Learning Compatibility Across Categories For Heterogeneous Item Recommendation
2016 IEEE 16th International Conference on Data Mining (ICDM)(2016)
摘要
Identifying relationships between items is a key task of an online recommender system, in order to help users discover items that are functionally complementary or visually compatible. In domains like clothing recommendation, this task is particularly challenging since a successful system should be capable of handling a large corpus of items, a huge amount of relationships among them, as well as the high-dimensional and semantically complicated features involved. Furthermore, the human notion of "compatibility" to capture goes beyond mere similarity: For two items to be compatible-whether jeans and a t-shirt, or a laptop and a charger-they should be similar in some ways, but systematically different in others.In this paper we propose a novel method, Monomer, to learn complicated and heterogeneous relationships between items in product recommendation settings. Recently, scalable methods have been developed that address this task by learning similarity metrics on top of the content of the products involved. Here our method relaxes the metricity assumption inherent in previous work and models multiple localized notions of 'relatedness,' so as to uncover ways in which related items should be systematically similar, and systematically different. Quantitatively, we show that our system achieves state-of-the-art performance on large-scale compatibility prediction tasks, especially in cases where there is substantial heterogeneity between related items.
更多查看译文
关键词
Recommender Systems,Visual Compatibility,Metric Learning
AI 理解论文
溯源树
样例
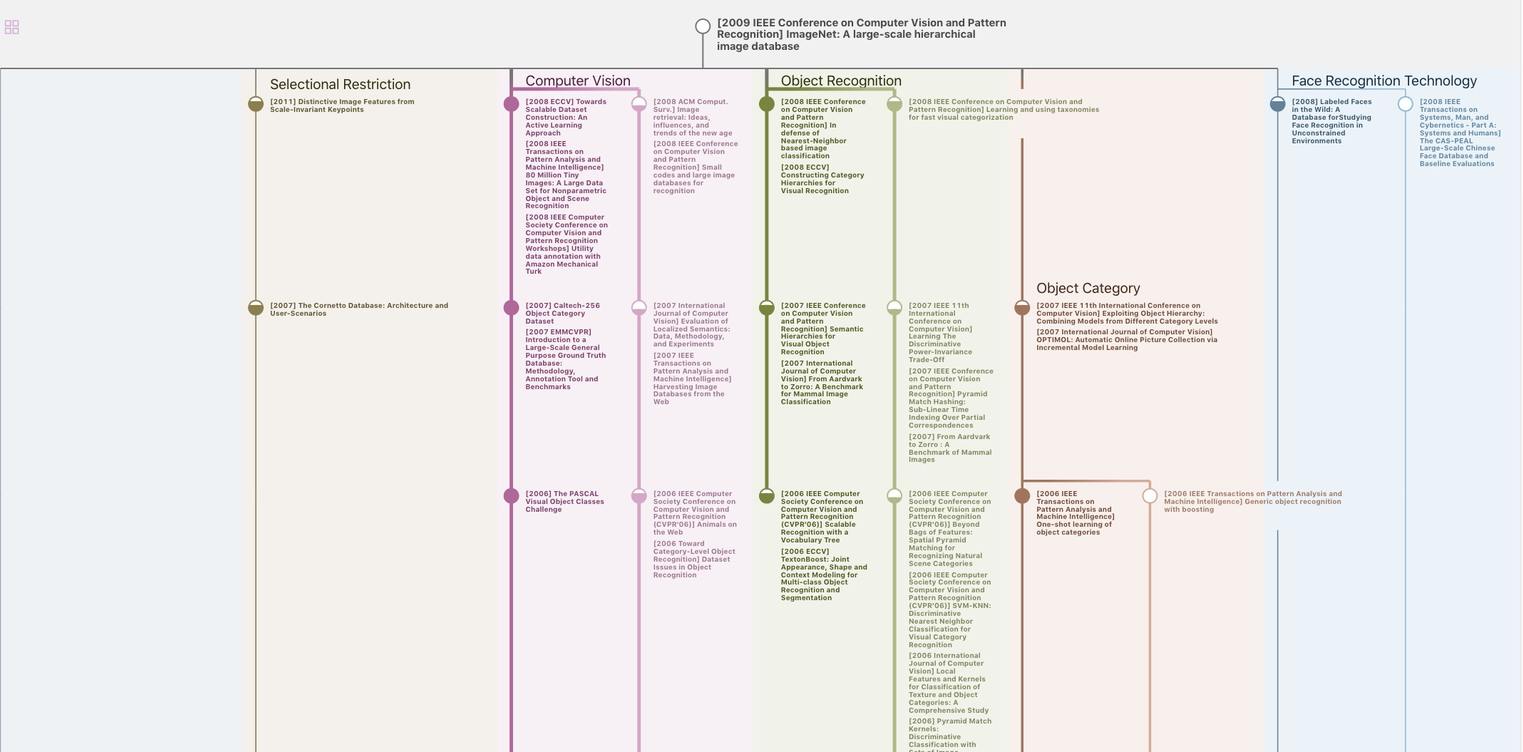
生成溯源树,研究论文发展脉络
Chat Paper
正在生成论文摘要