Max-pooling loss training of long short-term memory networks for small-footprint keyword spotting
2016 IEEE Spoken Language Technology Workshop (SLT)(2016)
摘要
We propose a max-pooling based loss function for training Long Short-Term Memory (LSTM) networks for small-footprint keyword spotting (KWS), with low CPU, memory, and latency requirements. The max-pooling loss training can be further guided by initializing with a cross-entropy loss trained network. A posterior smoothing based evaluation approach is employed to measure keyword spotting performance. Our experimental results show that LSTM models trained using cross-entropy loss or max-pooling loss outperform a cross-entropy loss trained baseline feed-forward Deep Neural Network (DNN). In addition, max-pooling loss trained LSTM with randomly initialized network performs better compared to cross-entropy loss trained LSTM. Finally, the max-pooling loss trained LSTM initialized with a cross-entropy pre-trained network shows the best performance, which yields 67:6% relative reduction compared to baseline feed-forward DNN in Area Under the Curve (AUC) measure.
更多查看译文
关键词
LSTM,keyword spotting,max-pooling loss,small-footprint
AI 理解论文
溯源树
样例
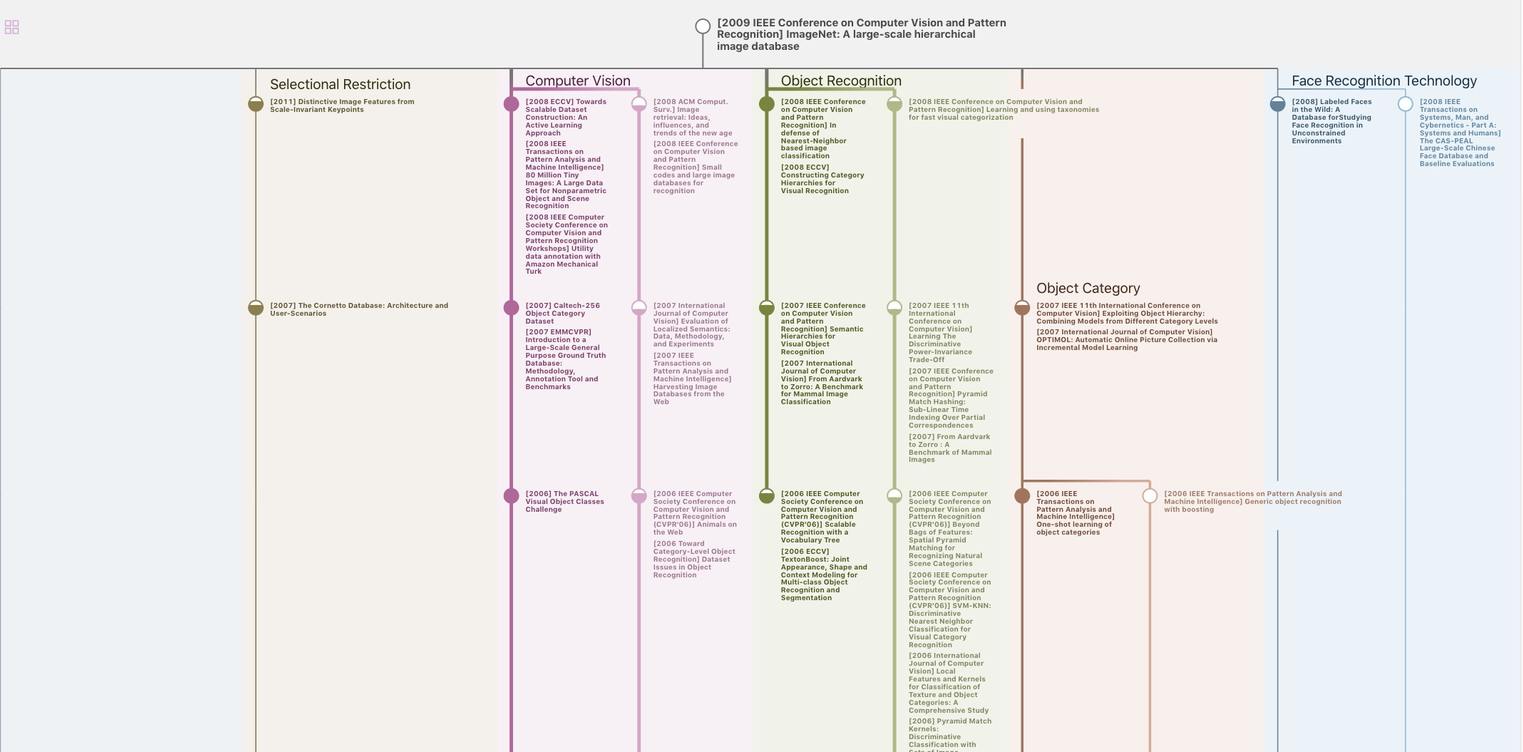
生成溯源树,研究论文发展脉络
Chat Paper
正在生成论文摘要