Robust Multi-View Feature Selection
2016 IEEE 16th International Conference on Data Mining (ICDM)(2016)
摘要
High-throughput technologies have enabled us to rapidly accumulate a wealth of diverse data types. These multi-view data contain much more information to uncover the cluster structure than single-view data, which draws raising attention in data mining and machine learning areas. On one hand, many features are extracted to provide enough information for better representations, on the other hand, such abundant features might result in noisy, redundant and irrelevant information, which harms the performance of the learning algorithms. In this paper, we focus on a new topic, multi-view unsupervised feature selection, which aims to discover the discriminative features in each view for better explanation and representation. Although there are some exploratory studies along this direction, most of them employ the traditional feature selection by putting the features in different views together and fail to evaluate the performance in the multi-view setting. The features selected in this way are difficult to explain due to the meaning of different views, which disobeys the goal of feature selection as well. In light of this, we intend to give a correct understanding of multi-view feature selection. Different from the existing work, which either incorrectly concatenates the features from different views, or takes huge time complexity to learn the pseudo labels, we propose a novel algorithm, Robust Multi-view Feature Selection (RMFS), which applies robust multi-view K-means to obtain the robust and high quality pseudo labels for sparse feature selection in an efficient way. Nontrivially we give the solution by taking the derivatives and further provide a K-means-like optimization to update several variables in a unified framework with the convergence guarantee. We demonstrate extensive experiments on three real-world multi-view data sets, which illustrate the effectiveness and efficiency of RMFS in terms of both single-view and multi-view evaluations by a large margin.
更多查看译文
关键词
Multi-view Learning,Feature Selection,Robust Clustering
AI 理解论文
溯源树
样例
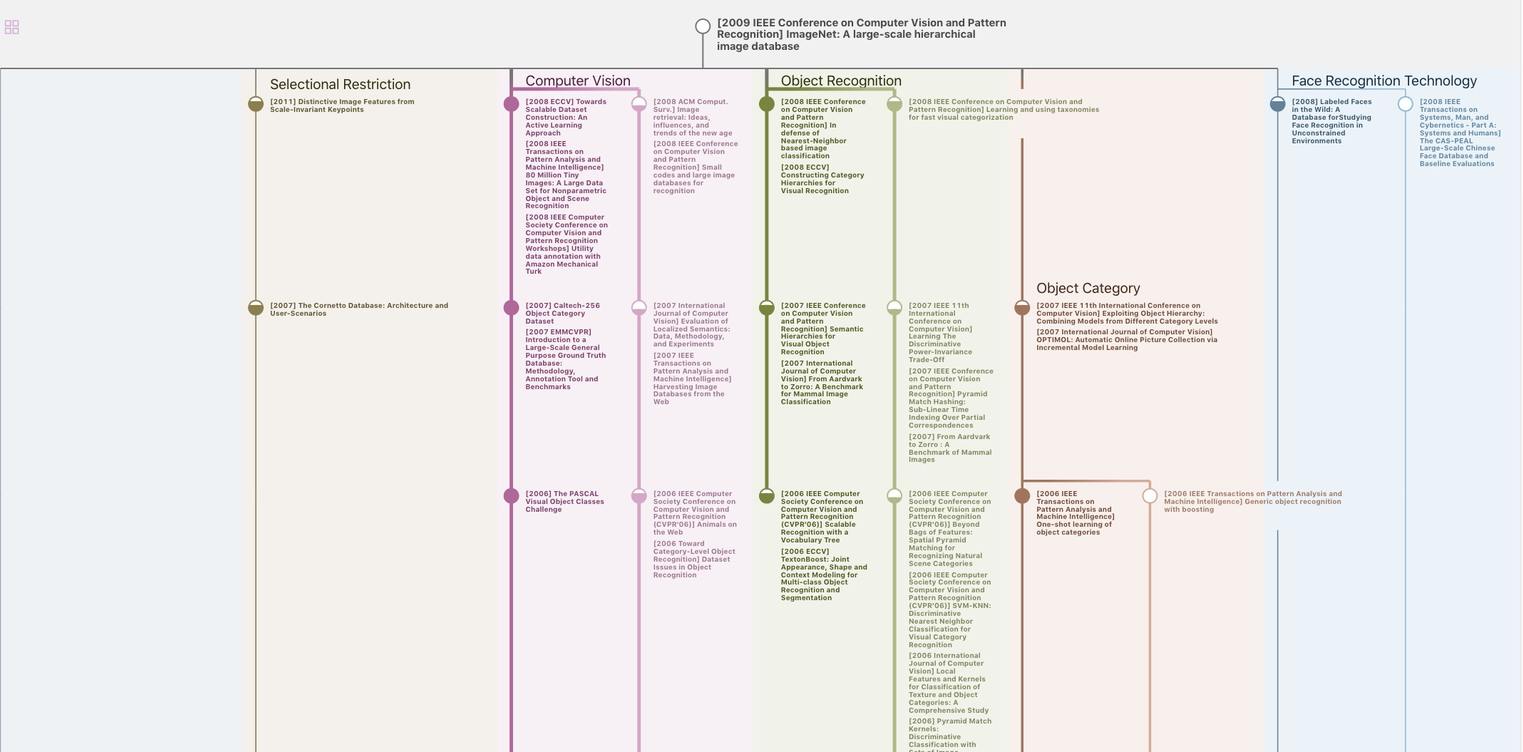
生成溯源树,研究论文发展脉络
Chat Paper
正在生成论文摘要