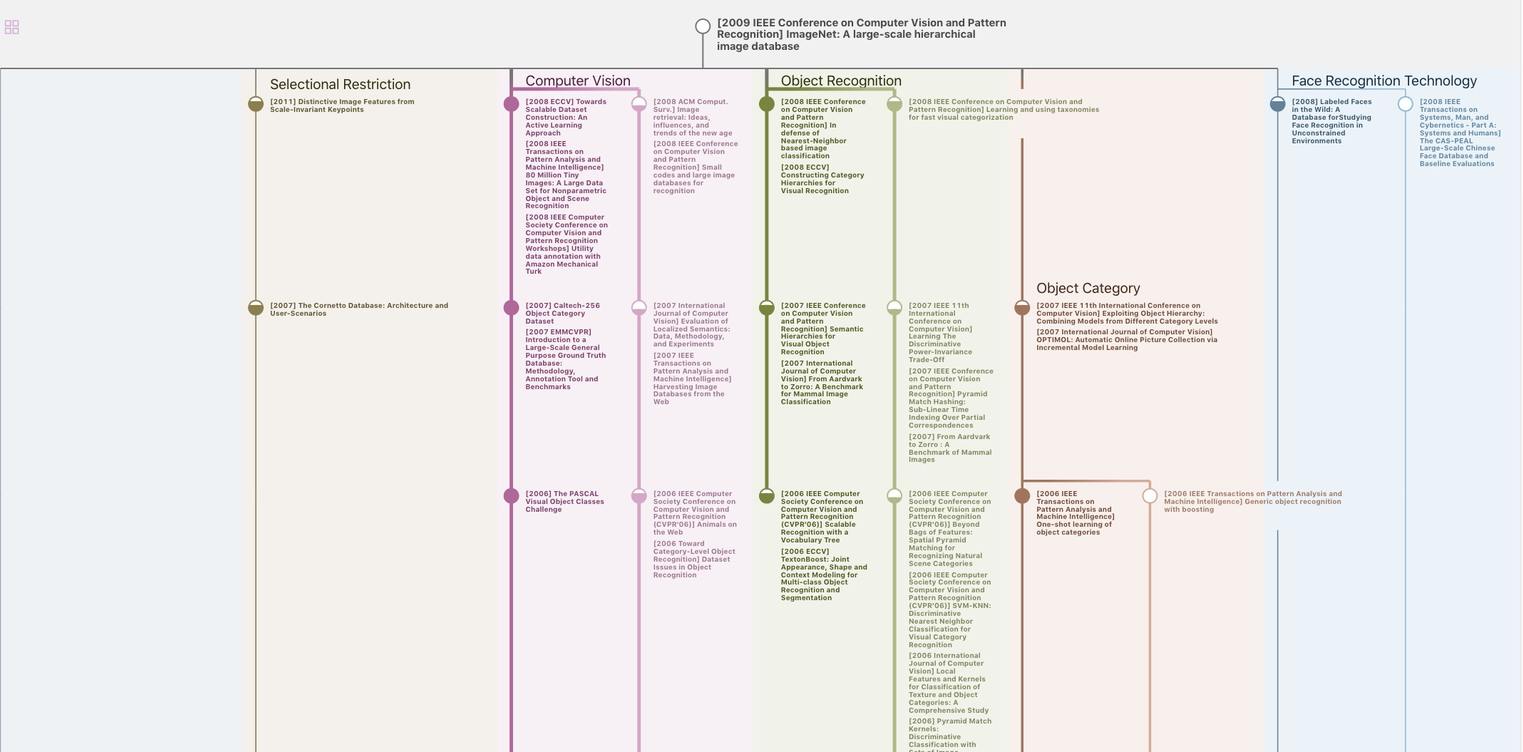
生成溯源树,研究论文发展脉络
Chat Paper
正在生成论文摘要