Estimation Of The Spatial Information In Gaussian Model Based Audio Source Separation Using Weighted Spectral Bases
2016 24th European Signal Processing Conference (EUSIPCO)(2016)
摘要
In Gaussian model based audio source separation, source spatial images are modeled by Gaussian distributions. The covariance matrices of the distributions are represented by source variances and spatial covariance matrices. Accordingly, the likelihood of observed mixtures of independent source signals is parametrized by the variances and the covariance matrices. The separation is performed by estimating the parameters and applying multichannel Wiener filtering. Assuming that spectral basis matrices trained on source power spectra are available, this work proposes a method to estimate the parameters by maximizing the likelihood using Expectation-Maximization. In terms of normalization, the variances are estimated applying singular value decomposition. Furthermore, by building weighted matrices from vectors of the trained matrices, semi-supervised nonnegative matrix factorization is applied to estimate the spatial covariance matrices. The experimental results prove the efficiency of the proposed algorithm in reverberant environments.
更多查看译文
关键词
Spectral bases,nonnegative matrix factorization,spatial covariance matrix,audio source separation
AI 理解论文
溯源树
样例
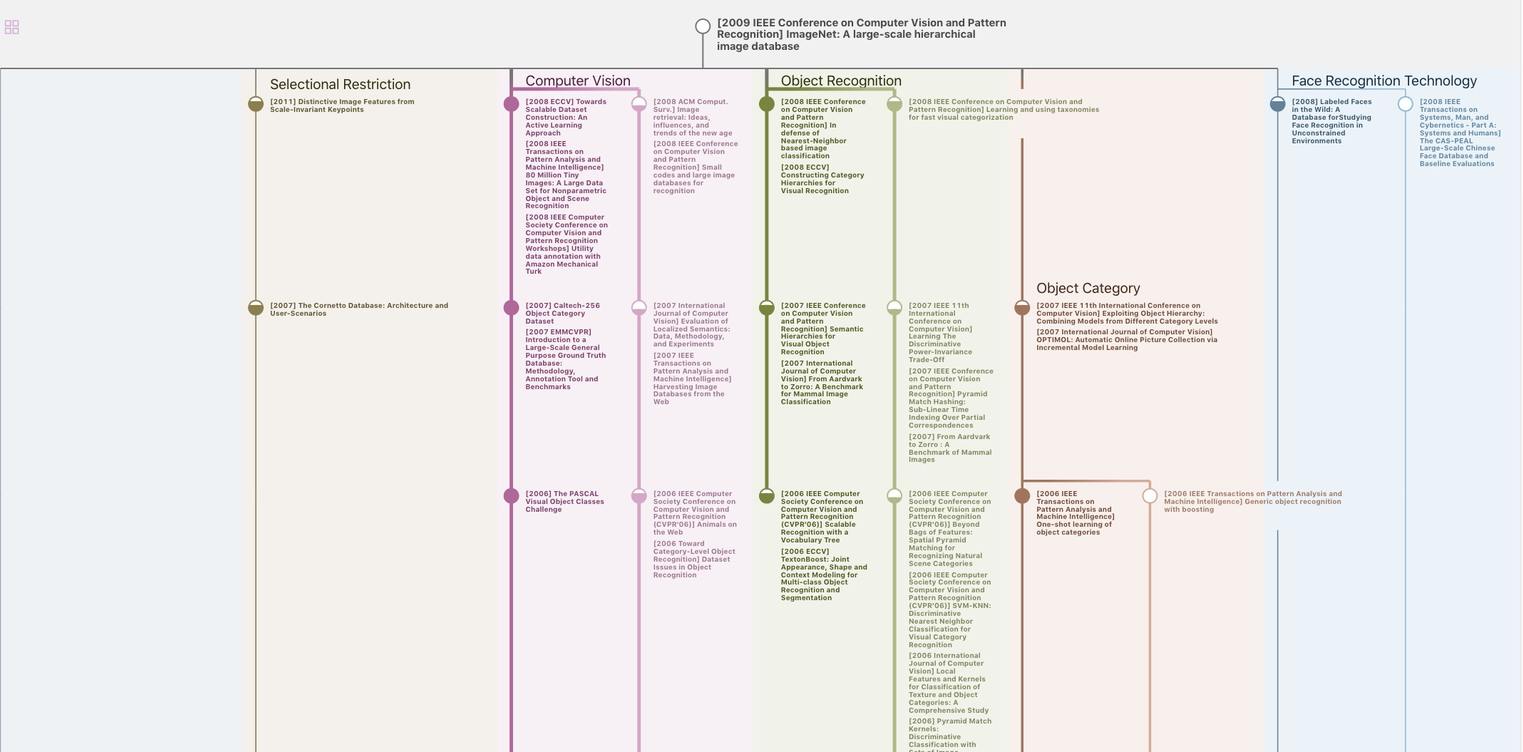
生成溯源树,研究论文发展脉络
Chat Paper
正在生成论文摘要