Entropy Based Pruning for Non-negative Matrix Based Language Models with Contextual Features
17TH ANNUAL CONFERENCE OF THE INTERNATIONAL SPEECH COMMUNICATION ASSOCIATION (INTERSPEECH 2016), VOLS 1-5: UNDERSTANDING SPEECH PROCESSING IN HUMANS AND MACHINES(2016)
摘要
Non-negative matrix based language models have been recently introduced [1] as a computationally efficient alternative to other feature-based models such as maximum -entropy models. We present a new entropy based pruning algorithm for this class of language models, which is fast and scalable. We present perplexity and word error rate results and compare these against regular n-gram pruning. We also train models with location and personalization features and report results at various pruning thresholds. We demonstrate that contextual features are helpful over the vanilla model even after pruning to a similar size.
更多查看译文
关键词
sparse non-negative matrix based language model, entropy based pruning, contextual features, personalization, adaptation, geolocation
AI 理解论文
溯源树
样例
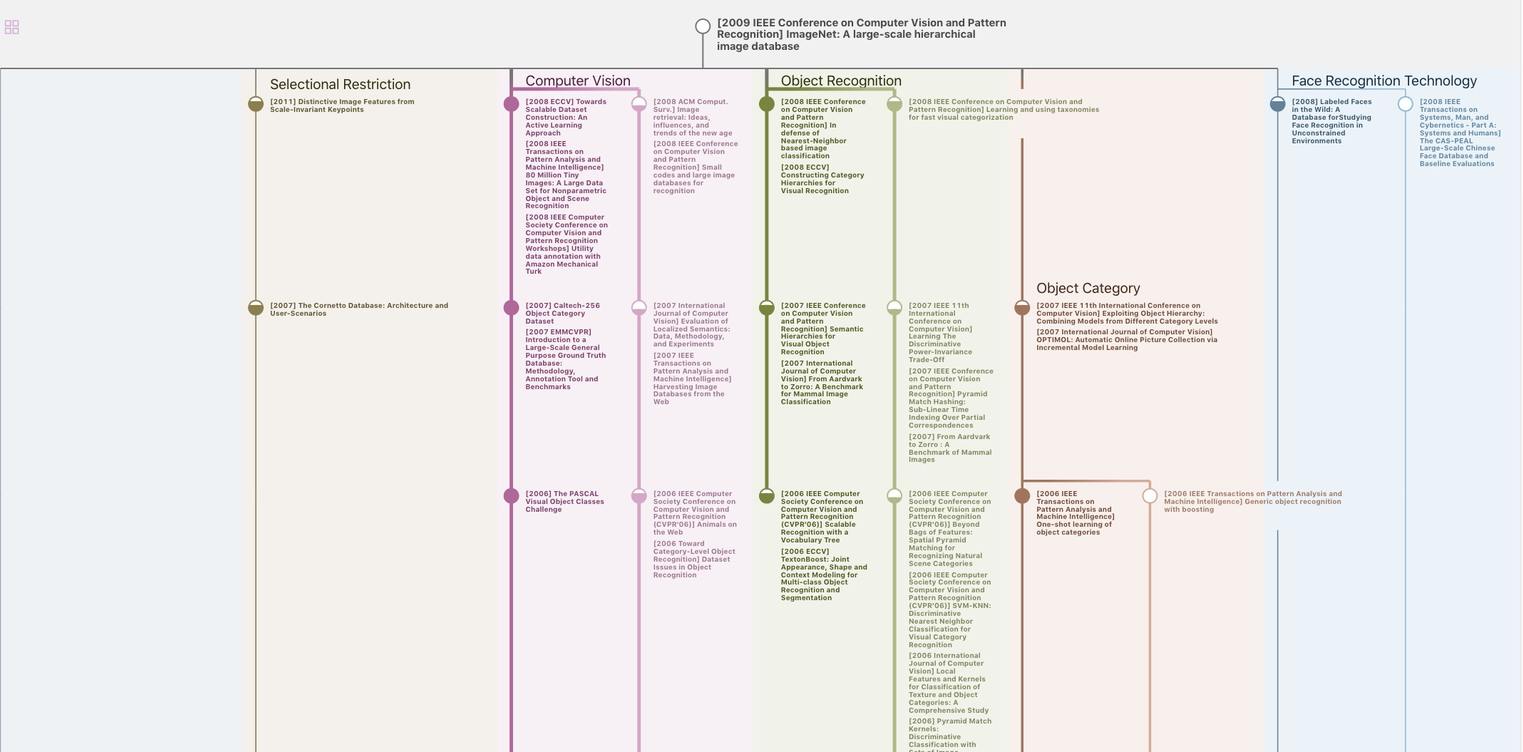
生成溯源树,研究论文发展脉络
Chat Paper
正在生成论文摘要