Active Semi-Supervised Classification Based on Multiple Clustering Hierarchies
2016 IEEE International Conference on Data Science and Advanced Analytics (DSAA)(2016)
摘要
Active semi-supervised learning can play an important role in classification scenarios in which labeled data are difficult to obtain, while unlabeled data can be easily acquired. This paper focuses on an active semi-supervised algorithm that can be driven by multiple clustering hierarchies. If there is one or more hierarchies that can reasonably align clusters with class labels, then a few queries are needed to label with high quality all the unlabeled data. We take as a starting point the well-known Hierarchical Sampling (HS) algorithm and perform changes in different aspects of the original algorithm in order to tackle its main drawbacks, including its sensitivity to the choice of a single particular hierarchy. Experimental results over many real datasets show that the proposed algorithm performs superior or competitive when compared to a number of state-of-the-art algorithms for active semi-supervised classification.
更多查看译文
关键词
active learning,classification
AI 理解论文
溯源树
样例
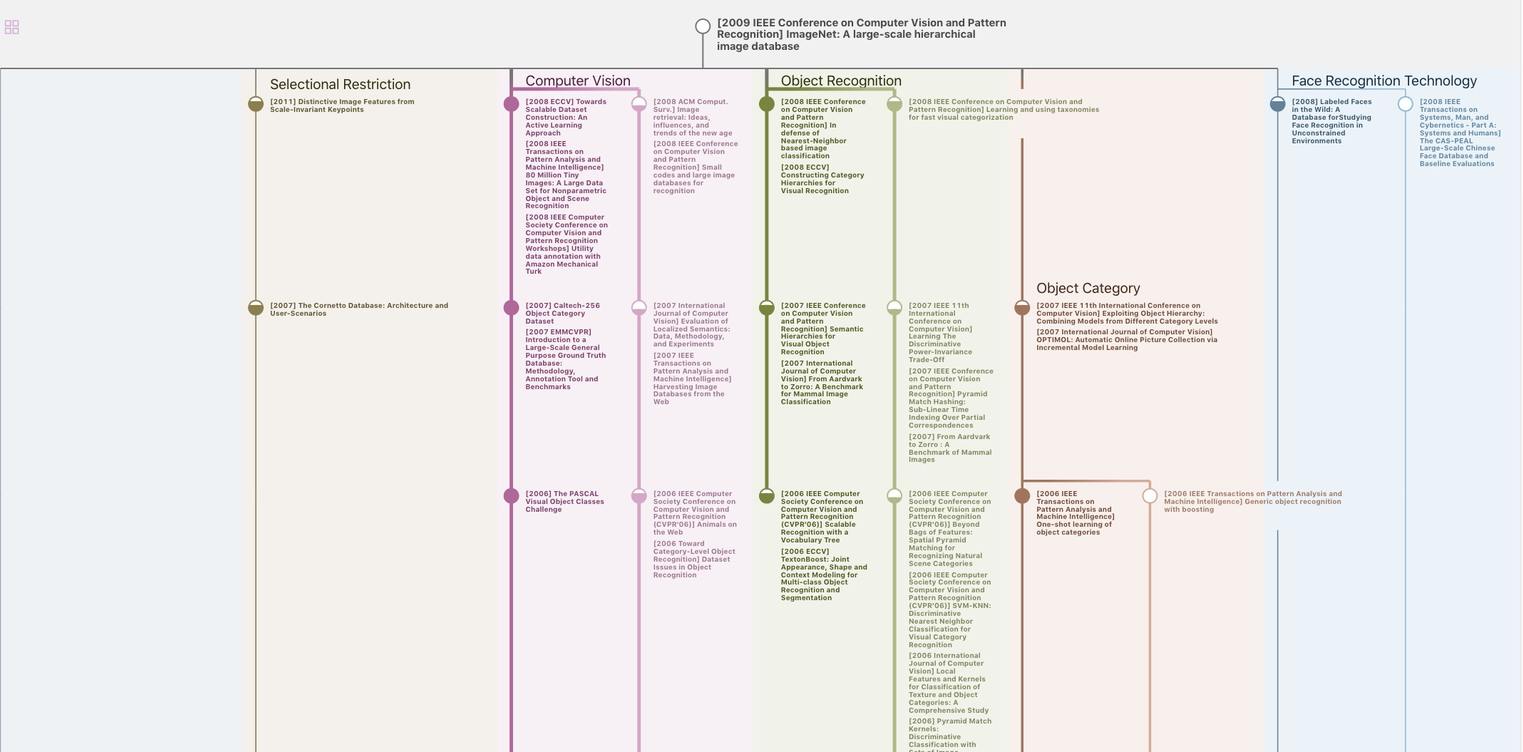
生成溯源树,研究论文发展脉络
Chat Paper
正在生成论文摘要