The Influence of Annotation, Corpus Design, and Evaluation on the Outcome of Automatic Classification of Human Emotions.
Front. ICT(2016)
摘要
The integration of emotions into human computer interaction applications promises a more natural dialog between the user and the technical system he operates. In order to construct such machinery, continuous measuring of the affective state of the user becomes essential. While basic research that is aimed to capture and classify affective signals has progressed, many issues are still prevailing that hinder easy integration of affective signals into human-computer interaction. In this paper, we identify and investigate pitfalls in three steps of the work-flow of affective classification studies. It starts with the process of collecting affective data for the purpose of training suitable classifiers. Emotional data has to be created in which the target emotions are present. Therefore, human participants have to be stimulated suitably. We discuss the nature of these stimuli, their relevance to human-computer interaction and the repeatability of the data recording setting. Second, aspects of annotation procedures are investigated, which include the variances of individual raters, annotation delay, the impact of the used annotation tool and how individual ratings are combined to a unified label. Finally, the evaluation protocol is examined which includes, amongst others, the impact of the performance measure on the accuracy of a classification model. We hereby focus especially on the evaluation of classifier outputs against continuously annotated dimensions. Alongside the discussed problems and pitfalls and the ways how they affect the outcome, we provide solutions and alternatives to overcome these issues. As a final part of the paper we sketch a recording scenario and a set of supporting technologies that can contribute to solve many of the issues mentioned above.
更多查看译文
AI 理解论文
溯源树
样例
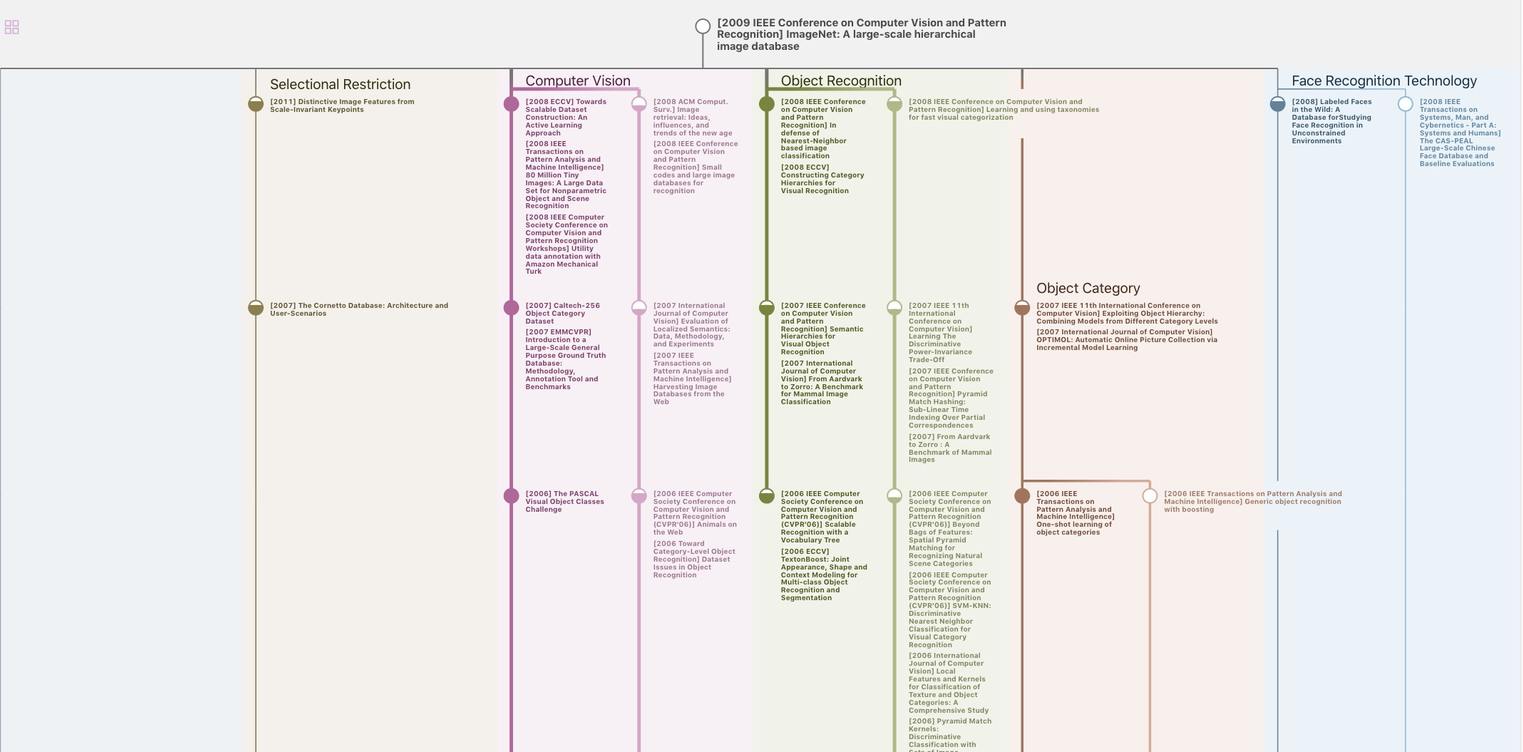
生成溯源树,研究论文发展脉络
Chat Paper
正在生成论文摘要