Robotic Grasp Detection using Deep Convolutional Neural Networks
2017 IEEE/RSJ International Conference on Intelligent Robots and Systems (IROS)(2017)
摘要
Deep learning has significantly advanced computer vision and natural language processing. While there have been some successes in robotics using deep learning, it has not been widely adopted. In this paper, we present a novel robotic grasp detection system that predicts the best grasping pose of a parallel-plate robotic gripper for novel objects using the RGB-D image of the scene. The proposed model uses a deep convolutional neural network to extract features from the scene and then uses a shallow convolutional neural network to predict the grasp configuration for the object of interest. Our multi-modal model achieved an accuracy of 89.21% on the standard Cornell Grasp Dataset and runs at real-time speeds. This redefines the state-of-the-art for robotic grasp detection.
更多查看译文
关键词
deep learning,parallel-plate robotic gripper,deep convolutional neural network,shallow convolutional neural network,standard Cornell Grasp Dataset,computer vision,natural language processing,robotic grasp detection system,RGB-D image
AI 理解论文
溯源树
样例
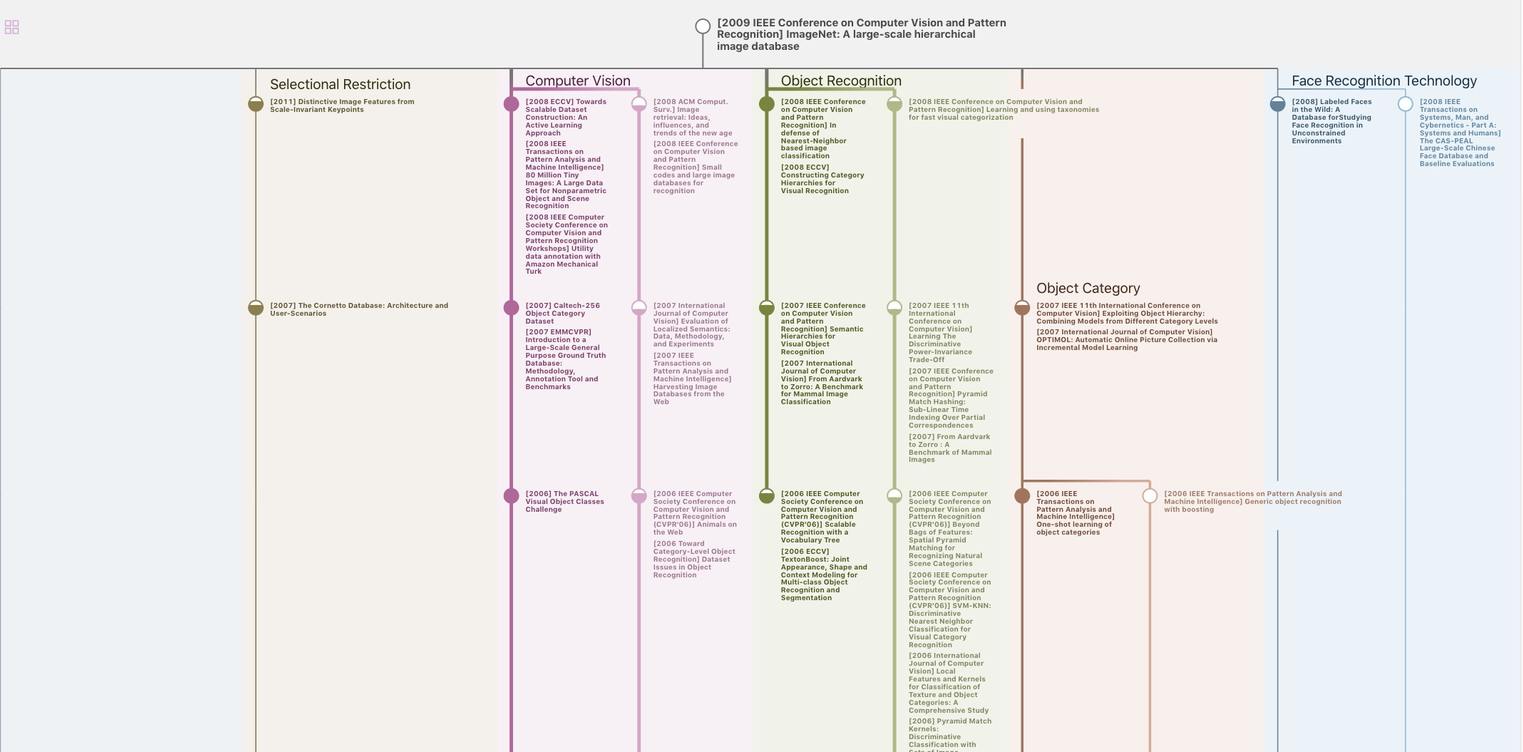
生成溯源树,研究论文发展脉络
Chat Paper
正在生成论文摘要