Sub-sampled Newton Methods with Non-uniform Sampling.
ADVANCES IN NEURAL INFORMATION PROCESSING SYSTEMS 29 (NIPS 2016)(2016)
摘要
We consider the problem of finding the minimizer of a convex function F : R-d -> R of the form F (w) := Sigma(n)(i=1) f(i) (w) + R (w) where a low-rank factorization of del(2) f(i) (w) is readily available. We consider the regime where n >> d. We propose randomized Newton-type algorithms that exploit non-uniform sub-sampling of {del(2) f(i) (w)}(i=1)(n), as well as inexact updates, as means to reduce the computational complexity, and are applicable to a wide range of problems in machine learning. Two non-uniform sampling distributions based on block norm squares and block partial leverage scores are considered. Under certain assumptions, we show that our algorithms inherit a linear-quadratic convergence rate in w and achieve a lower computational complexity compared to similar existing methods. In addition, we show that our algorithms exhibit more robustness and better dependence on problem specific quantities, such as the condition number. We empirically demonstrate that our methods are at least twice as fast as Newton's methods on several real datasets.
更多查看译文
关键词
newton methods,sub-sampled,non-uniform
AI 理解论文
溯源树
样例
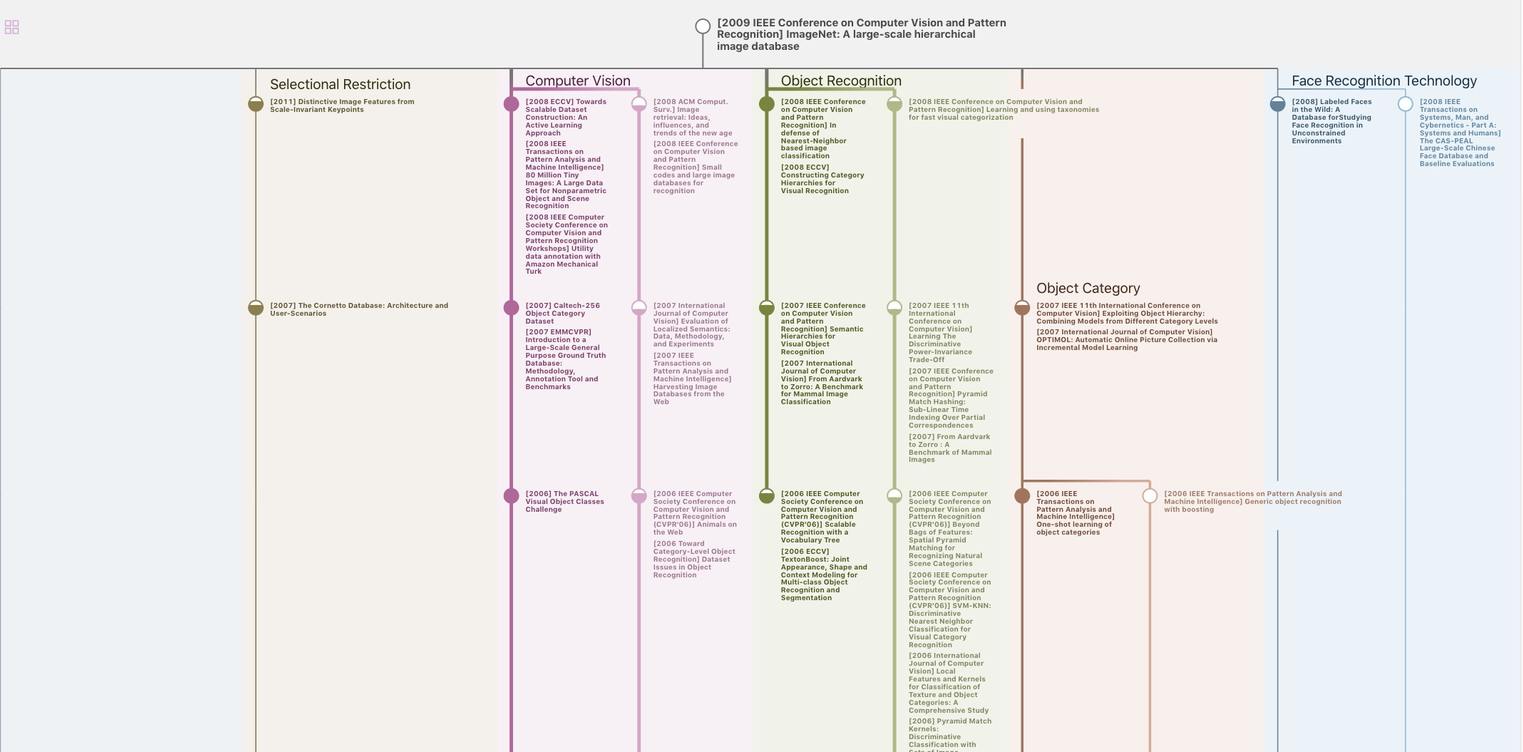
生成溯源树,研究论文发展脉络
Chat Paper
正在生成论文摘要