Enhancing the performance of MOEAs: an experimental presentation of a new fitness guided mutation operator.
JOURNAL OF EXPERIMENTAL & THEORETICAL ARTIFICIAL INTELLIGENCE(2017)
摘要
Multi-objective evolutionary algorithms (MOEAs) are currently a dynamic field of research that has attracted considerable attention. Mutation operators have been utilized by MOEAs as variation mechanisms. In particular, polynomial mutation (PLM) is one of the most popular variation mechanisms and has been utilized by many well-known MOEAs. In this paper, we revisit the PLM operator and we propose a fitness-guided version of the PLM. Experimental results obtained by non-dominated sorting genetic algorithm II and strength Pareto evolutionary algorithm 2 show that the proposed fitness-guided mutation operator outperforms the classical PLM operator, based on different performance metrics that evaluate both the proximity of the solutions to the Pareto front and their dispersion on it.
更多查看译文
关键词
Multi-objective optimisation,evolutionary algorithms,mutation
AI 理解论文
溯源树
样例
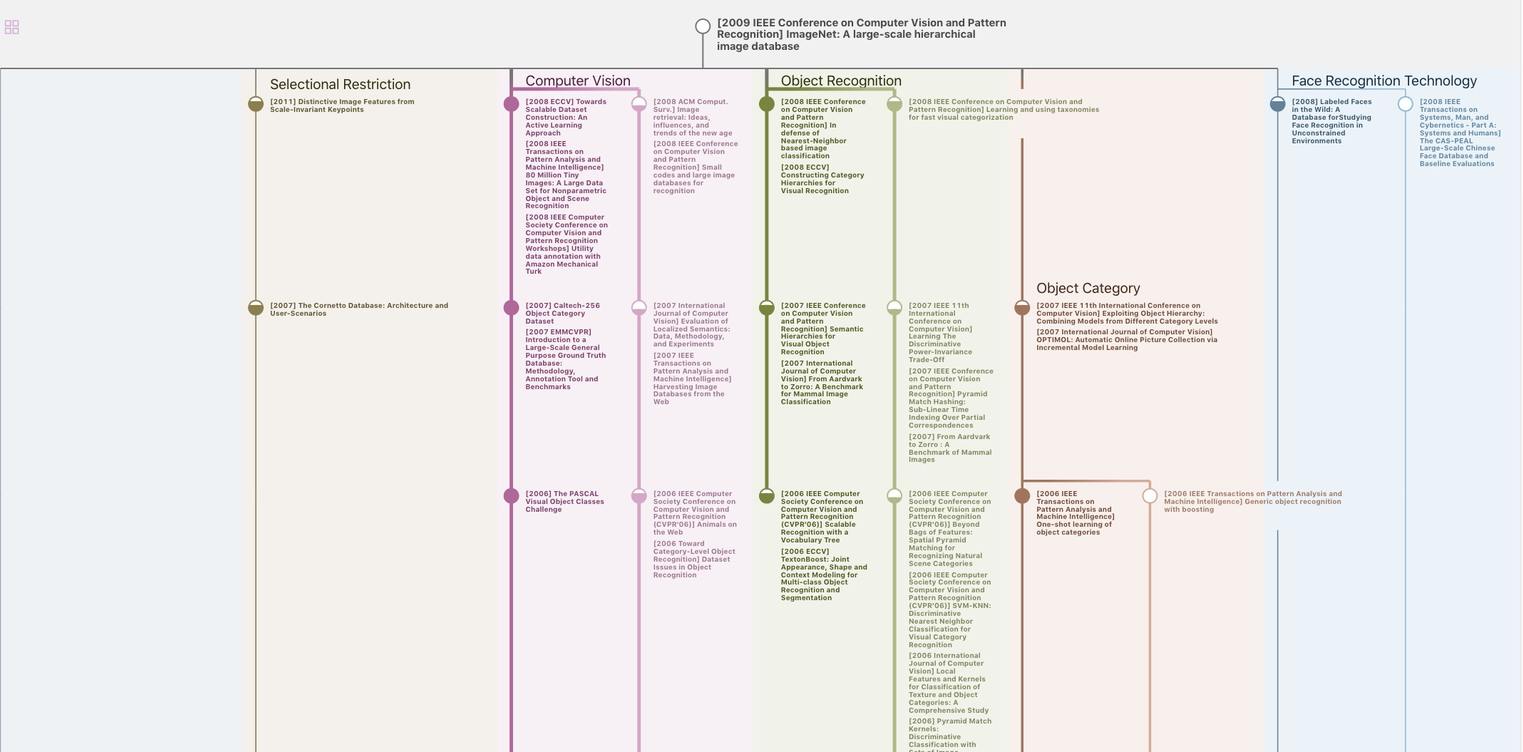
生成溯源树,研究论文发展脉络
Chat Paper
正在生成论文摘要