Non-Iterative Parameter Estimation for Total Variability Model Using Randomized Singular Value Decomposition
17TH ANNUAL CONFERENCE OF THE INTERNATIONAL SPEECH COMMUNICATION ASSOCIATION (INTERSPEECH 2016), VOLS 1-5: UNDERSTANDING SPEECH PROCESSING IN HUMANS AND MACHINES(2016)
摘要
In this paper, we address the problem of parameter estimation for the Total Variability Model (TVM) [1]. Typically, the estimation of the Total Variability Matrix requires several iterations of the Expectation Maximization (EM) algorithm [2], and can be considerably demanding computationally. As a result, fast and efficient parameter estimation remains a key challenge facing the model. We show that it is possible to reduce the Maximum Likelihood parameter estimation problem for TVM into a Singular Value Decomposition (SVD) problem by making some suitably justified approximations in the likelihood function. By using randomized algorithms for efficient computation of the SVD, it becomes possible to accelerate the parameter estimation task remarkably. In addition, we show that this method is able to increase the efficiency of the ivector extraction procedure, and also lends some interpretability to the extracted ivectors.
更多查看译文
关键词
Total Variability Model, ivector
AI 理解论文
溯源树
样例
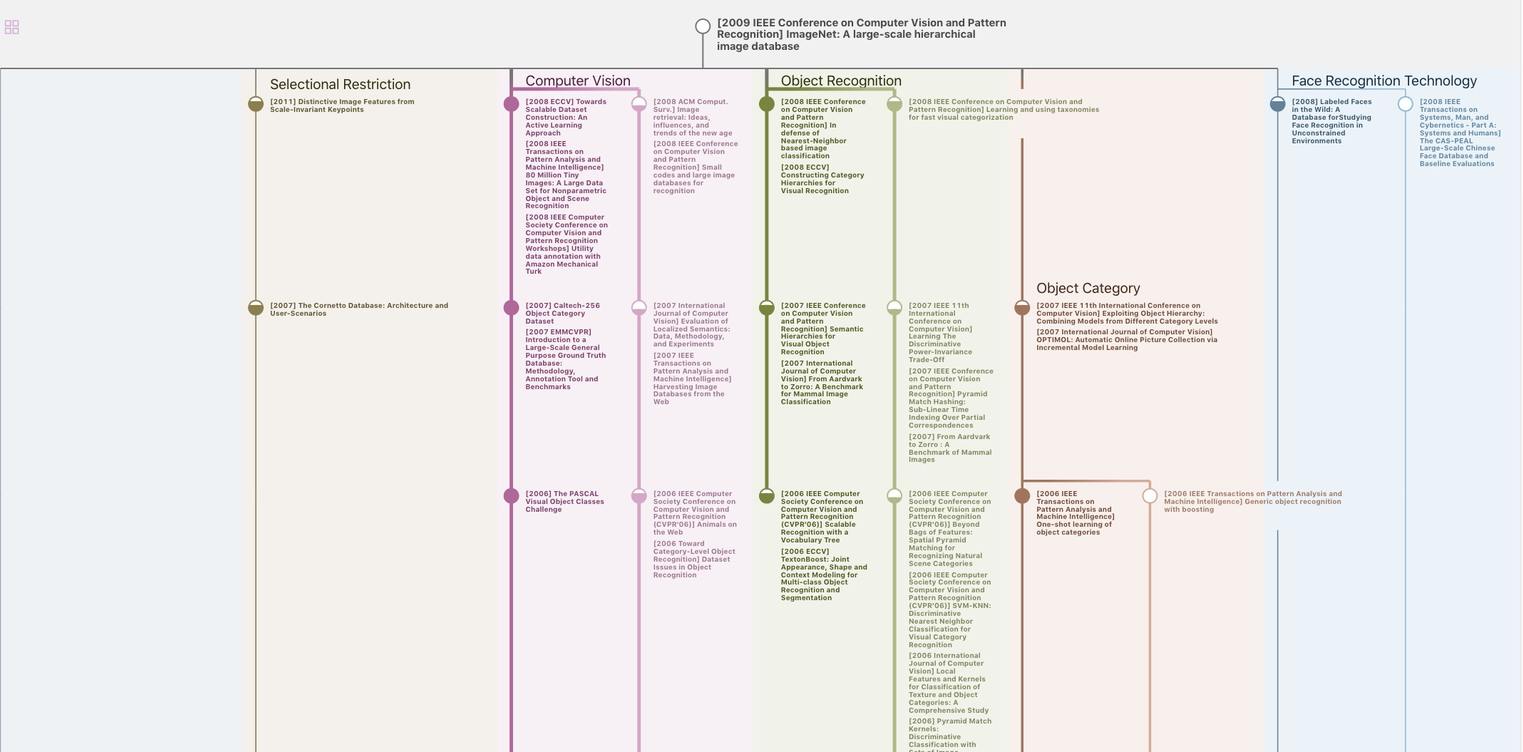
生成溯源树,研究论文发展脉络
Chat Paper
正在生成论文摘要