Spatially-Intensive Decision Tree Prediction of Traffic Flow across the Entire UK Road Network.
DS-RT(2016)
摘要
This paper introduces a novel approach to predicting UK-wide daily traffic counts on all roads in England and Wales, irrespective of sensor data availability. A key finding of this research is that many roads in a network may have no local connection, but may still share some common law, and this fact can be exploited to improve simulation. In this paper we show that: (1) Traffic counts are a function of dependant spatial, temporal and neighbourhood variables, (2) Large open-source data, such as school location and public transport hubs can, with appropriate GIS and machine learning, assist the prediction of traffic counts, (3) Real-time simulation can be scaled-up to large networks with the aid of machine learning and, (4) Such techniques can be employed in real-world tools. Validation of the proposed approach demonstrates an 88.2% prediction accuracy on traffic counts across the UK.
更多查看译文
关键词
REPTree,Traffic Flow,Real Estate,Machine Learning
AI 理解论文
溯源树
样例
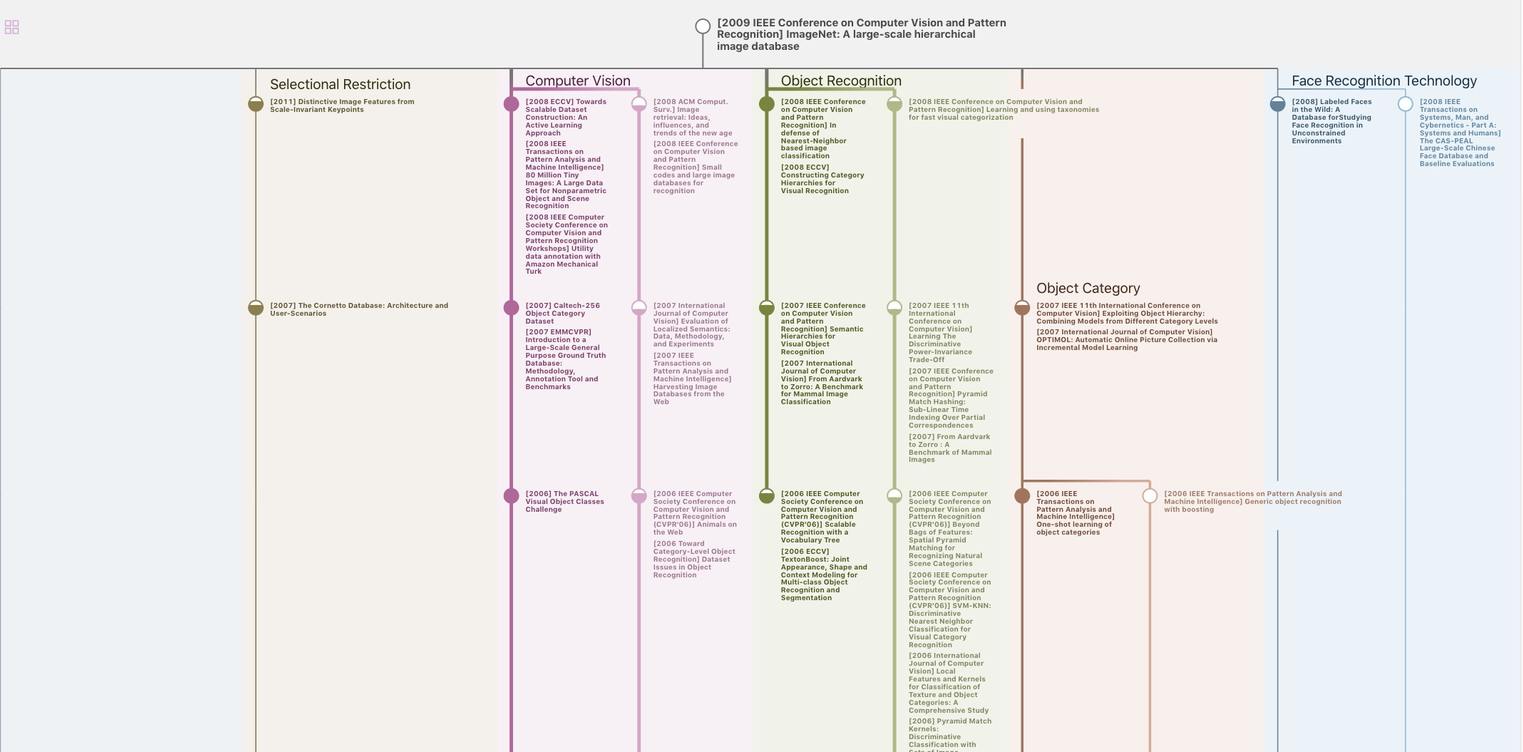
生成溯源树,研究论文发展脉络
Chat Paper
正在生成论文摘要