Enabling Intelligent Energy Management For Robots Using Publicly Available Maps
2016 IEEE/RSJ International Conference on Intelligent Robots and Systems (IROS)(2016)
摘要
Energy consumption represents one of the most basic constraints for mobile robot autonomy. We propose a new framework to predict energy consumption using information extracted from publicly available maps. This method avoids having to model internal robot configurations, which arc often unavailable, while still providing invaluable predictions for both explored and unexplored trajectories. Our approach uses a heteroscedastic Gaussian Process to model the power consumption, which explicitly accounts for variance due to exogenous latent factors such as traffic and weather conditions. We evaluate our framework on 30km of data collected from a city centre environment with a mobile robot travelling on pedestrian walkways. Results across live different test routes show an average difference between predicted and measured power consumption of 3.3%, leading to an average error of 6.6% on predictions of energy consumption. The distinct advantage of our model is our ability to predict measurement variance. The variance predictions improved by 84.3% over a benchmark.
更多查看译文
关键词
intelligent energy management,publicly available maps,mobile robot autonomy,energy consumption prediction,heteroscedastic Gaussian process,power consumption,exogenous latent factors,measurement variance prediction
AI 理解论文
溯源树
样例
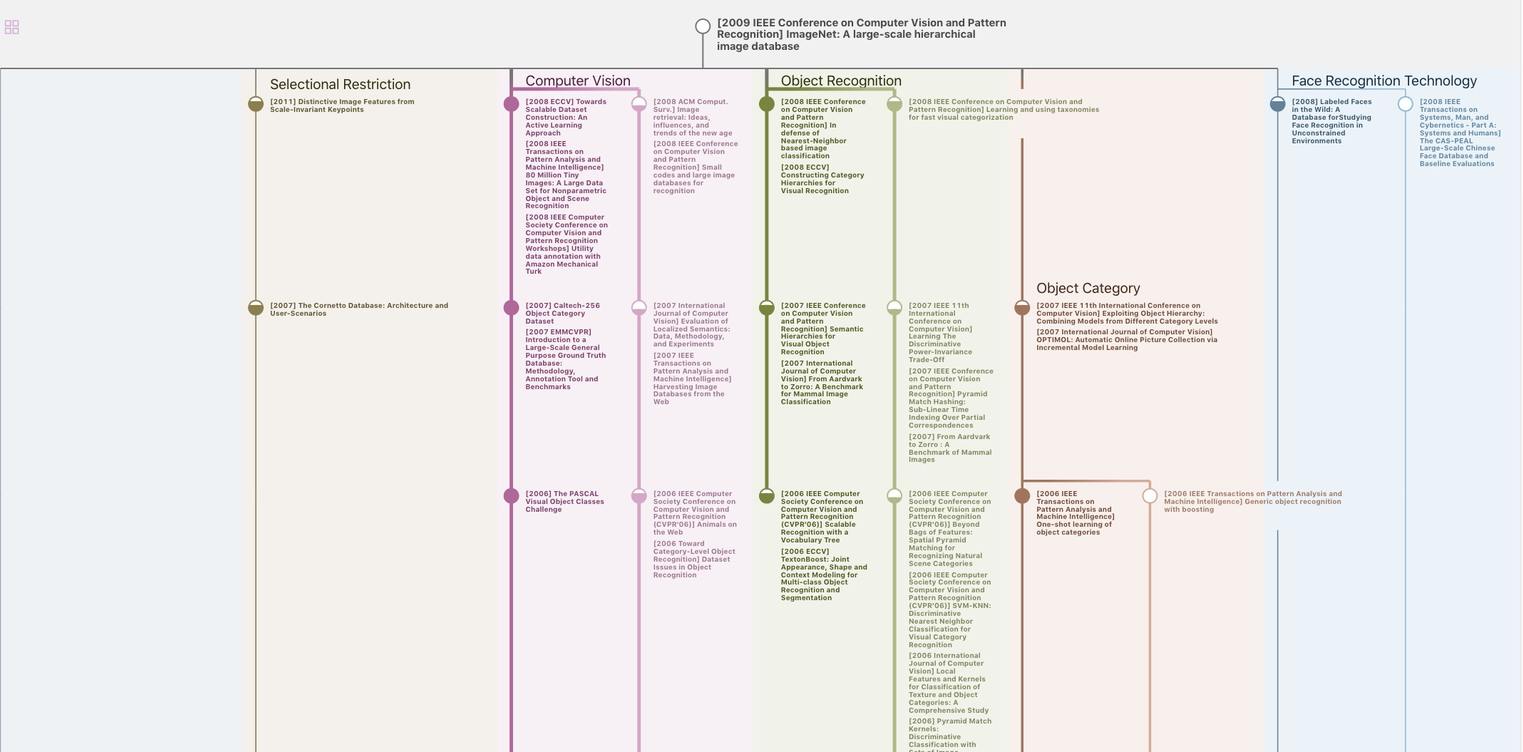
生成溯源树,研究论文发展脉络
Chat Paper
正在生成论文摘要