Saliency Analysis And Region-Of-Interest Extraction For Satellite Images By Biological Sparse Modeling
2016 IEEE International Conference on Image Processing (ICIP)(2016)
摘要
Traditional models for saliency analysis in satellite images cannot genuinely mimic the selection mechanism of human vision system. Furthermore, feature selection needs variant considering the complexity of data distribution of different satellite images thereby not being one-size-fits-all. Aiming at these problems, we propose a novel model based on sparse representation for saliency analysis with biological plausibility and preferably, our model only needs to decide the number of feature without considering feature complexity and massive parameters tuning in other feature learning algorithms. First, sparse filtering is adopted to learn a sparse dictionary for satellite images. Then, we use Incremental Coding Length (ICL) to measure the saliency contribution of every feature for the final saliency map. The region-of-interest (ROI) can be extracted based on saliency maps by thresholding segmentation. Experimental results show that our model achieves better performance compared with several traditional models for saliency analysis and ROIs extraction in satellite images.
更多查看译文
关键词
Image processing,saliency analysis,region-of-interest,sparse filtering,incremental coding length
AI 理解论文
溯源树
样例
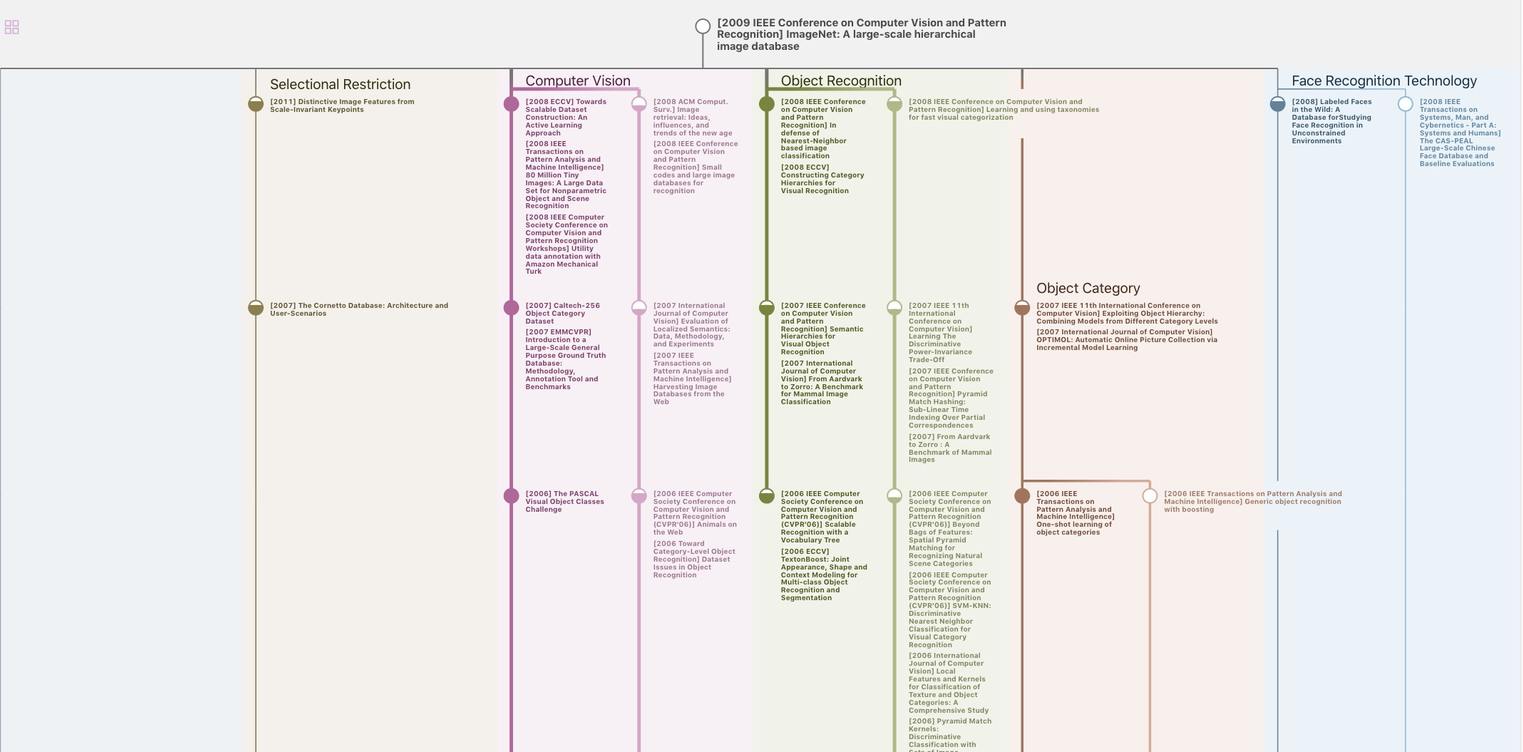
生成溯源树,研究论文发展脉络
Chat Paper
正在生成论文摘要