Topology-Aware Performance Optimization And Modeling Of Adaptive Mesh Refinement Codes For Exascale
SC(2016)
摘要
We introduce a topology-aware performance optimization and modeling workflow for AMR simulation that includes two new modeling tools, ProgrAMR and Mota Mapper, which interface with the BoxLib AMR framework and the SST-macro network simulator. ProgrAMR allows us to generate and model the execution of task dependency graphs from high-level specifications of AMR-based applications, which we demonstrate by analyzing two example AMR-based multigrid solvers with varying degrees of asynchrony. Mota Mapper generates multi-objective, network topology-aware box mappings, which we apply to optimize the data layout for the example multigrid solvers. While the sensitivity of these solvers to layout and execution strategy appears to be modest for balanced scenarios, the impact of better mapping algorithms can be significant when performance is highly constrained by network hop latency. Furthermore, we show that network latency in the multigrid bottom solve is the main contributing factor preventing good scaling on exascale-class machines.
更多查看译文
关键词
topology-aware performance optimization,adaptive mesh refinement codes,AMR simulation,ProgrAMR modeling tool,Mota Mapper modeling tool,BoxLib AMR framework,SSTmacronetwork simulator,task dependency graphs,AMR-based multigrid solvers,multiobjective network topology-aware box mappings,data layout optimization,network hop latency,exascale-class machines
AI 理解论文
溯源树
样例
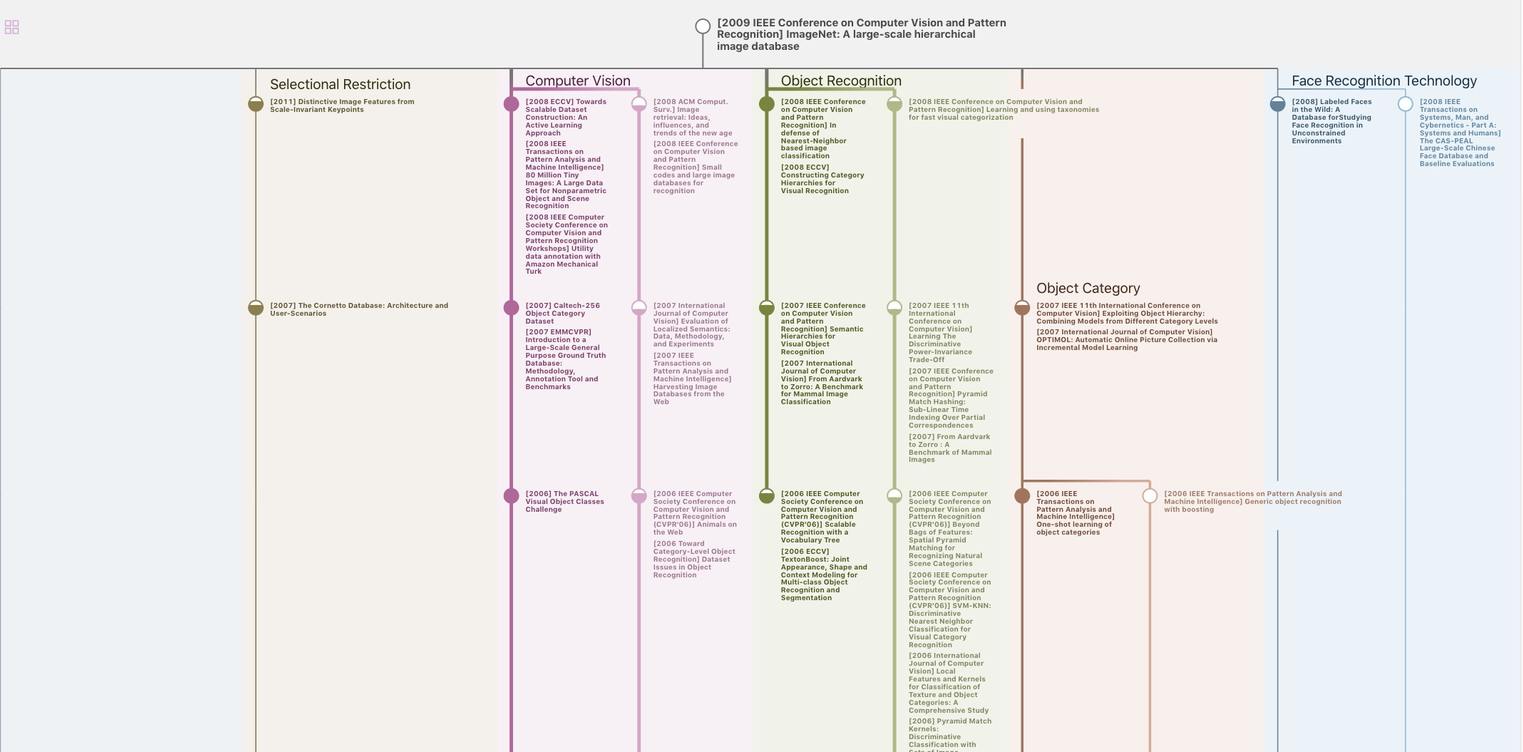
生成溯源树,研究论文发展脉络
Chat Paper
正在生成论文摘要