Deep Feature Flow for Video Recognition
2017 IEEE Conference on Computer Vision and Pattern Recognition (CVPR)(2017)
摘要
Deep convolutional neutral networks have achieved great success on image recognition tasks. Yet, it is non-trivial to transfer the state-of-the-art image recognition networks to videos as per-frame evaluation is too slow and unaffordable. We present deep feature flow, a fast and accurate framework for video recognition. It runs the expensive convolutional sub-network only on sparse key frames and propagates their deep feature maps to other frames via a flow field. It achieves significant speedup as flow computation is relatively fast. The end-to-end training of the whole architecture significantly boosts the recognition accuracy. Deep feature flow is flexible and general. It is validated on two recent large scale video datasets. It makes a large step towards practical video recognition.
更多查看译文
关键词
deep feature flow,deep convolutional neutral networks,expensive convolutional sub-network,sparse key frames,deep feature maps,flow field,flow computation,recognition accuracy,video recognition,image recognition networks,large scale video datasets,end-to-end training
AI 理解论文
溯源树
样例
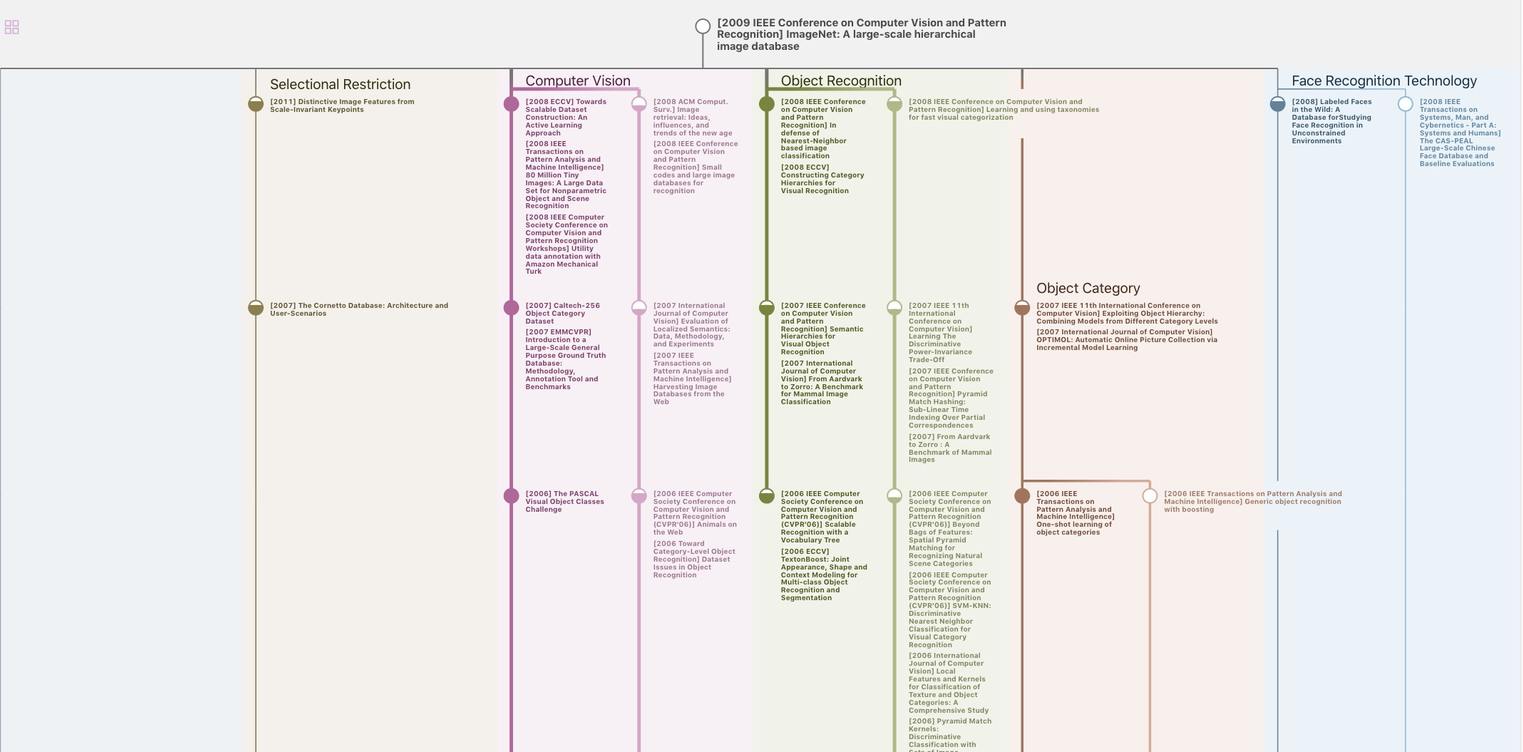
生成溯源树,研究论文发展脉络
Chat Paper
正在生成论文摘要