DERIV: Distributed In-Memory Brand Perception Tracking Framework
2016 15th IEEE International Conference on Machine Learning and Applications (ICMLA)(2016)
摘要
Social media captures voice of customers at a rapid pace. Consumer perception of a brand is crucial to its success. Current techniques for measuring brand perception using lengthy surveys of handpicked users in person, by mail, phone or online are time consuming and increasingly inadequate. A more effective technique to measure brand perception is to interpret customer voice directly from social media and other open data. In this work we present DERIV, a DistributEd, in-memoRy framework for trackIng consumer Voice based on a brand perception measure using storylines generated from open data. The framework measures perception of a brand in comparison to peer brands with in-memory distributed algorithms utilizing supervised machine learning techniques. Experiments performed with open data and models built with storylines of known peer brands show the technique as highly accurate and effective in capturing brand perception.
更多查看译文
关键词
Big Data,In-Memory Distribution,Brand Perception,Machine Learning
AI 理解论文
溯源树
样例
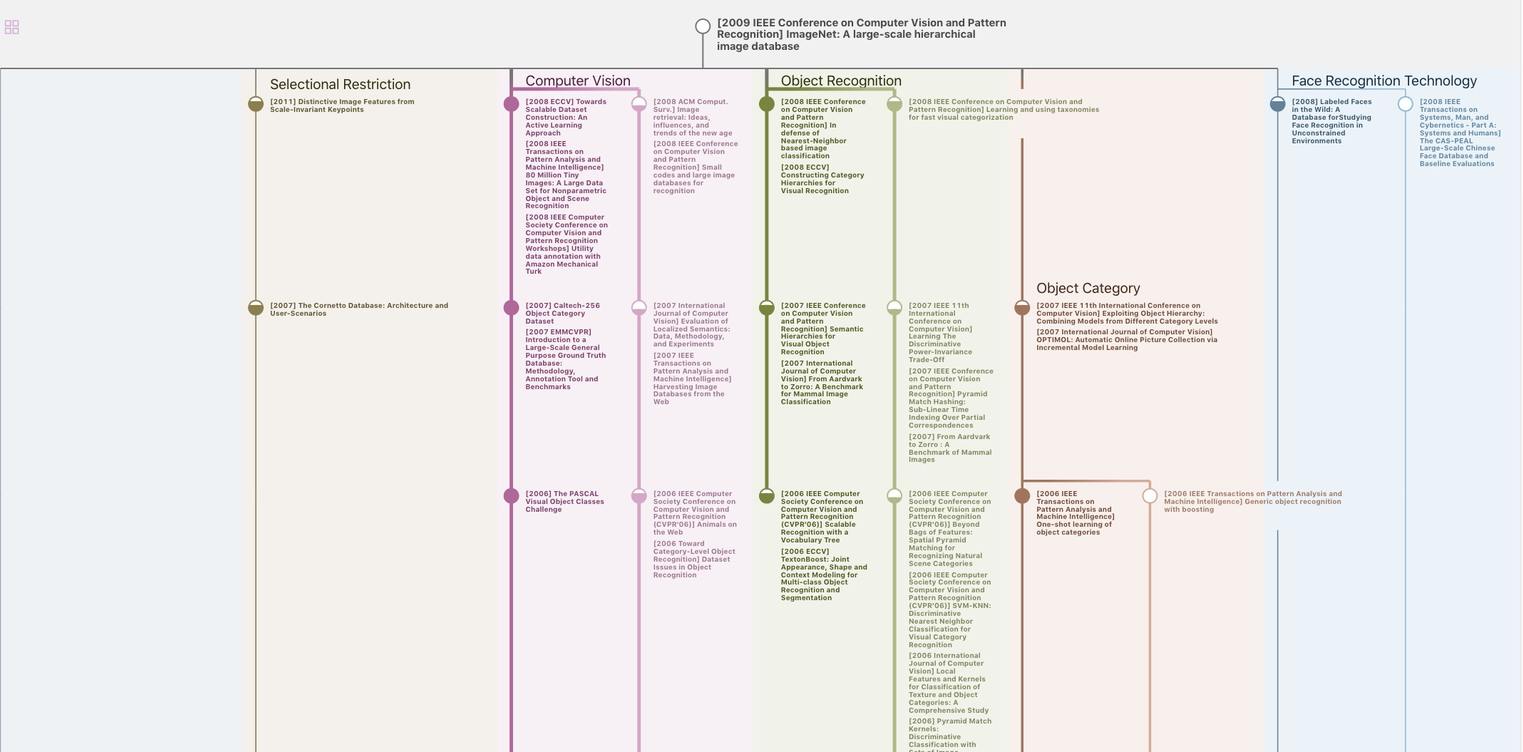
生成溯源树,研究论文发展脉络
Chat Paper
正在生成论文摘要