Stochastic Quasi-Newton Methods For Non-Strongly Convex Problems: Convergence And Rate Analysis
2016 IEEE 55TH CONFERENCE ON DECISION AND CONTROL (CDC)(2016)
摘要
Motivated by applications in optimization and machine learning, we consider stochastic quasi-Newton (SQN) methods for solving stochastic optimization problems. In the literature, the convergence analysis of these algorithms relies on strong convexity of the objective function. To our knowledge, no rate statements exist in the absence of this assumption. Motivated by this gap, we allow the objective function to be merely convex and develop a regularized SQN method. In this scheme, both the gradient mapping and the Hessian approximation are regularized at each iteration and updated alternatively. Unlike the classical regularization schemes, we allow the regularization parameter to be updated iteratively and decays to zero. Under suitable assumptions on the stepsize and regularization parameters, we show that the function value converges to its optimal value in both an almost sure and an expected-value sense. In each case, a set of regularization and steplength sequences is provided under which convergence may be guaranteed. Moreover, the rate of convergence is derived in terms of function value. Our empirical analysis on a binary classification problem shows that the proposed scheme performs well compared to both classical regularized SQN and stochastic approximation schemes.
更多查看译文
关键词
Convergence,Radio frequency,Linear programming,Optimization,Stochastic processes,Algorithm design and analysis,Approximation algorithms
AI 理解论文
溯源树
样例
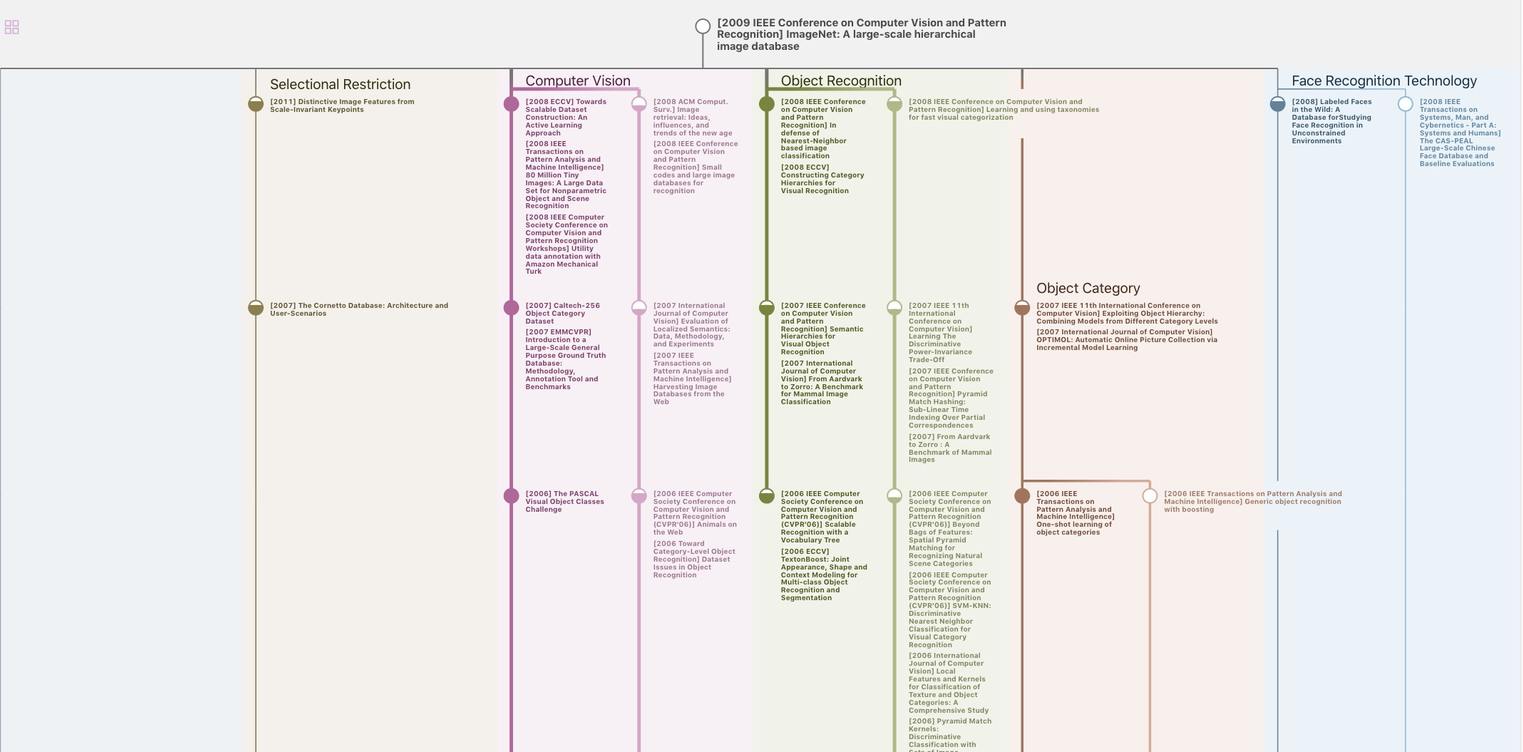
生成溯源树,研究论文发展脉络
Chat Paper
正在生成论文摘要