Syntax or semantics? knowledge-guided joint semantic frame parsing
2016 IEEE Spoken Language Technology Workshop (SLT)(2016)
摘要
Spoken language understanding (SLU) is a core component of a spoken dialogue system, which involves intent prediction and slot filling and also called semantic frame parsing. Recently recurrent neural networks (RNN) obtained strong results on SLU due to their superior ability of preserving sequential information over time. Traditionally, the SLU component parses semantic frames for utterances considering their flat structures, as the underlying RNN structure is a linear chain. However, natural language exhibits linguistic properties that provide rich, structured information for better understanding. This paper proposes to apply knowledge-guided structural attention networks (K-SAN), which additionally incorporate non-flat network topologies guided by prior knowledge, to a language understanding task. The model can effectively figure out the salient substructures that are essential to parse the given utterance into its semantic frame with an attention mechanism, where two types of knowledge, syntax and semantics, are utilized. The experiments on the benchmark Air Travel Information System (ATIS) data and the conversational assistant Cortana data show that 1) the proposed K-SAN models with syntax or semantics outperform the state-of-the-art neural network based results, and 2) the improvement for joint semantic frame parsing is more significant, because the structured information provides rich cues for sentence-level understanding, where intent prediction and slot filling can be mutually improved.
更多查看译文
关键词
Spoken language understanding,joint semantic frame parsing,knowledge-guided structural attention networks,deep learning,spoken dialogue system
AI 理解论文
溯源树
样例
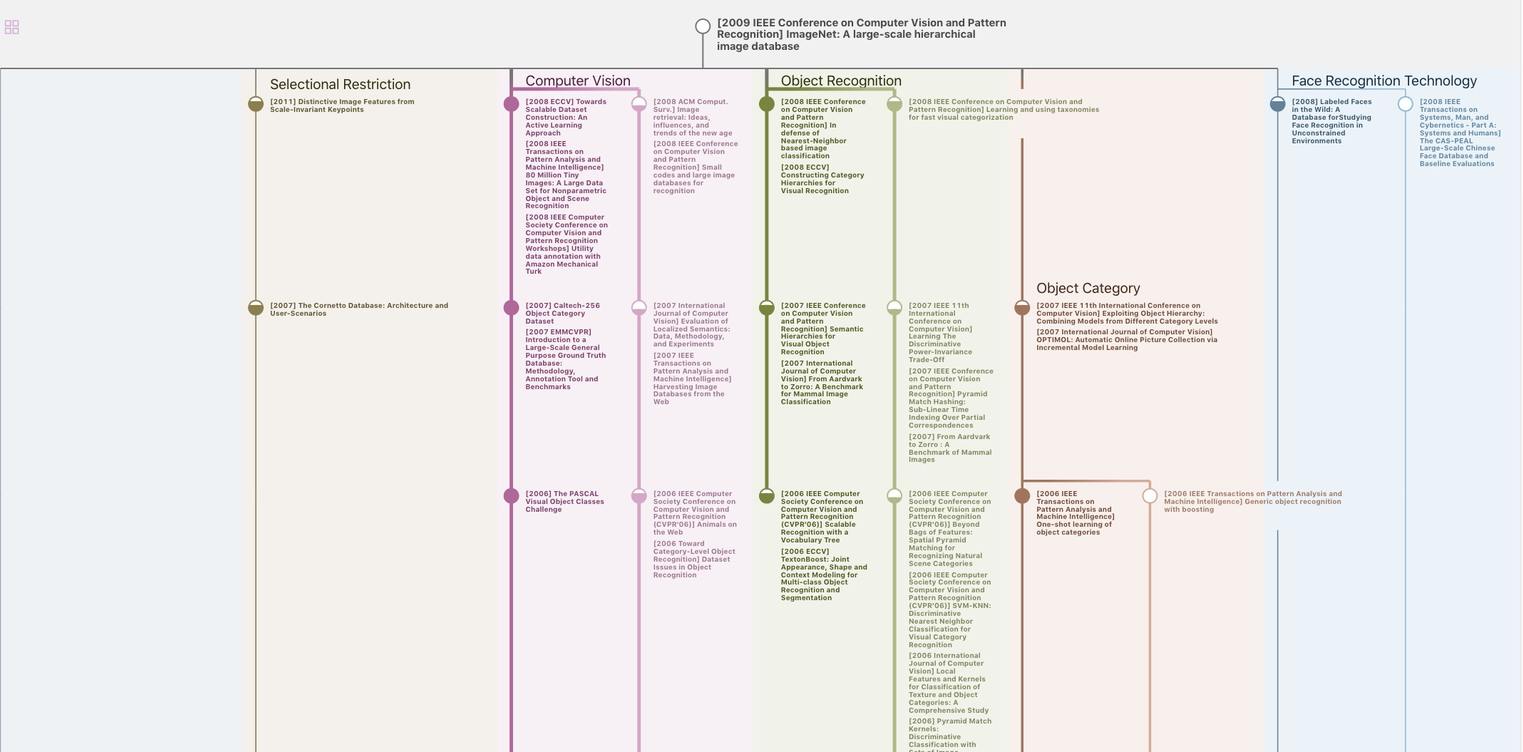
生成溯源树,研究论文发展脉络
Chat Paper
正在生成论文摘要