Comparison of deep learning models to extract silt storage dams from remote sensing images
PROCEEDINGS OF THE INSTITUTION OF CIVIL ENGINEERS-WATER MANAGEMENT(2023)
摘要
Determining the locations and shapes of silt storage dams (SSDs) is necessary before planning and constructing new ones or maintaining old ones. Google images with a spatial resolution of 0.54 m were cropped, labelled and enhanced to establish two schemes of remote sensing images that contain SSDs with different input and batch sizes. Five deep learning models (FCN (fully connected convolutional neural network, SegNet (deep convolutional encoder-decoder architecture for image segmentation), U-Net (convolutional networks for biomedical image segmentation), PSPNet (pyramid scene parsing network) and DeepLab-V3+) were constructed to extract SSDs from the images based on the two schemes. The loss curves, accuracies and extraction results derived from the five models were compared to identify the optimal model for SSD extraction. The results show that the overall accuracies, F-1 scores and mean intersections over unions obtained from DeepLab-V3+ were, respectively, 95.29%, 70.33% and 74.13% for scheme 1 (S1) and 96.29%, 73.34% and 76.99% for scheme 2 (S2), which were better than the values for other models. PSPNet had the shortest training times (128 s/step for S1 and 348 s/step for S2). An input size of 480 x 480 pixels, a batch size of 4 and 2304 images enhanced the extraction accuracy and prevented overfitting. The results provide a reference for the planning, construction and maintenance of water and soil conservation measures.
更多查看译文
关键词
artificial intelligence,dams, barrages & reservoirs,erosion,remote sensing images,silt storage dams
AI 理解论文
溯源树
样例
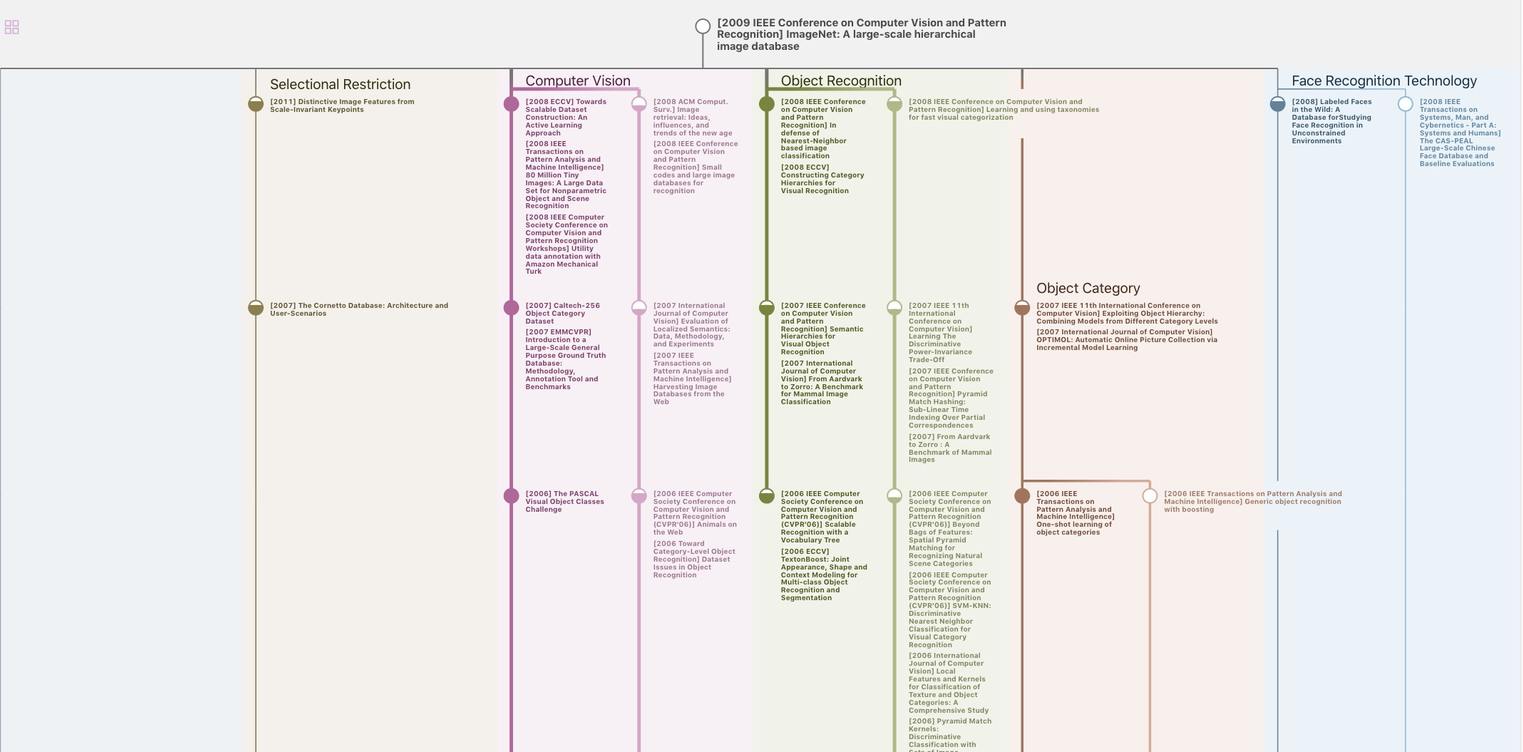
生成溯源树,研究论文发展脉络
Chat Paper
正在生成论文摘要