Affective word ratings for concatenative text-to-speech synthesis.
PCI(2016)
摘要
This work explores affective word ratings as an auxiliary target cost for unit-selection-based concatenative speech synthesis. The method does not require task-specific crafted corpora, nor does it rely on additional annotations, making it ideal for found data. Following the general philosophy of our text-to-speech system, the approach does not enforce any explicit prosodic model, instead the affect information is implicitly modeled via its contribution to the unit-selection cost function. The auxiliary affective feature vector comprises of continuous ratings in three dimensions (valence, arousal and dominance), extracted at the word level via state-of-the-art sentiment analysis techniques. In this case study, speech data consists of several professionally-produced children's audiobooks totaling about 5 hours of speech. The affective dimensions are shown to correlate well with acoustic/prosodic features extracted from the speech data, highlighting their utility for the affective speech synthesis. This is further confirmed via a preference listening test between the baseline and the affective voice.
更多查看译文
关键词
text-to-speech synthesis, affective speech synthesis, sentiment analysis for speech synthesis
AI 理解论文
溯源树
样例
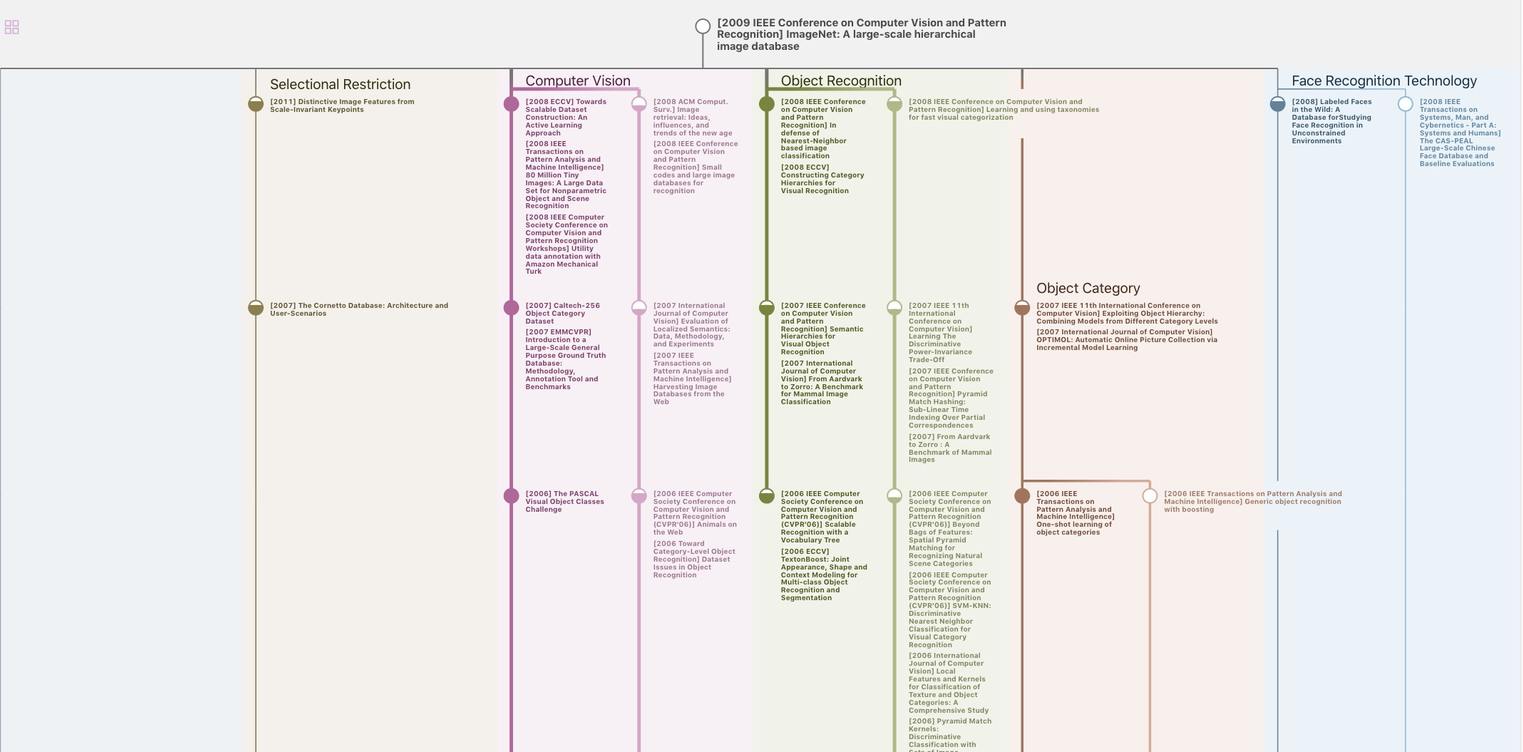
生成溯源树,研究论文发展脉络
Chat Paper
正在生成论文摘要