Expanding the Limits of Vision-based Localization for Long-term Route-following Autonomy.
J. Field Robotics(2017)
摘要
Vision-based, autonomous, route-following algorithms enable robots to autonomously repeat manually driven routes over long distances. Through the use of inexpensive, commercial vision sensors, these algorithms have the potential to enable robotic applications across multiple industries. However, in order to extend these algorithms to long-term autonomy, they must be able to operate over long periods of time. This poses a difficult challenge for vision-based systems in unstructured and outdoor environments, where appearance is highly variable. While many techniques have been developed to perform localization across extreme appearance change, most are not suitable or untested for vision-in-the-loop systems such as autonomous route following, which requires continuous metric localization to keep the robot driving. In this paper, we present a vision-based, autonomous, route-following algorithm that combines multiple channels of information during localization to increase robustness against daily appearance change such as lighting. We explore this multichannel visual teach and repeat framework by adding the following channels of information to the basic single-camera, gray-scale, localization pipeline: images that are resistant to lighting change and images from additional stereo cameras to increase the algorithm's field of view. Using these methods, we demonstrate robustness against appearance change through extensive field deployments spanning over 26 km with an autonomy rate greater than 99.9%. We furthermore discuss the limits of this system when subjected to harsh environmental conditions by investigating keypoint match degradation through time.
更多查看译文
AI 理解论文
溯源树
样例
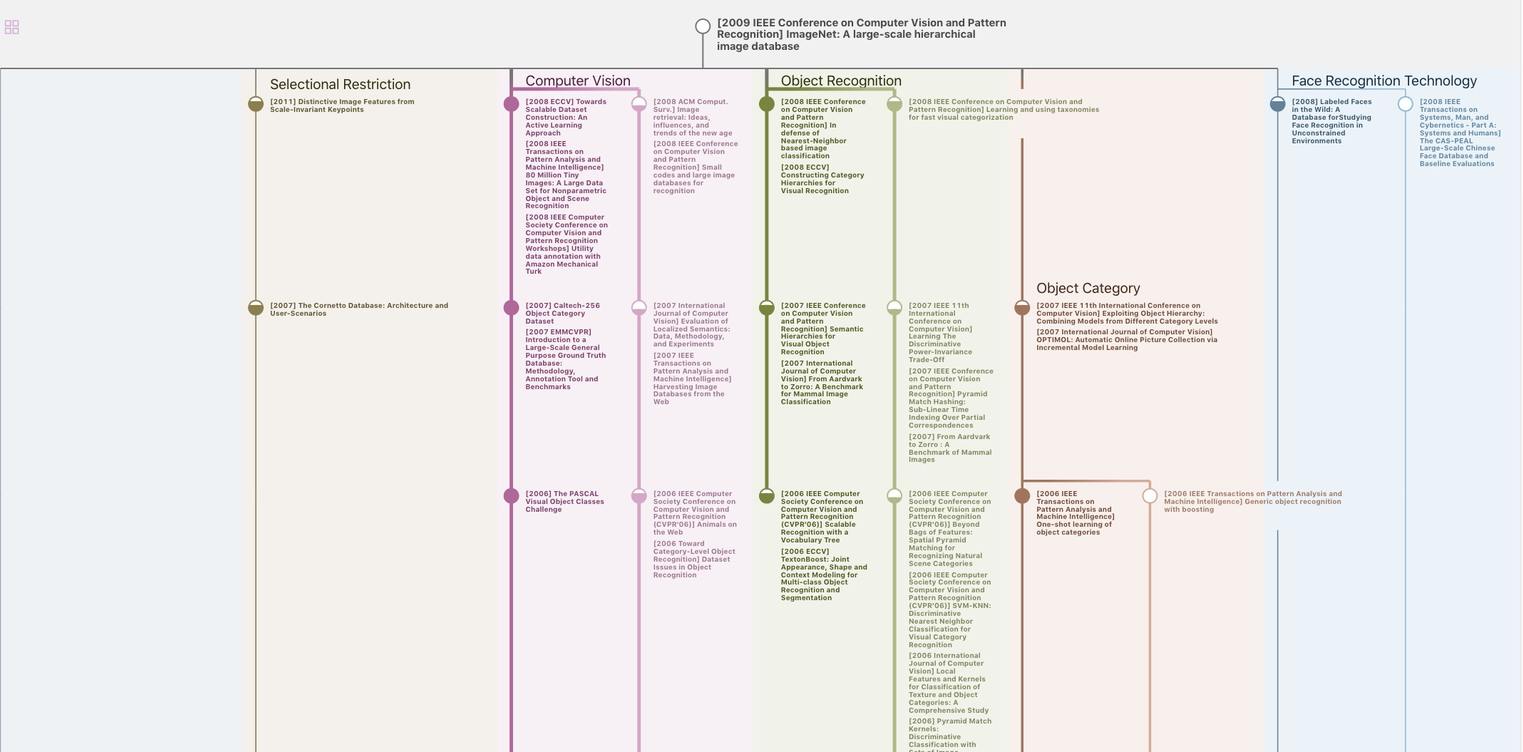
生成溯源树,研究论文发展脉络
Chat Paper
正在生成论文摘要