Recurrent Neural Network-based Phoneme Sequence Estimation using Multiple ASR Systems' Outputs for Spoken Term Detection
17TH ANNUAL CONFERENCE OF THE INTERNATIONAL SPEECH COMMUNICATION ASSOCIATION (INTERSPEECH 2016), VOLS 1-5: UNDERSTANDING SPEECH PROCESSING IN HUMANS AND MACHINES(2016)
摘要
This paper describes a novel correct phoneme sequence estimation method that uses a recurrent neural network (RNN)-based framework for spoken term detection (STD). In an automatic speech recognition (ASR)-based STD framework, ASR performance (word orsub word error rate) affects STD performance. Therefore, it is important to reduce ASR errors to obtain good STD results. In this study, we use an RNN-based phoneme estimator, which estimates a correct phoneme sequence of an utterance from some sorts of phoneme-based transcriptions produced by multiple ASR systems in post-processing, to reduce phoneme errors. With two types of test speech corpora, the proposed phoneme estimator obtained phoneme-based N-best transcriptions with fewer phoneme recognition errors than the N-best transcriptions from the best ASR system we prepared. In addition, the STD system with the RNN-based phoneme estimator drastically improved STD performance with two test collections for STD compared to our previously proposed STD system with a conditional random fields-based phoneme estimator.
更多查看译文
关键词
correct phoneme estimation, post-processing, recurrent neural network, spoken term detection
AI 理解论文
溯源树
样例
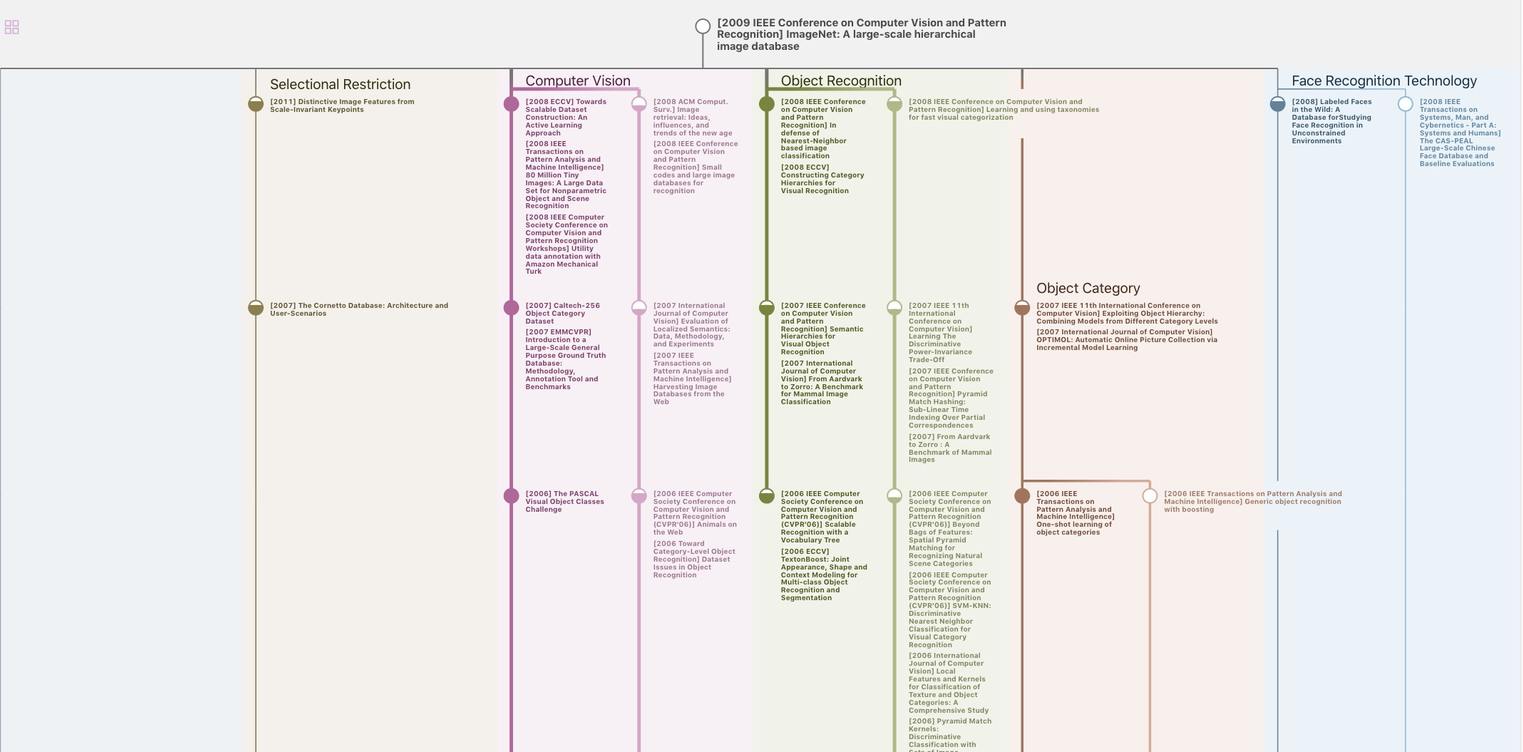
生成溯源树,研究论文发展脉络
Chat Paper
正在生成论文摘要