Generalized Information Filtering For Mav Parameter Estimation
2016 IEEE/RSJ International Conference on Intelligent Robots and Systems (IROS)(2016)
摘要
In this paper we present a new estimation algorithm that allows for the combination of information from any number of process and measurement models. This adds more flexibility to the design of the estimator and in our case avoids the need for state augmentation. We achieve this by adapting the maximum likelihood formulation of the Kalman Filter, and thereby represent all measurement models as residuals. Posing the problem in this form allows for the straightforward integration of any number of (nonlinear) constraints between two subsequent states. To solve the optimization we present a closed form recursive set of equations that directly marginalizes out information that is not required, this leads to an efficient and generic implementation. The new algorithm is applied to parameter estimation on MAVs which have two dynamic models, the MAV dynamic model and the IMU-driven model. We show the benefits and limitations of the new filtering approach on a simplified simulation example and on a real MAV system.
更多查看译文
关键词
generalized information filtering,MAV parameter estimation,estimation algorithm,estimator design,maximum likelihood formulation,Kalman filter,optimization,closed form recursive set,MAV dynamic model,IMU-driven model,microaerial vehicles
AI 理解论文
溯源树
样例
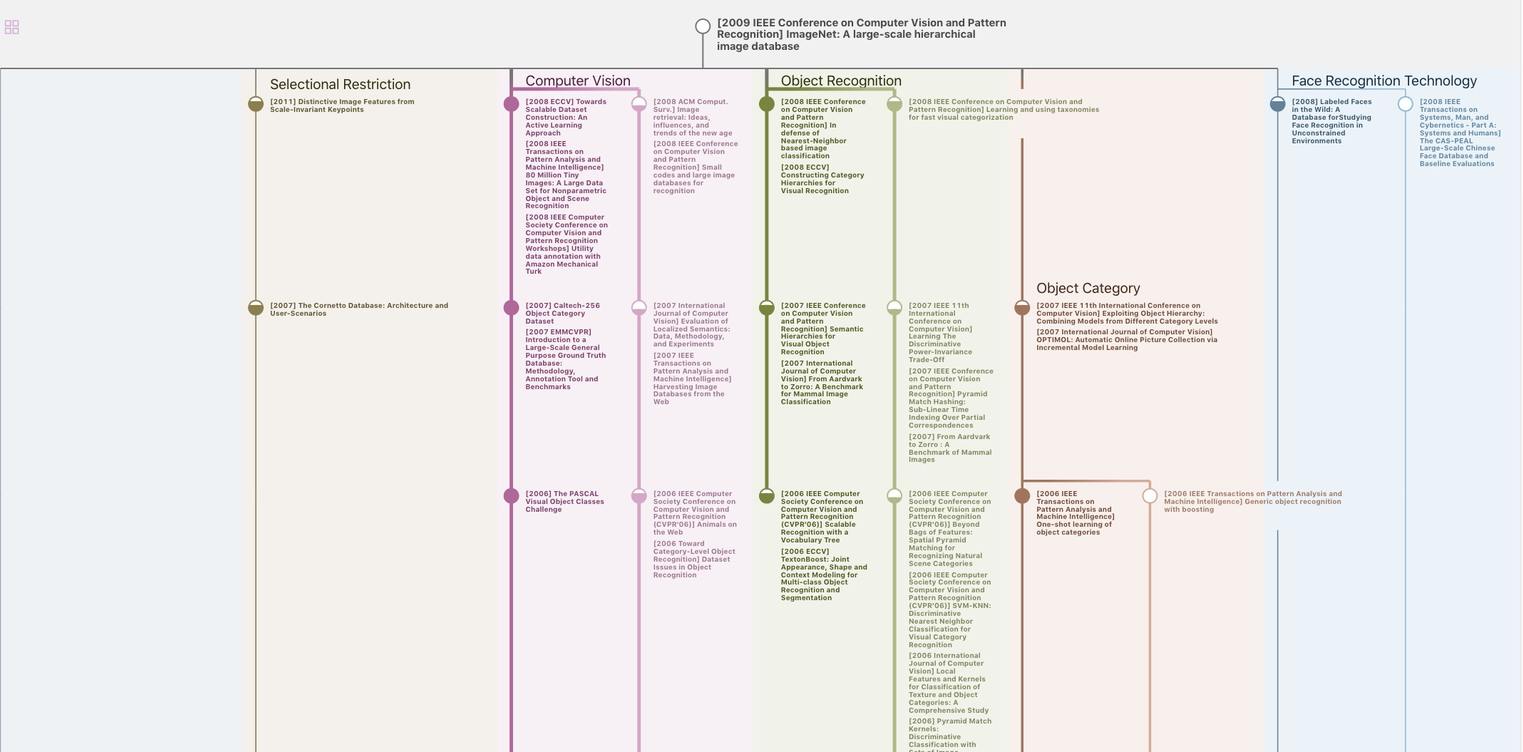
生成溯源树,研究论文发展脉络
Chat Paper
正在生成论文摘要