Multi-GPU-based detection of protein cavities using critical points.
Future Generation Computer Systems(2017)
摘要
Protein cavities are specific regions on the protein surface where ligands (small molecules) may bind. Such cavities are putative binding sites of proteins for ligands. Usually, cavities correspond to voids, pockets, and depressions of molecular surfaces. The location of such cavities is important to better understand protein functions, as needed in, for example, structure-based drug design. This article introduces a geometric method to detecting cavities on the molecular surface based on the theory of critical points. The method, called CriticalFinder, differs from other surface-based methods found in the literature because it directly uses the curvature of the scalar field (or function) that represents the molecular surface, instead of evaluating the curvature of the Connolly function over the molecular surface. To evaluate the accuracy of CriticalFinder, we compare it to other seven geometric methods (i.e., LIGSITECS, GHECOM, ConCavity, POCASA, SURFNET, PASS, and Fpocket). The benchmark results show that CriticalFinder outperforms those methods in terms of accuracy. In addition, the performance analysis of the GPU implementation of CriticalFinder in terms of time consumption and memory space occupancy was carried out.
更多查看译文
关键词
Protein cavity detection,Protein pocket detection,Critical point,Molecular surface,CUDA
AI 理解论文
溯源树
样例
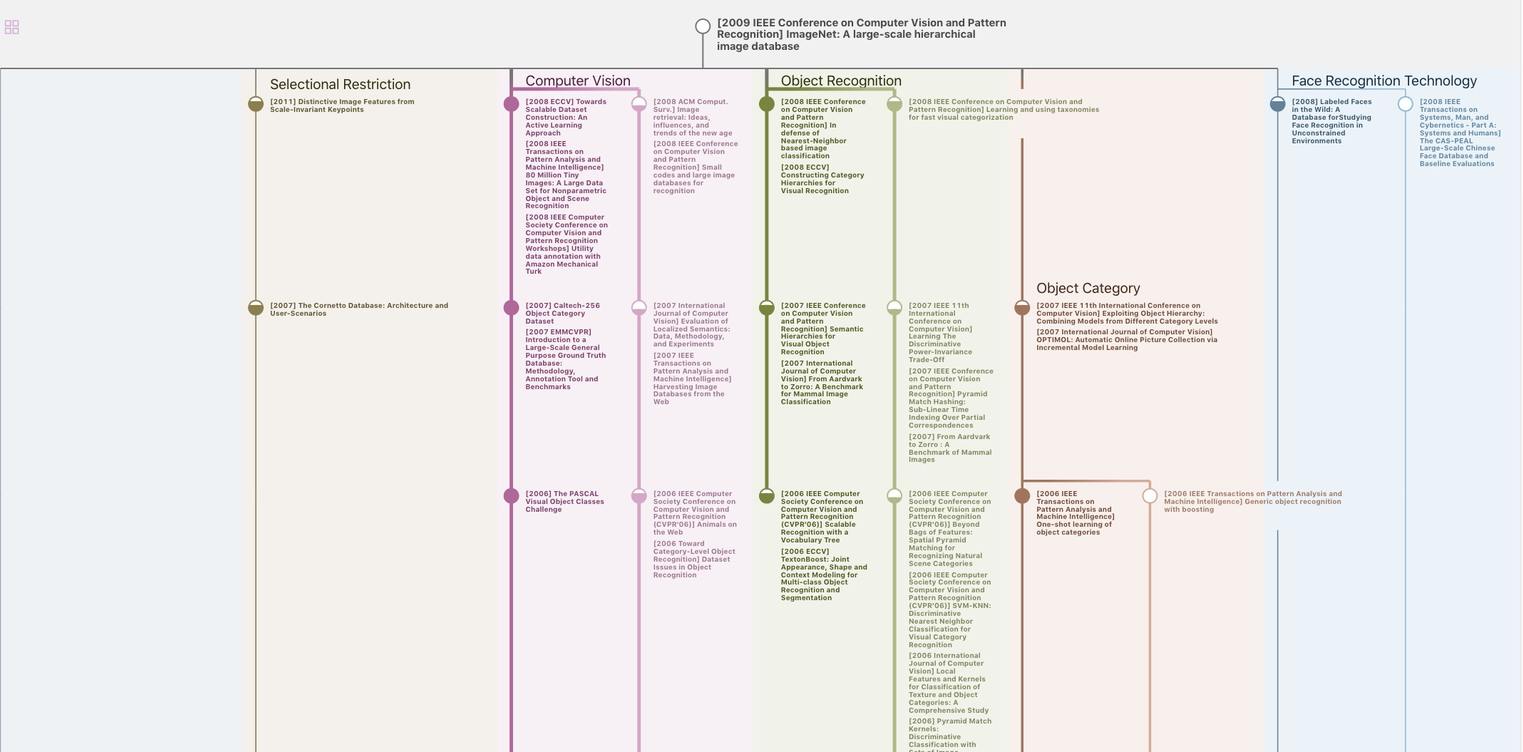
生成溯源树,研究论文发展脉络
Chat Paper
正在生成论文摘要