Large Scale Data Clustering And Graph Partitioning Via Simulated Mixing
2016 IEEE 55th Conference on Decision and Control (CDC)(2016)
摘要
In this paper, we propose a new spectral clustering algorithm relying on a simulated mixing process over a graph. In contrast to existing spectral clustering algorithms, our algorithm does not necessitate the computation of eigenvectors. Alternatively, our algorithm determines the equivalent of a linear combination of eigenvectors of the normalized similarity matrix, which are weighted by the corresponding eigenvalues obtained by the mixing process on the graph. We use the information gained from this linear combination of eigenvectors directly to partition the dataset into meaningful clusters. Simulations on real datasets show that our algorithm achieves better accuracy than standard spectral clustering methods as the number of clusters increase.
更多查看译文
关键词
Clustering algorithms,Eigenvalues and eigenfunctions,Laplace equations,Partitioning algorithms,Symmetric matrices,Atmospheric measurements,Particle measurements
AI 理解论文
溯源树
样例
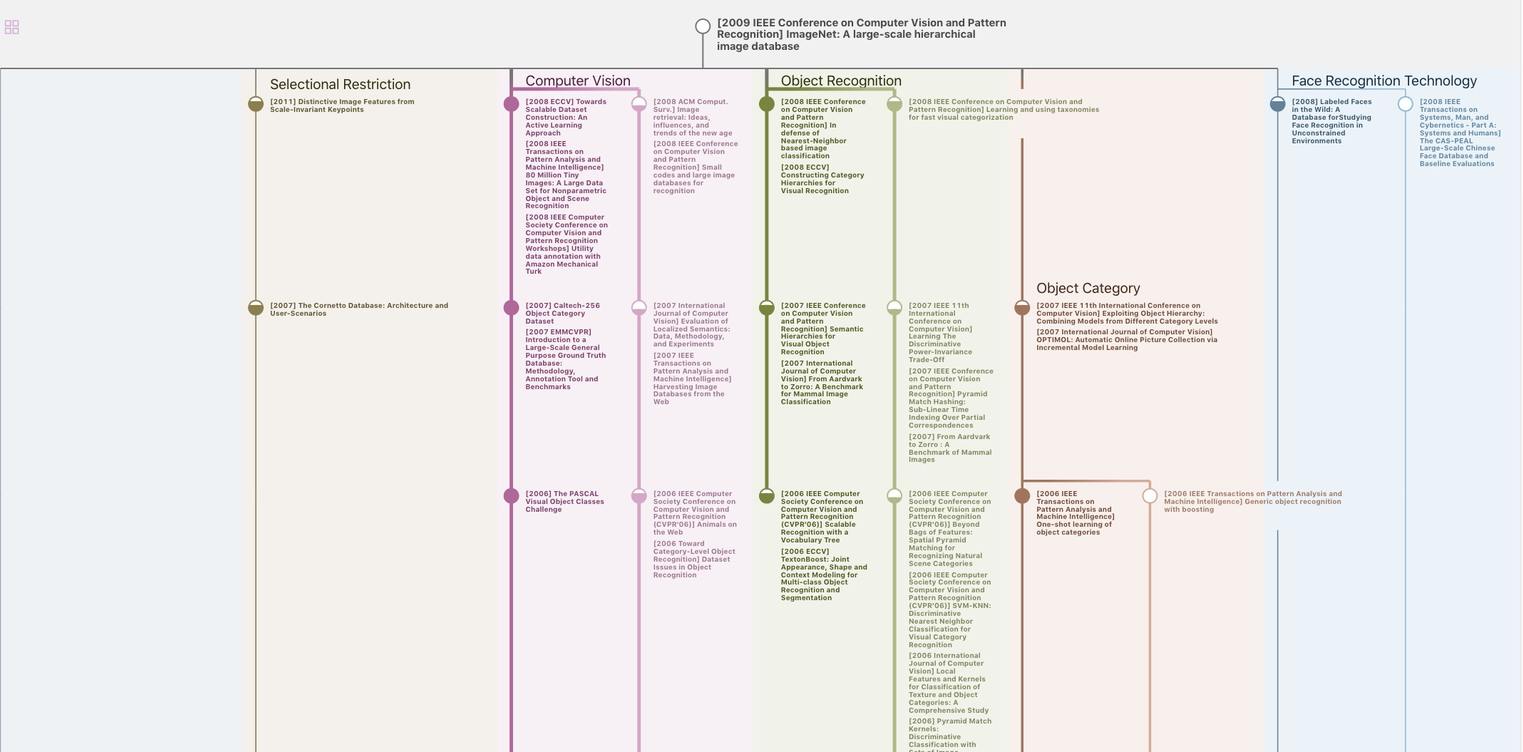
生成溯源树,研究论文发展脉络
Chat Paper
正在生成论文摘要