Tracking by switching state space models.
Computer Vision and Image Understanding(2016)
摘要
Our tracker adaptively changes the state space models (SSMs). Our approach is different in that different types of SSMs (i.e. Image Plane, Ground Plane) are mixed, for the same camera and for the same target representation.Our method efficiently tracks the target on the Ground Plane. Compared to conventional trackers using the Ground Plane, our method only uses a single, possibly moving camera.Our method employs the advanced Particle Filter for visual tracking, which does not fix one SSM and instead switches between multiple possibilities. That eases the handling of complex motion patterns even more, as one can use the SSM where they appear simplest (principle of parsimony). We propose a novel tracking method that allows to switch between different state representations as, e.g., image coordinates in different views or image and ground plane coordinates. During the tracking process, our method adaptively switches between these representations. We demonstrate the applicability of our method for dynamic cameras tracking dynamic objects: Using the image based representation (non-smooth trajectories if the camera is shaking) together with the ground plane based one (estimation uncertainty in visual odometry or ground plane orientation), the disadvantages of both representation forms can be overcome: Non-occluded observations on the image plane provide strong appearance cues for the target. Smooth paths on the ground plane provide strong motion cues with the camera motion factored out. Following a Bayesian tracking approach, we propose a probabilistic framework that determines the most appropriate state space model (SSM)image or ground plane or bothat each time instance. Experimental results demonstrate that our method outperforms the state-of-the-art.
更多查看译文
关键词
Visual tracking,Probabilistic model,State space switch,Ground plane
AI 理解论文
溯源树
样例
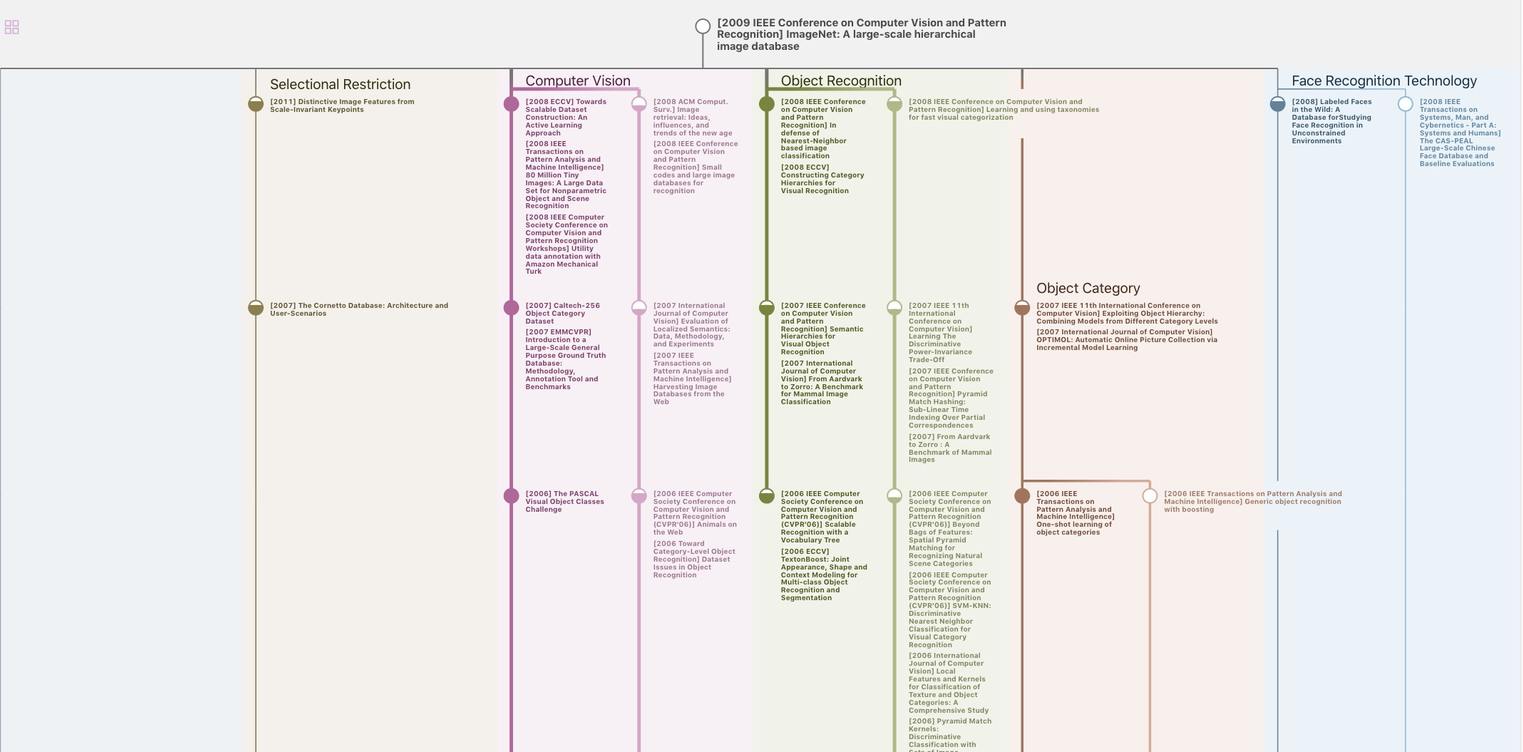
生成溯源树,研究论文发展脉络
Chat Paper
正在生成论文摘要