Harvester: Influence Optimization in Symmetric Interaction Networks
2016 IEEE International Conference on Data Science and Advanced Analytics (DSAA)(2016)
摘要
The problem of optimizing influence diffusion in a network has applications in areas such as marketing, disease control, social media analytics, and more. In all cases, an initial set of influencers are chosen so as to optimize influence propagation.While a lot of research has been devoted to the influence maximization problem, most solutions proposed to date apply on directed networks, considering the undirected case to be solvable as a special case. In this paper, we propose a novel algorithm, Harvester, that achieves results of higher quality than the state of the art on symmetric interaction networks, leveraging the particular characteristics of such networks. Harvester is based on the aggregation of instances of live-edge graphs, from which we compute the influence potential of each node. We show that this technique can be applied for both influence maximization under a known seed size and also for the dual problem of seed minimization under a target influence spread.Our experimental study with real data sets demonstrates that:(a) Harvester outperforms the state-of-the-art method, IMM,in terms of both influence spread and seed size; and (b) its variant for the seed minimization problem yields good seed size estimates, reducing the number of required trial influence spread estimations by a factor of two; and (c) it is scalable with growing graph size and robust to variant edge influence probabilities.
更多查看译文
关键词
influence diffusion optimization,symmetric interaction networks,influence propagation optimization,influence maximization problem,Harvester algorithm,live-edge graph instance aggregation,target influence spread,IMM,seed minimization problem,seed size estimation,trial influence spread estimation,graph size,edge influence probabilities
AI 理解论文
溯源树
样例
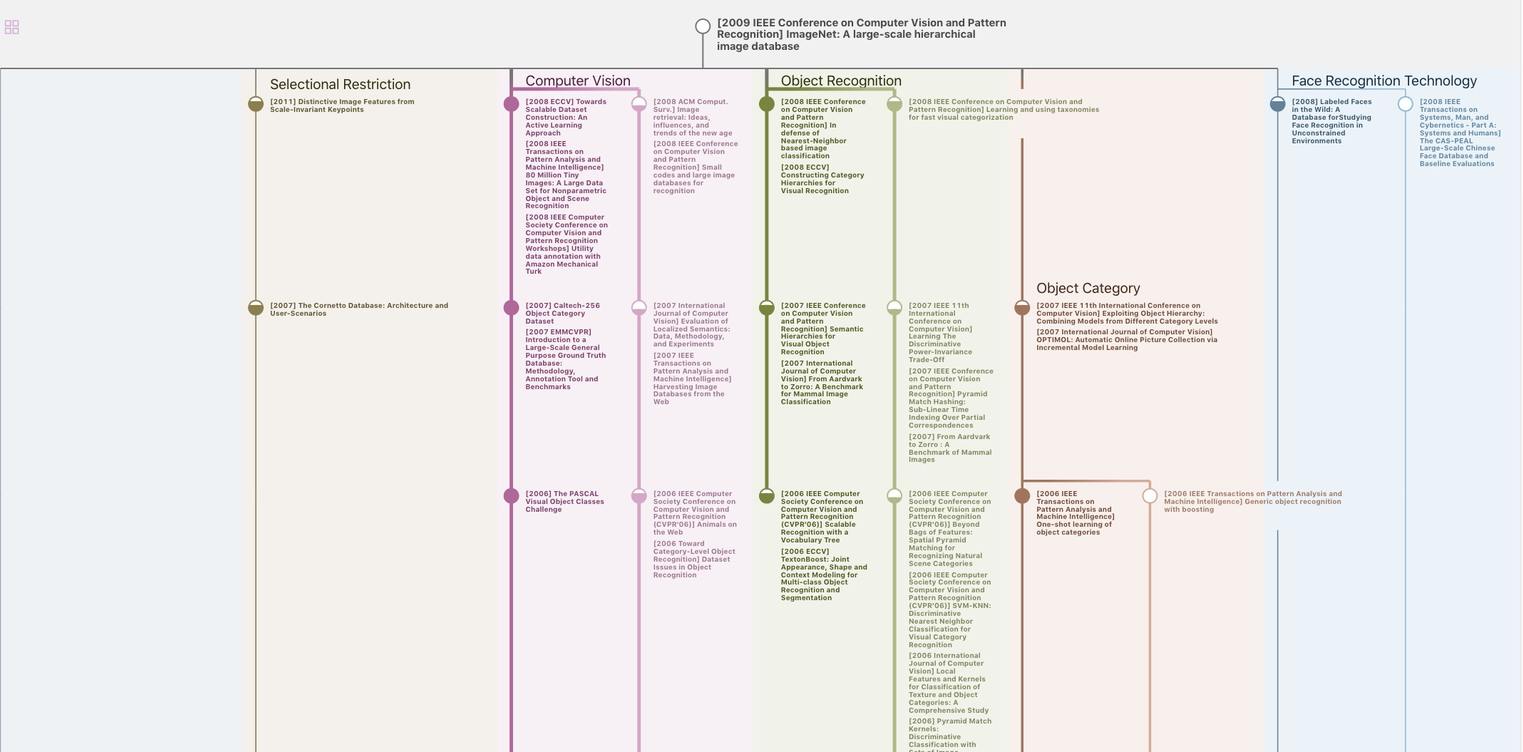
生成溯源树,研究论文发展脉络
Chat Paper
正在生成论文摘要