Functional Regression with Mode-Sparsity Constraint
2016 IEEE 16TH INTERNATIONAL CONFERENCE ON DATA MINING (ICDM)(2016)
摘要
Functional data is ubiquitous in many domains such as healthcare, social media, manufacturing process, sensor networks, etc. Functional data analysis involves the analysis of data which is treated as infinite-dimensional continuous functions rather than discrete, finite-dimensional vectors. In this paper, we propose a novel function-on-function regression model based on mode-sparsity regularization. The main idea is to represent the regression coefficient function between predictor and response as the double expansion of basis functions, and then use mode-sparsity constraint to automatically filter out the irrelevant basis functions for both predictors and responses. The mode-sparsity regularization covers a wide spectrum of sparse models for function-on-function regression. The resulting optimization problem is challenging due to the non-smooth property of the mode-sparsity. We develop an efficient and convergence-guaranteed algorithm to solve the problem. The effectiveness of the proposed approach is verified on benchmark functional data sets in various domains.
更多查看译文
关键词
Functional regression,mode-sparsity
AI 理解论文
溯源树
样例
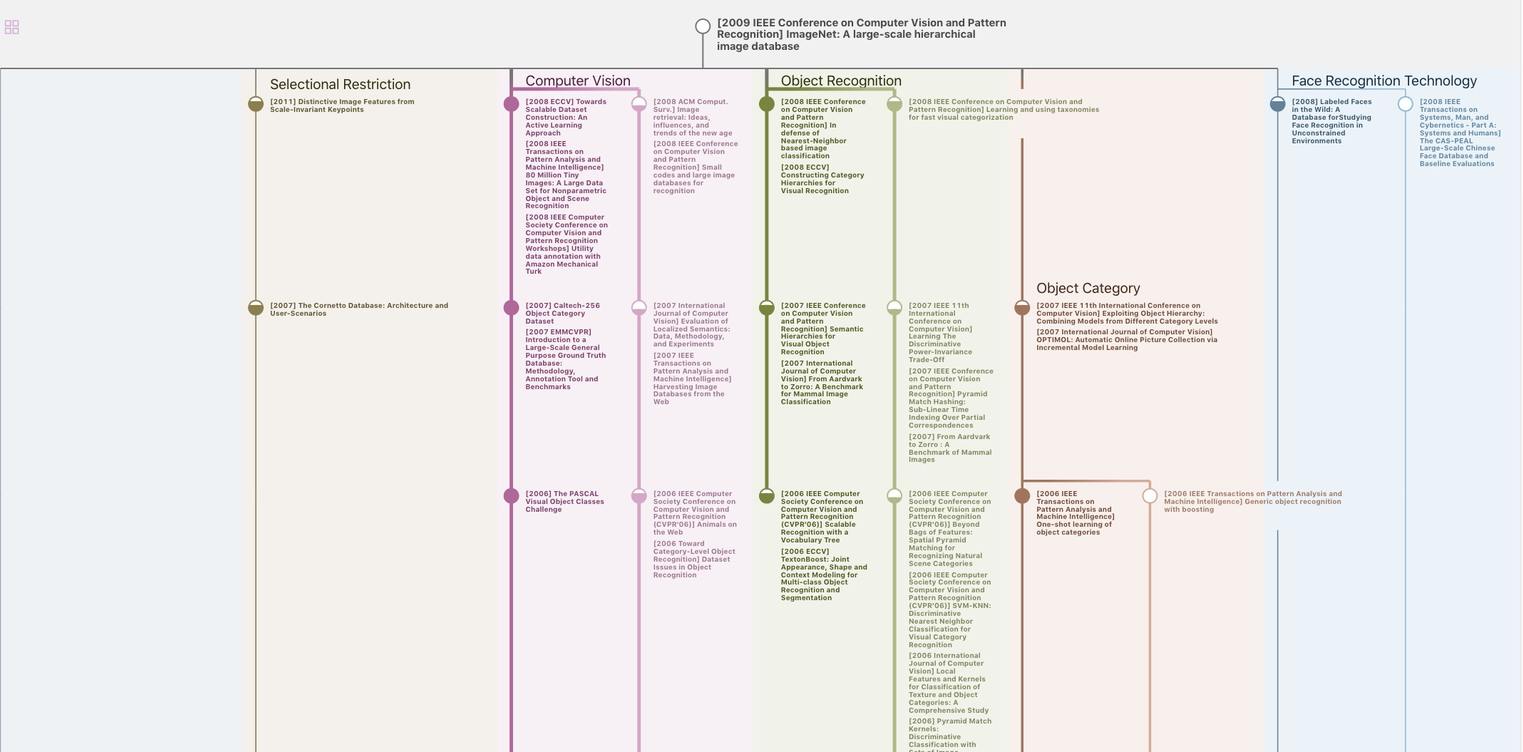
生成溯源树,研究论文发展脉络
Chat Paper
正在生成论文摘要