Noise Aware and Combined Noise Models for Speech Denoising in Unknown Noise Conditions
17TH ANNUAL CONFERENCE OF THE INTERNATIONAL SPEECH COMMUNICATION ASSOCIATION (INTERSPEECH 2016), VOLS 1-5: UNDERSTANDING SPEECH PROCESSING IN HUMANS AND MACHINES(2016)
摘要
Traditional denoising schemes require prior knowledge or statistics of the noise corrupting the signal, or estimate the noise from noise-only portions of the signal, which requires knowledge of speech boundaries. Extending denoising methods to perform well in unknown noise conditions can facilitate processing of data captured in different real life environments, and relax rigid data acquisition protocols. In this paper we propose two methods for denoising speech signals in unknown noise conditions. The first method has two stages. In the first stage we use Long Term Signal Variability features to decide which noise model to use from a pool of available models. Once we determine the noise type, we use Nonnegative Matrix Factorization with a dictionary trained on that noise to denoise the signal. In the second method, we create a combined noise dictionary from different types of noise, and use that dictionary in the denoising phase. Both of our systems improve signal quality, as measured by PESQ scores, for all the noise types we tested, and for different Signal to Noise Ratio levels.
更多查看译文
关键词
Nonnegative Matrix Factorization, Speech Signal Processing, Denoising, Long-Term Signal Variability
AI 理解论文
溯源树
样例
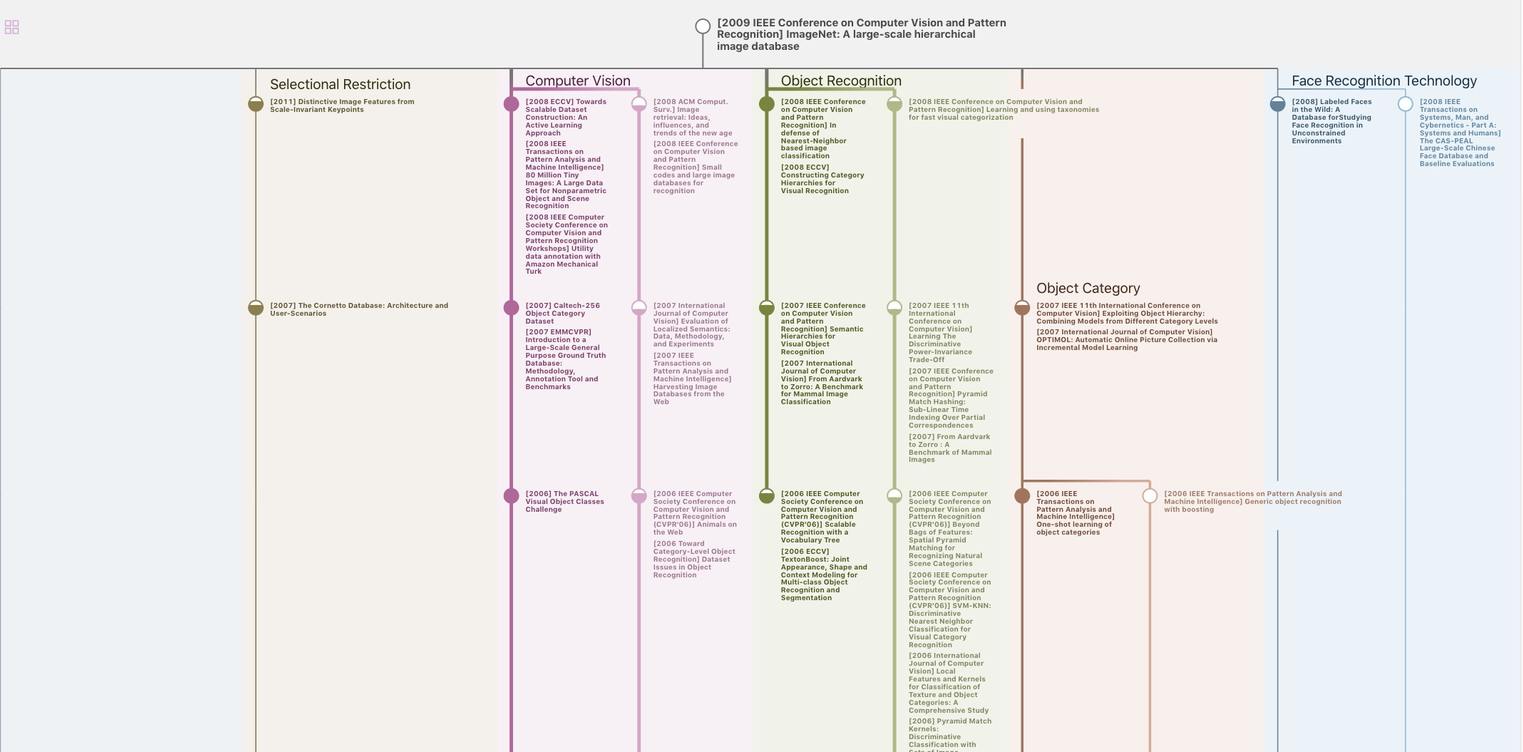
生成溯源树,研究论文发展脉络
Chat Paper
正在生成论文摘要