The Temporal Limits Of Agency For Reaching Movements In Augmented Virtuality
2016 IEEE International Conference on Systems, Man, and Cybernetics (SMC)(2016)
摘要
The sense of agency (SoA) describes the feeling of being the author and in control of one's movements. It is closely linked to automated aspects of sensorimotor control and understood to depend on one's ability to monitor the details of one's movements. As such SoA has been argued to be a critical component of self-awareness in general and contribute to presence in virtual reality environments in particular. A common approach to investigating SoA is to ask participants to perform goal-directed movements and introducing spatial or temporal visuomotor mismatches in the feedback. Feedback movements are traditionally either switched with someone else's movements using a 2D video-feed or modified by providing abstracted feedback about one's actions on a computer screen. The aim of the current study was to quantify conscious monitoring and the SoA for ecologically valid, three dimensional feedback of the participants' actual limb and movements. This was achieved by displaying an Infra-Red (IR) feed of the participants' upper limbs in an augmented virtuality environment (AVE) using a head-mounted display (HMD). Movements could be fed back in real-time (46ms system delay) or with an experimental delay of up to 570ms. As hypothesized, participant's SoA decreased with increasing temporal visuomotor mismatches (p<.001), replicating previous findings and extending them to AVEs. In-line with this literature, we report temporal limits of 222 +/- 60ms (50% psychometric threshold) in N=28 participants. Our results demonstrate the validity of the experimental platform by replicating studies in SoA both qualitatively and quantitatively. We discuss our findings in relation to the use of virtual and mixed reality in research and implications for neurorehabilitation therapies.
更多查看译文
关键词
augmented virtuality,sense of agency,presence,sensory integration,movement feedback,motion capture
AI 理解论文
溯源树
样例
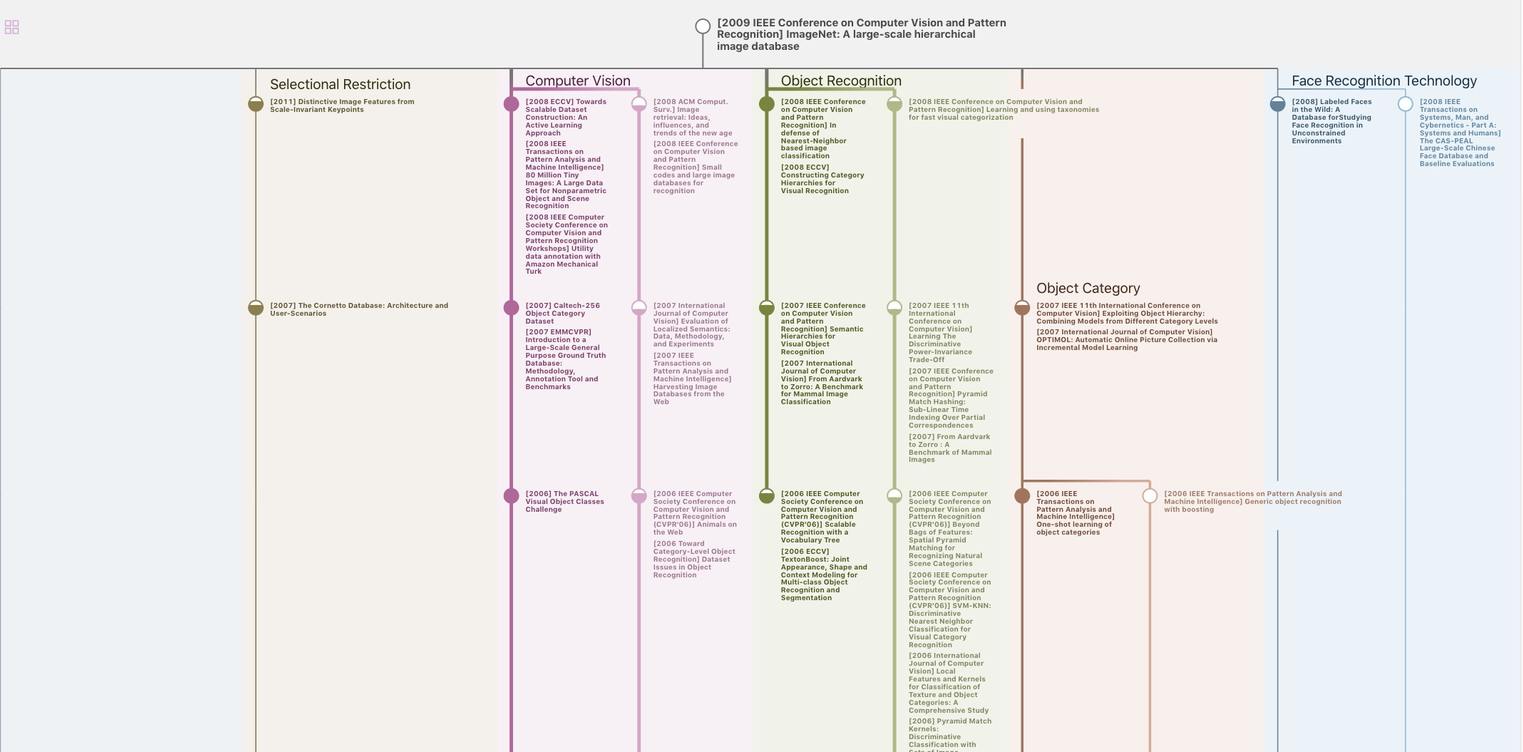
生成溯源树,研究论文发展脉络
Chat Paper
正在生成论文摘要