Joint Learning Of Similarity Graph And Image Classifier From Partial Labels
2016 Asia-Pacific Signal and Information Processing Association Annual Summit and Conference (APSIPA)(2016)
摘要
Learning of a binary classifier from partial labels is a fundamental and important task in image classification. Leveraging on recent advance in graph signal processing (GSP), a recent work poses classifier learning as a graph-signal restoration problem from partial observations, where the ill-posed problem is regularized using a graph-signal smoothness prior. In this paper, we extend this work by using the same smoothness prior to refine the underlying similarity graph also, so that the same graph-signal projected on the modified graph will be even smoother. Specifically, assuming an edge weight connecting two vertices i and j is computed as the exponential kernel of the weighted sum of feature function differences at the two vertices, we find locally "optimal" feature weights via iterative Newton's method. We show that the conditioning of the Hessian matrix reveals redundancy in the feature functions, which thus can be eliminated for improved computation efficiency. Experimental results show that our joint optimization of the classifier graph-signal and the underlying graph has better classification performance then the previous work and spectral clustering.
更多查看译文
关键词
similarity graph,image classifier,partial labels,binary classifier,image classification,graph signal processing,GSP,graph-signal smoothness prior,feature function differences,optimal feature weights,iterative Newton method,Hessian matrix,semisupervised learning
AI 理解论文
溯源树
样例
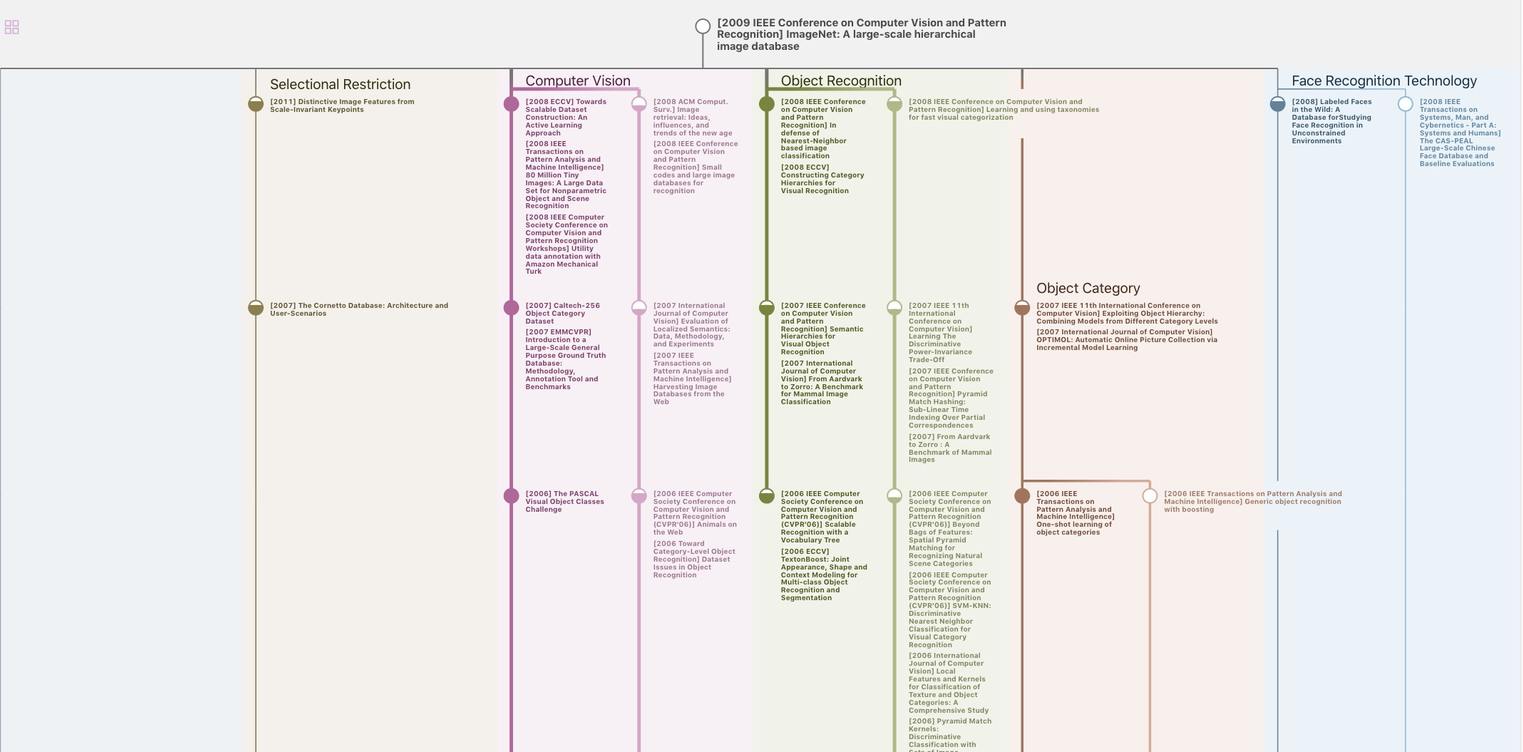
生成溯源树,研究论文发展脉络
Chat Paper
正在生成论文摘要