From Training To Match Performance: A Predictive And Explanatory Study On Novel Tracking Data
2016 IEEE 16TH INTERNATIONAL CONFERENCE ON DATA MINING WORKSHOPS (ICDMW)(2016)
摘要
The recent FIFA approval of the use of Electronic Performance and Tracking Systems (EPTS) during competition, has provided the availability of novel data regarding physical player performance. The analysis of this kind of information will provide teams with competitive advantages, by gaining a deeper understanding of the relation between training and match load, and individual player's fitness characteristics. In order to make sense of this physical data, which is inherently complex, machine learning algorithms that exploit both non-linear and linear relations among variables could be of great aid on building predictive and explanatory models. Also, the increasing availability of information brings the necessity and the challenge for successful interpretation of these models in order to be able to translate the findings into information that can be quickly applied by fast-paced practitioners, such as physical coaches. For season 2015-2016 F.C.Barcelona has collected both physical information from both training sessions and matches using EPTS devices. This study focuses primarily on evaluating up to what extent is possible to predict match performance from training and match physical information. Different machine learning algorithms are applied for building predictive regression models, in combination with feature selection techniques and Principal Component Analysis (PCA) for dimensionality reduction. Physical Variables are segmented into three groups: Locomotor, Metabolic and Mechanical variables, reaching successful prediction rates in 11 out of 17 total variables, based on a threshold determined by expert physical coaches. A normalized root mean square error metric is proposed that allows better understanding of results for practitioners. The second part of this study is focused on understanding the predictor variables that better explain each of the 17 analyzed match variables. It was found that specific variables can act as representatives of the set of highly correlated ones, so reducing greatly the amount of variables needed in the periodical physical analysis carried out by coaches, passing from 17 to 4 variables in average.
更多查看译文
关键词
sports analytics,gps,regression,football analytics,epts,feature selection
AI 理解论文
溯源树
样例
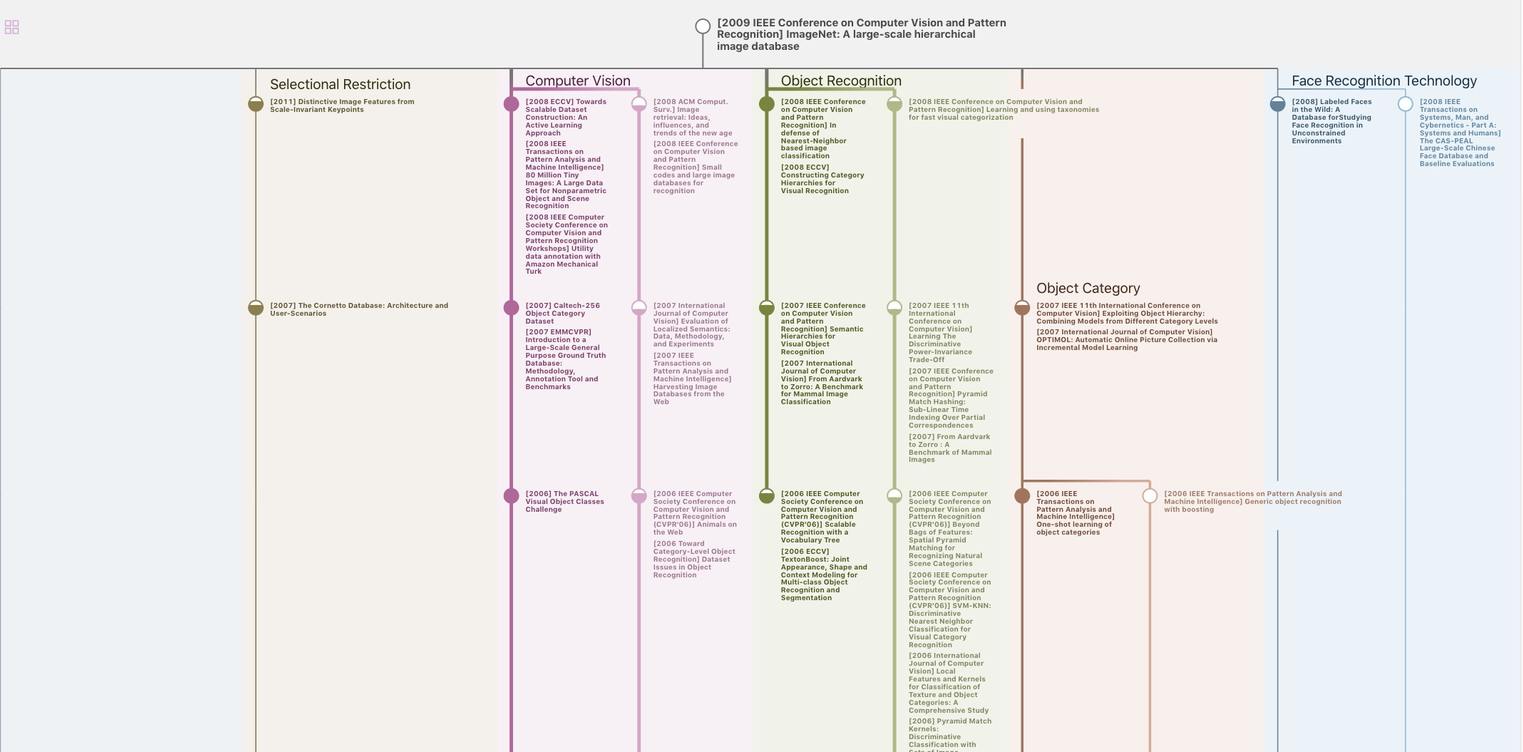
生成溯源树,研究论文发展脉络
Chat Paper
正在生成论文摘要