Improved Neural Network Initialization by Grouping Context-Dependent Targets for Acoustic Modeling
17TH ANNUAL CONFERENCE OF THE INTERNATIONAL SPEECH COMMUNICATION ASSOCIATION (INTERSPEECH 2016), VOLS 1-5: UNDERSTANDING SPEECH PROCESSING IN HUMANS AND MACHINES(2016)
摘要
Neural Network (NN) Acoustic Models (AMs) are usually trained using context-dependent Hidden Markov Model (CDHMM) states as independent targets. For example, the CDHMM states of A-b-2 (second variant of beginning state of A) and A-m-1 (first variant of middle state of A) both correspond to the phone A, and A-b-1 and A-b-2 both correspond to the Context-independent HMM (CI-HMM) state A-b, but this relationship is not explicitly modeled. We propose a method that treats some neurons in the final hidden layer just below the output layer as dedicated neurons for phones or CI-HMM states by initializing connections between the dedicated neurons and the corresponding CD-HMM outputs with stronger weights than to other outputs. We obtained 6.5% and 3.6% relative error reductions with a DNN AM and a CNN AM, respectively, on a 50-hour English broadcast news task and 4.6% reduction with a CNN AM on a 500-hour Japanese task, in all cases after Hessian-free sequence training. Our proposed method only changes the NN parameter initialization and requires no additional computation in NN training or speech recognition runtime.
更多查看译文
关键词
speech recognition, deep neural network, convolutional neural network, context-dependent HMM state target, parameter initialization
AI 理解论文
溯源树
样例
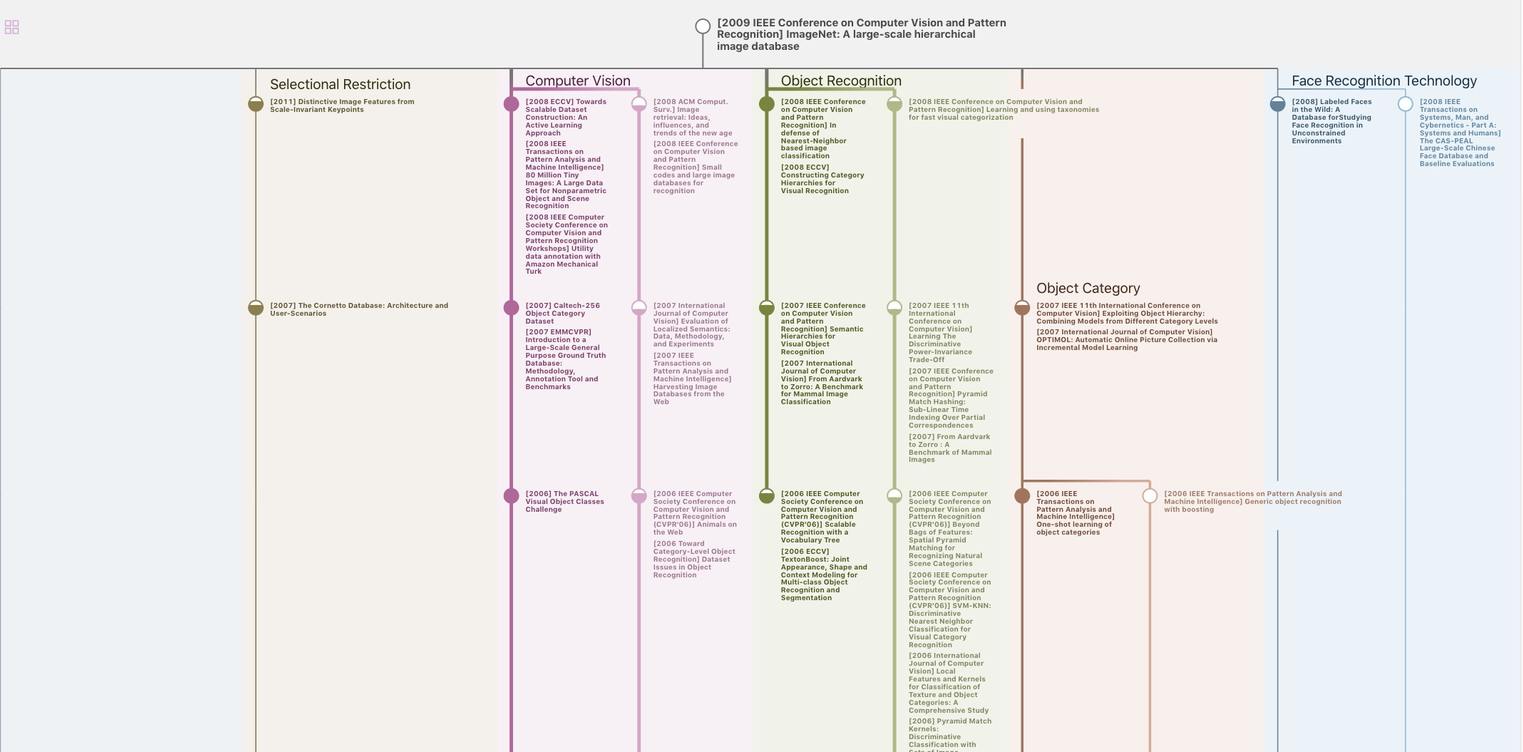
生成溯源树,研究论文发展脉络
Chat Paper
正在生成论文摘要