Microphone Distance Adaptation Using Cluster Adaptive Training for Robust Far Field Speech Recognition
17TH ANNUAL CONFERENCE OF THE INTERNATIONAL SPEECH COMMUNICATION ASSOCIATION (INTERSPEECH 2016), VOLS 1-5: UNDERSTANDING SPEECH PROCESSING IN HUMANS AND MACHINES(2016)
摘要
Microphone distance adaptation is an important and challenging problem for far field speech recognition using a single distant microphone. This paper investigates the use of Cluster Adaptive Training (CAT) to learn a structured Deep Neural Network (DNN) that can be quickly adapted to cope with changes in the distance between the microphone and speaker at test time. A speech corpus was created by re-recording the Wall Street Journal (WSJO) audio using far-field microphones with 8 different distances from the source. Experimental results show that unsupervised adaptation of the CAT-DNN model achieved up to 0.9% absolute word error rate reduction compared to the canonical model trained on multi-style data.
更多查看译文
关键词
deep neural networks, speaker-microphone distance, acoustic modeling, adaptation
AI 理解论文
溯源树
样例
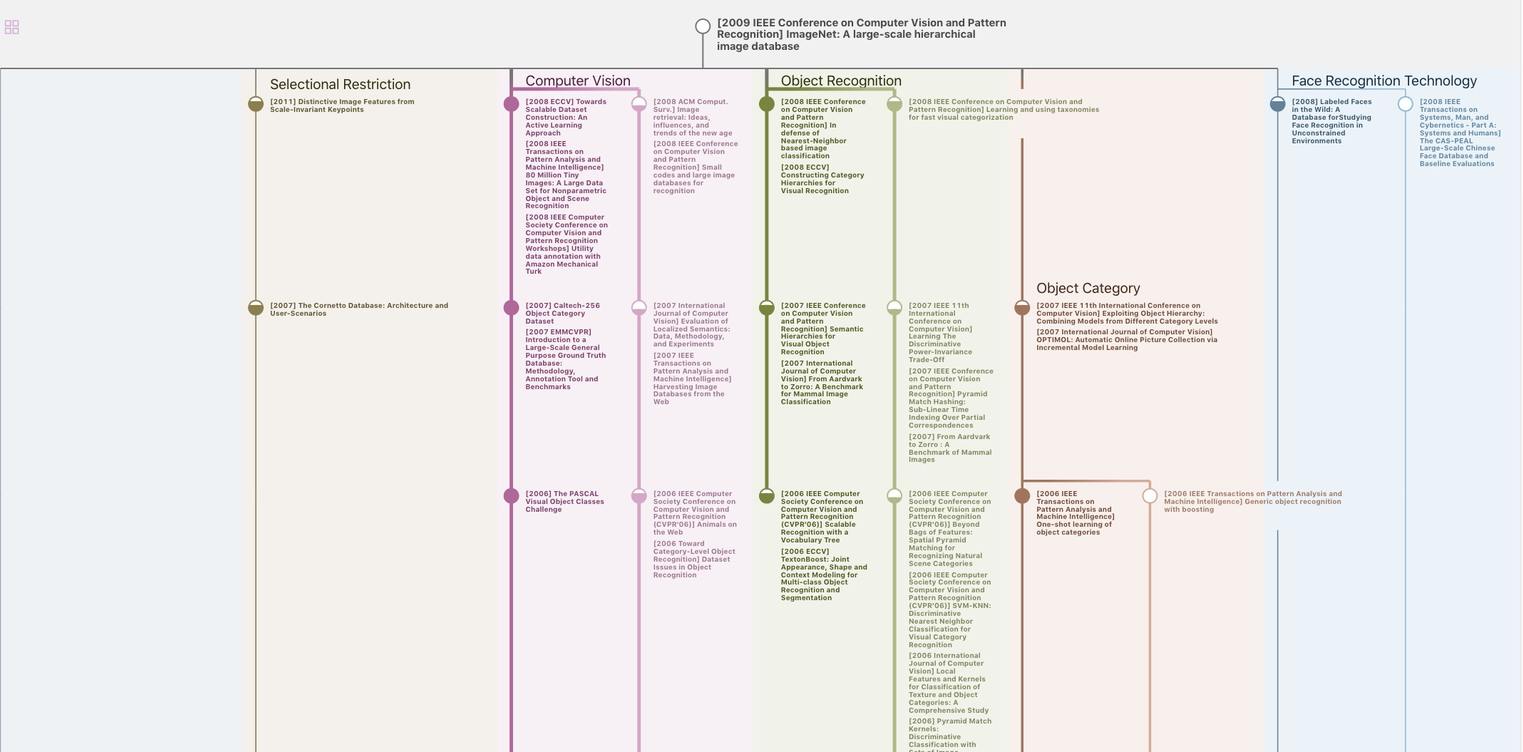
生成溯源树,研究论文发展脉络
Chat Paper
正在生成论文摘要