Convolutional Multi-directional Recurrent Network for Offline Handwritten Text Recognition
2016 15th International Conference on Frontiers in Handwriting Recognition (ICFHR)(2016)
摘要
In this paper, we propose a new network architecture called Convolutional Multi-directional Recurrent Network (CDRN) for offline handwritten text recognition. The conventional recurrent neural network model obtains the local context from limited directions, whereas we build up the multi-directional long short-term memory (MDirLSTM) module to abstract contextual information in various directions. Moreover, we develop a shortcut connection strategy in our proposed architecture for faster yet better convergence. In cooperation with the aforementioned methods, the proposed architecture also benefits from the following properties: (1) it obtains informative features of the input directly without involving hand-crafted features and segmentation, and (2) it is an end-to-end trainable model whose components are trained conjointly. We evaluate the performance of the proposed method on two databases: IAM words and IRONOFF. Our experimental results demonstrate a significant increase in recognition performance using MDirLSTM and shortcut connections, which suggests the effectiveness of these two proposed methods.
更多查看译文
关键词
Offline handwritten text recognition,multidirectional LSTM,shortcut connection,end-to-end trainable
AI 理解论文
溯源树
样例
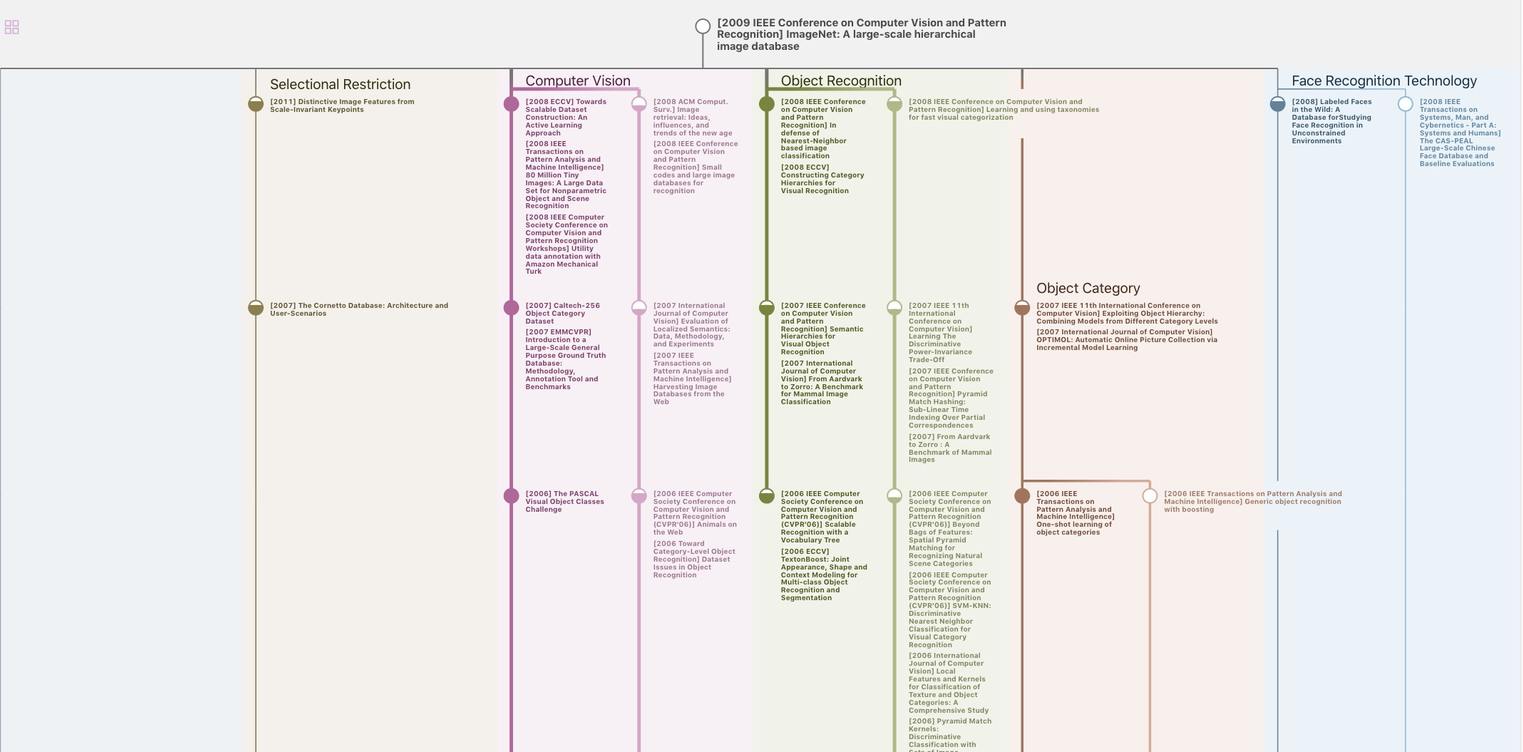
生成溯源树,研究论文发展脉络
Chat Paper
正在生成论文摘要