Adaptive Neighborhood Propagation by Joint L2,1-Norm Regularized Sparse Coding for Representation and Classification
2016 IEEE 16th International Conference on Data Mining (ICDM)(2016)
摘要
We propose a new transductive label propagation method, termed Adaptive Neighborhood Propagation (Adaptive-NP) by joint L2,1-norm regularized sparse coding, for semi-supervised classification. To make the predicted soft labels more accurate for predicting the labels of samples and to avoid the tricky process of choosing the optimal neighborhood size or kernel width for graph construction, Adaptive-NP seamlessly integrates sparse coding and neighborhood propagation into a unified framework. That is, the sparse reconstruction error and classification error are combined for joint minimization, which clearly differs from traditional methods that explicitly separate graph construction and label propagation into independent steps, which may result in inaccurate predictions. Note that our Adaptive-NP alternately optimize the sparse codes and soft labels matrices, where the sparse codes are used as adaptive weights for neighborhood propagation at each iteration, so the tricky process of determining neighborhood size or kernel width is avoided. Besides, for enhancing sparse coding, we use the L2,1-norm constraint on the sparse coding coefficients and the reconstruction error at the same time for delivering more accurate and robust representations. Extensive simulations show that our model can deliver state-of-the-art performances on several public datasets for classification.
更多查看译文
关键词
Transductive learning,linear neighborhood propagation,L2,1-norm regularized sparse coding,classification
AI 理解论文
溯源树
样例
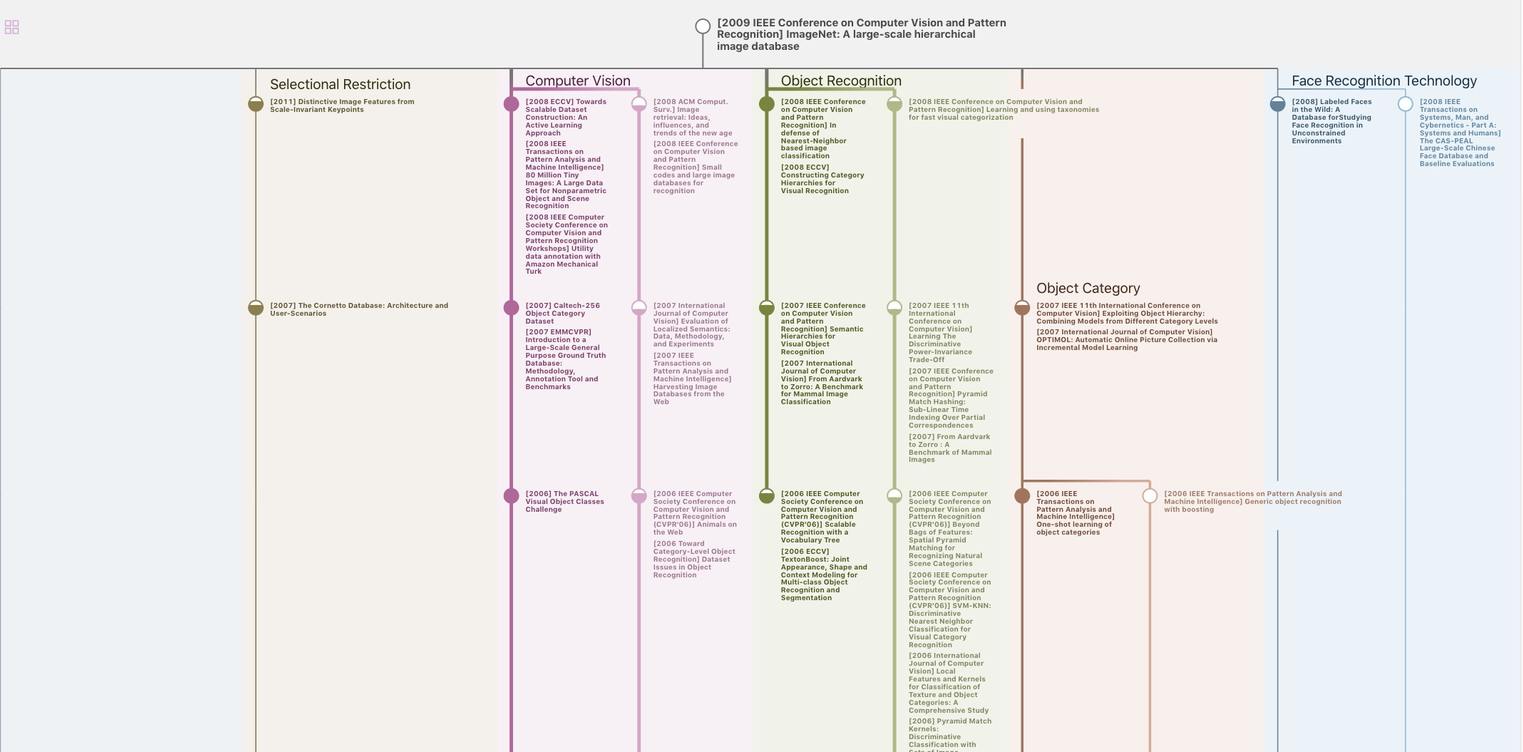
生成溯源树,研究论文发展脉络
Chat Paper
正在生成论文摘要